Targeted Supervised Contrastive Learning for Long-Tailed Recognition
IEEE Conference on Computer Vision and Pattern Recognition(2022)
摘要
Real-world data often exhibits long tail distributions with heavy class imbalance, where the majority classes can dominate the training process and alter the decision bound-aries of the minority classes. Recently, researchers have in-vestigated the potential of supervised contrastive learning for long-tailed recognition, and demonstrated that it provides a strong performance gain. In this paper, we show that while supervised contrastive learning can help improve performance, past baselines suffer from poor uniformity brought in by imbalanced data distribution. This poor uni-formity manifests in samples from the minority class having poor separability in the feature space. To address this problem, we propose targeted supervised contrastive learning (TSC), which improves the uniformity of the feature distribution on the hypersphere. TSC first generates a set of targets uniformly distributed on a hypersphere. It then makes the features of different classes converge to these distinct and uniformly distributed targets during training. This forces all classes, including minority classes, to main-tain a uniform distribution in the feature space, improves class boundaries, and provides better generalization even in the presence of long-tail data. Experiments on multi-ple datasets show that TSC achieves state-of-the-art performance on long-tailed recognition tasks.
更多查看译文
关键词
Transfer/low-shot/long-tail learning
AI 理解论文
溯源树
样例
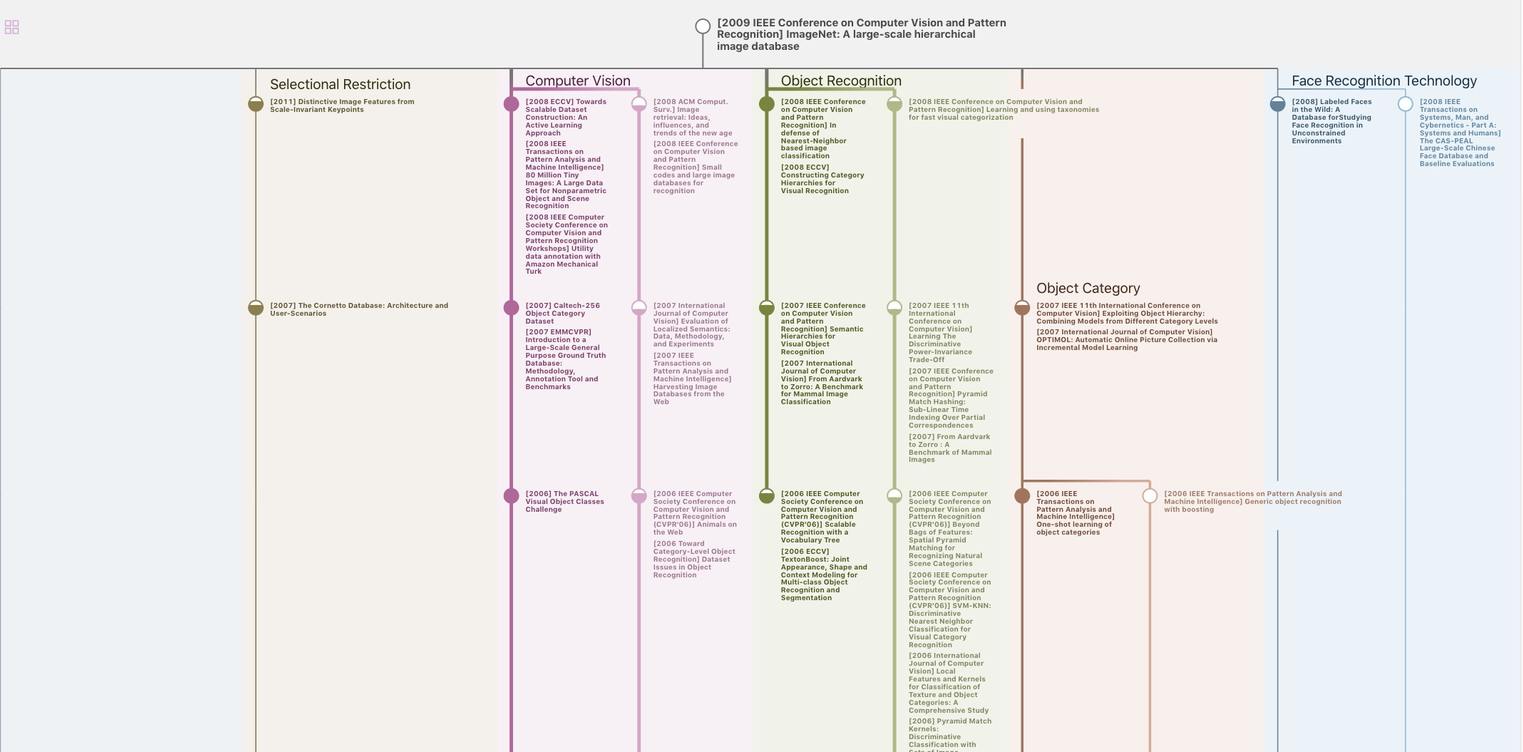
生成溯源树,研究论文发展脉络
Chat Paper
正在生成论文摘要