A Framework for Stochastic Optimization of Parameters for Integrative Modeling of Macromolecular Assemblies
LIFE-BASEL(2021)
摘要
Integrative modeling of macromolecular assemblies requires stochastic sampling, for example, via MCMC (Markov Chain Monte Carlo), since exhaustively enumerating all structural degrees of freedom is infeasible. MCMC-based methods usually require tuning several parameters, such as the move sizes for coarse-grained beads and rigid bodies, for sampling to be efficient and accurate. Currently, these parameters are tuned manually. To automate this process, we developed a general heuristic for derivative-free, global, stochastic, parallel, multiobjective optimization, termed StOP (Stochastic Optimization of Parameters) and applied it to optimize sampling-related parameters for the Integrative Modeling Platform (IMP). Given an integrative modeling setup, list of parameters to optimize, their domains, metrics that they influence, and the target ranges of these metrics, StOP produces the optimal values of these parameters. StOP is adaptable to the available computing capacity and converges quickly, allowing for the simultaneous optimization of a large number of parameters. However, it is not efficient at high dimensions and not guaranteed to find optima in complex landscapes. We demonstrate its performance on several examples of random functions, as well as on two integrative modeling examples, showing that StOP enhances the efficiency of sampling the posterior distribution, resulting in more good-scoring models and better sampling precision.
更多查看译文
关键词
integrative modeling, molecular simulations, MCMC, stochastic sampling, derivative-free optimization
AI 理解论文
溯源树
样例
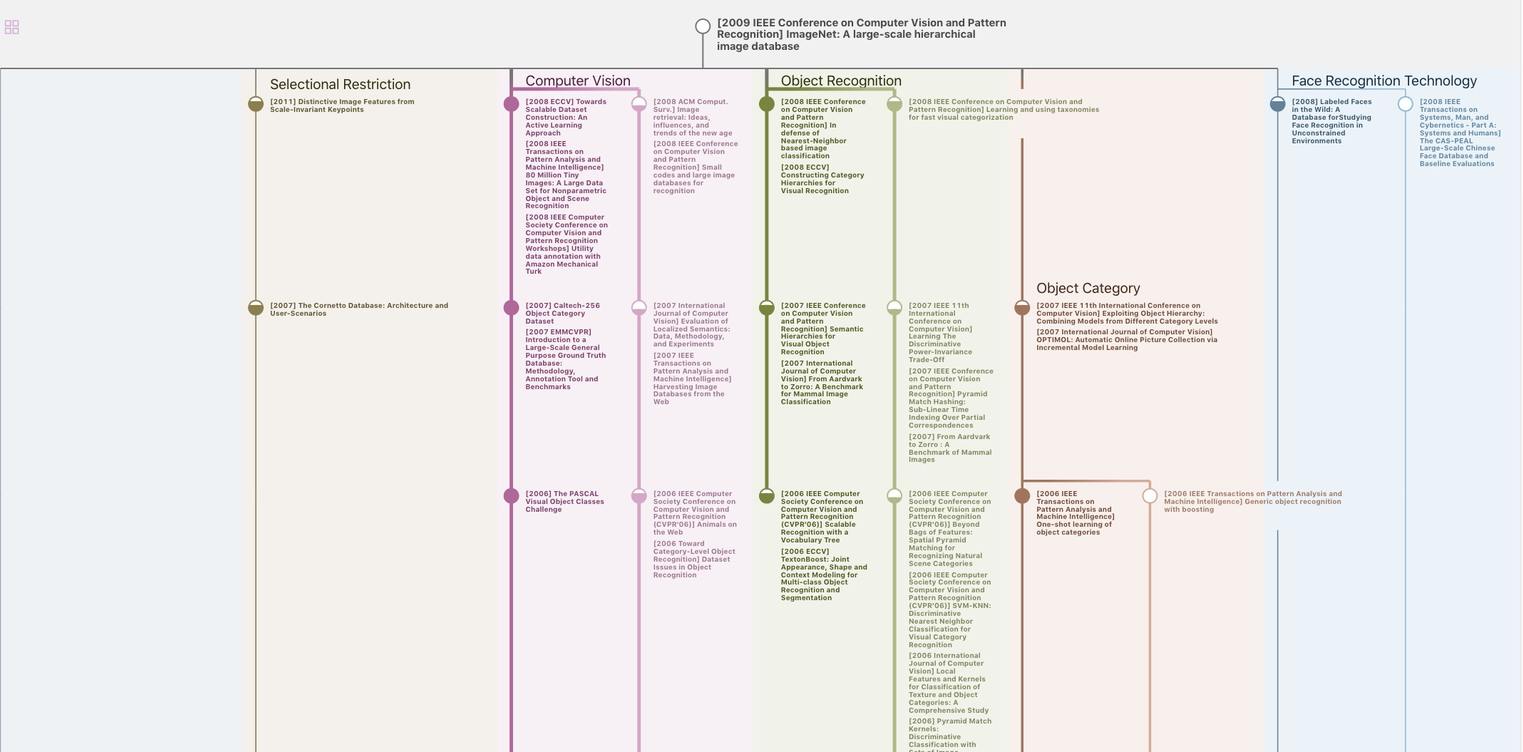
生成溯源树,研究论文发展脉络
Chat Paper
正在生成论文摘要