Prediction of Neoadjuvant Chemotherapy Response in Osteosarcoma Using Convolutional Neural Network of Tumor Center F-18-FDG PET Images
DIAGNOSTICS(2021)
摘要
We compared the accuracy of prediction of the response to neoadjuvant chemotherapy (NAC) in osteosarcoma patients between machine learning approaches of whole tumor utilizing fluorine-(18)fluorodeoxyglucose (F-18-FDG) uptake heterogeneity features and a convolutional neural network of the intratumor image region. In 105 patients with osteosarcoma, F-18-FDG positron emission tomography/computed tomography (PET/CT) images were acquired before (baseline PET0) and after NAC (PET1). Patients were divided into responders and non-responders about neoadjuvant chemotherapy. Quantitative F-18-FDG heterogeneity features were calculated using LIFEX version 4.0. Receiver operating characteristic (ROC) curve analysis of F-18-FDG uptake heterogeneity features was used to predict the response to NAC. Machine learning algorithms and 2-dimensional convolutional neural network (2D CNN) deep learning networks were estimated for predicting NAC response with the baseline PET0 images of the 105 patients. ML was performed using the entire tumor image. The accuracy of the 2D CNN prediction model was evaluated using total tumor slices, the center 20 slices, the center 10 slices, and center slice. A total number of 80 patients was used for k-fold validation by five groups with 16 patients. The CNN network test accuracy estimation was performed using 25 patients. The areas under the ROC curves (AUCs) for baseline PET maximum standardized uptake value (SUVmax), total lesion glycolysis (TLG), metabolic tumor volume (MTV), and gray level size zone matrix (GLSZM) were 0.532, 0.507, 0.510, and 0.626, respectively. The texture features test accuracy of machine learning by random forest and support vector machine were 0.55 and 0. 54, respectively. The k-fold validation accuracy and validation accuracy were 0.968 & PLUSMN; 0.01 and 0.610 & PLUSMN; 0.04, respectively. The test accuracy of total tumor slices, the center 20 slices, center 10 slices, and center slices were 0.625, 0.616, 0.628, and 0.760, respectively. The prediction model for NAC response with baseline PET0 texture features machine learning estimated a poor outcome, but the 2D CNN network using F-18-FDG baseline PET0 images could predict the treatment response before prior chemotherapy in osteosarcoma. Additionally, using the 2D CNN prediction model using a tumor center slice of F-18-FDG PET images before NAC can help decide whether to perform NAC to treat osteosarcoma patients.
更多查看译文
关键词
F-18-FDG heterogeneity, convolutional neural network, chemotherapy response, osteosarcoma, machine learning
AI 理解论文
溯源树
样例
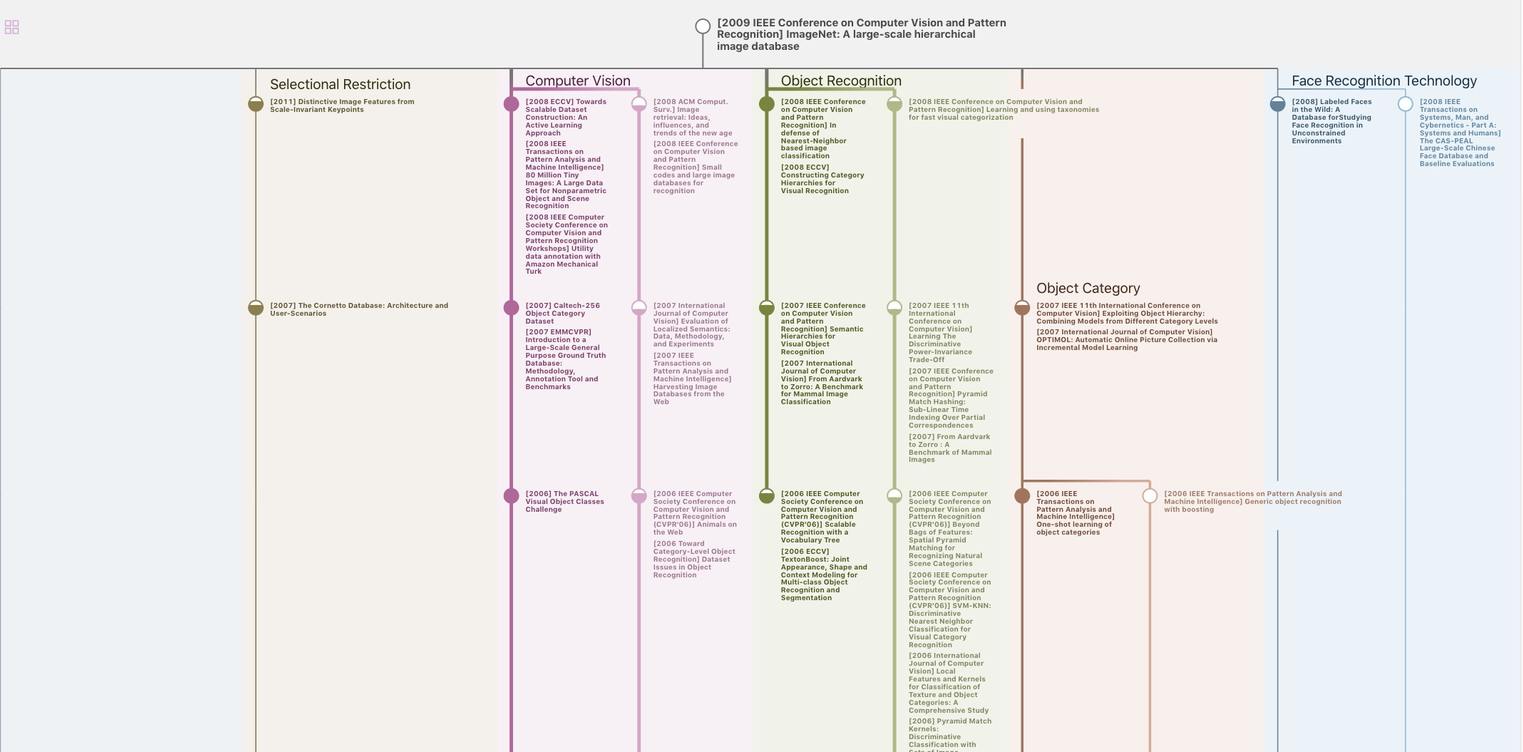
生成溯源树,研究论文发展脉络
Chat Paper
正在生成论文摘要