Jointly Learning Agent and Lane Information for Multimodal Trajectory Prediction.
ICPR(2022)
摘要
Predicting the plausible future trajectories of nearby agents is a core challenge for the safety of Autonomous Vehicles and it mainly depends on two external cues: the dynamic neighbor agents and static scene context. Recent approaches have made great progress in characterizing the two cues separately. However, they ignore the correlation between the two cues and most of them are difficult to achieve map-adaptive prediction. In this paper, we use lane as scene data and propose a staged network that Jointly learning Agent and Lane information for Multimodal Trajectory Prediction (JAL-MTP). JAL-MTP use a Social to Lane (S2L) module to jointly represent the static lane and the dynamic motion of the neighboring agents as instance-level lane, a Recurrent Lane Attention (RLA) mechanism for utilizing the instance-level lanes to predict the map-adaptive future trajectories and two selectors to identify the typical and reasonable trajectories. The experiments conducted on the public Argoverse dataset demonstrate that JAL-MTP significantly outperforms the existing models in both quantitative and qualitative.
更多查看译文
关键词
accurate trajectories,agent dynamics,instance-level lanes,JAL-MTP use,Multimodal Trajectory Prediction,nearby agents,plausible future trajectories,reasonable multimodal trajectories,reasonable trajectories,Recurrent Lane Attention mechanism,scene context,social agents,typical trajectories
AI 理解论文
溯源树
样例
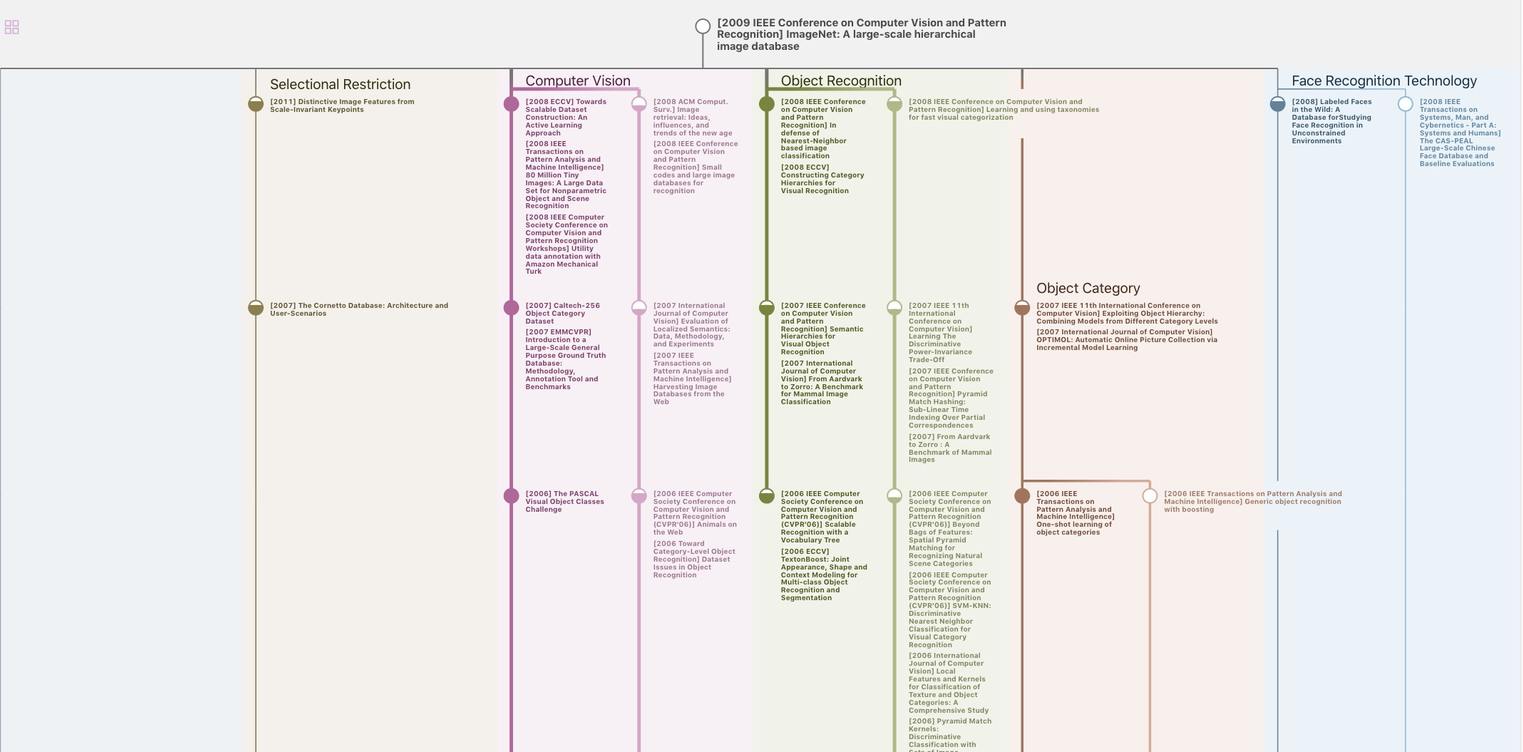
生成溯源树,研究论文发展脉络
Chat Paper
正在生成论文摘要