AutoHEnsGNN: Winning Solution to AutoGraph Challenge for KDD Cup 2020
2022 IEEE 38th International Conference on Data Engineering (ICDE)(2022)
摘要
Graph Neural Networks (GNNs) have become increasingly popular and achieved impressive results in many graph-based applications. However, extensive manual work and domain knowledge are required to design effective architectures, and the results of GNN models have high variance with different training setups, which limits the application of existing GNN models. In this paper, we present AutoHEnsGNN, a framework to build effective and robust models for graph tasks without any human intervention. AutoHEnsGNN won first place in the AutoGraph Challenge for KDD Cup 2020, and achieved the best rank score of five real-life datasets in the final phase. Given a task, AutoHEnsGNN first applies a fast proxy evaluation to automatically select a pool of promising GNN models. Then it builds a hierarchical ensemble framework: 1) We propose graph self-ensemble (GSE), which can reduce the variance of weight initialization and efficiently exploit the information of local and global neighborhoods; 2) Based on GSE, a weighted ensemble of different types of GNN models is used to effectively learn more discriminative node representations. To efficiently search the architectures and ensemble weights, we propose AutoHEnsGNN
Gradient
, which treats the architectures and ensemble weights as architecture parameters and uses gradient-based architecture search to obtain optimal configurations, and AutoHEnsGNN
Adaptive
, which can adaptively adjust the ensemble weight based on the model accuracy. Extensive experiments on node classification, graph classification, edge prediction and KDD Cup challenge demonstrate the effectiveness and generality of AutoHEnsGNN.
更多查看译文
关键词
graph neural network,automated,hierarchical ensemble,kddcup
AI 理解论文
溯源树
样例
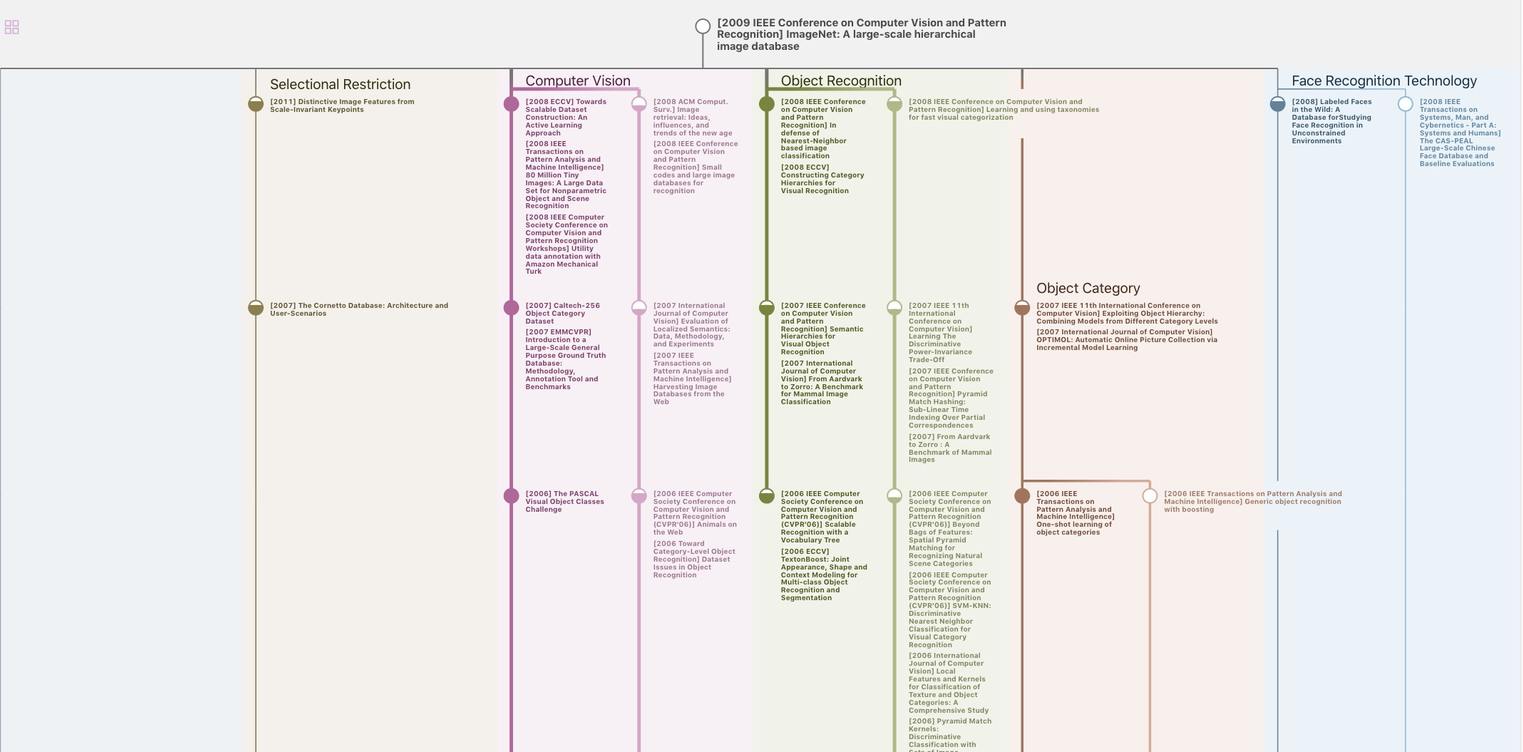
生成溯源树,研究论文发展脉络
Chat Paper
正在生成论文摘要