Variational Gibbs Inference for Statistical Model Estimation from Incomplete Data
JOURNAL OF MACHINE LEARNING RESEARCH(2023)
摘要
Statistical models are central to machine learning with broad applicability across a range of downstream tasks. The models are controlled by free parameters that are typically esti-mated from data by maximum-likelihood estimation or approximations thereof. However, when faced with real-world data sets many of the models run into a critical issue: they are formulated in terms of fully-observed data, whereas in practice the data sets are plagued with missing data. The theory of statistical model estimation from incomplete data is con-ceptually similar to the estimation of latent-variable models, where powerful tools such as variational inference (VI) exist. However, in contrast to standard latent-variable models, parameter estimation with incomplete data often requires estimating exponentially-many conditional distributions of the missing variables, hence making standard VI methods in -tractable. We address this gap by introducing variational Gibbs inference (VGI), a new general-purp ose method to estimate the parameters of statistical models from incomplete data. We validate VGI on a set of synthetic and real-world estimation tasks, estimating important machine learning models such as variational autoencoders and normalising flows from incomplete data. The proposed method, whilst general-purp ose, achieves competitive or better performance than existing model-specific estimation methods.
更多查看译文
关键词
statistical model estimation,variational inference,Gibbs sampling,missing data,amortised inference
AI 理解论文
溯源树
样例
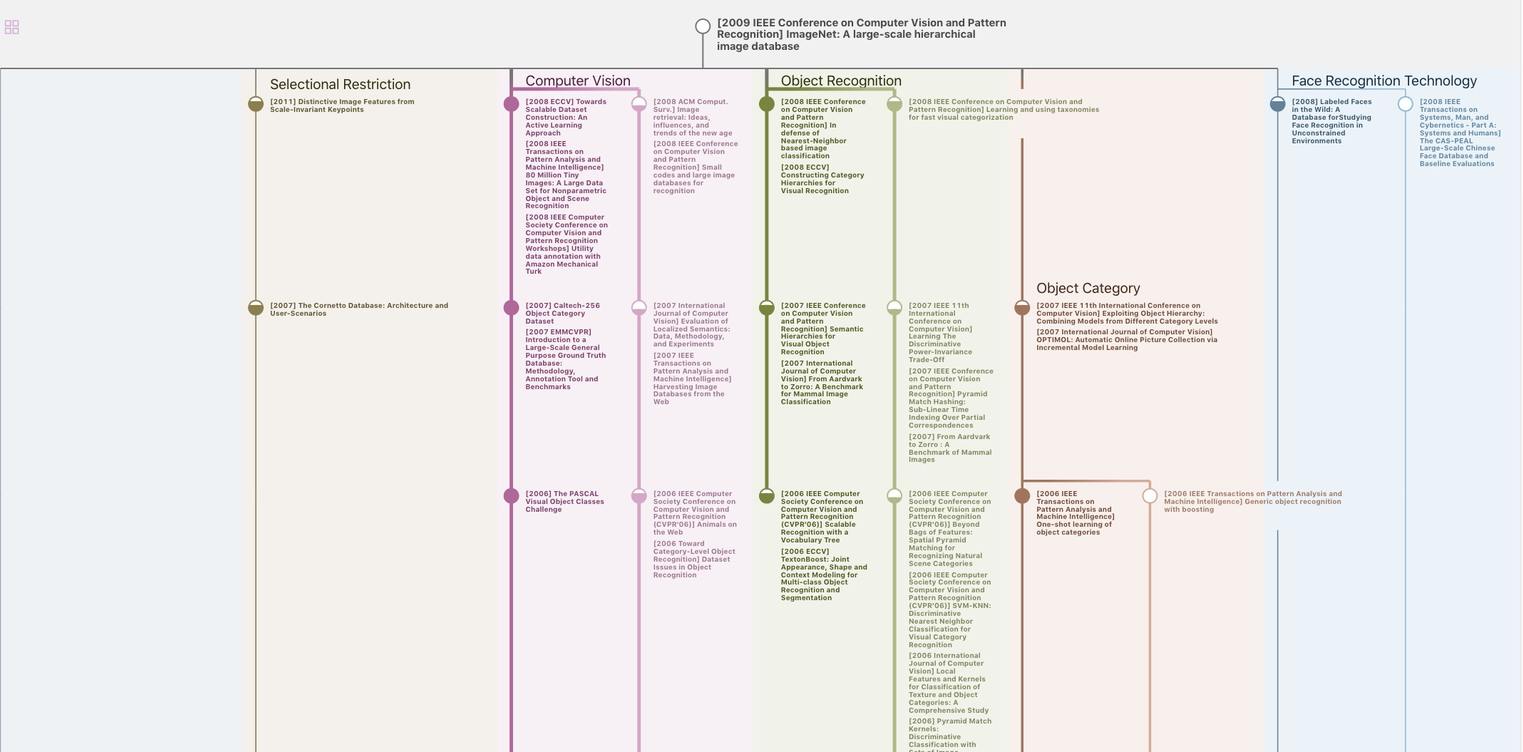
生成溯源树,研究论文发展脉络
Chat Paper
正在生成论文摘要