Neural Decoding of EEG Signals with Machine Learning: A Systematic Review
BRAIN SCIENCES(2021)
摘要
Electroencephalography (EEG) is a non-invasive technique used to record the brain's evoked and induced electrical activity from the scalp. Artificial intelligence, particularly machine learning (ML) and deep learning (DL) algorithms, are increasingly being applied to EEG data for pattern analysis, group membership classification, and brain-computer interface purposes. This study aimed to systematically review recent advances in ML and DL supervised models for decoding and classifying EEG signals. Moreover, this article provides a comprehensive review of the state-of-the-art techniques used for EEG signal preprocessing and feature extraction. To this end, several academic databases were searched to explore relevant studies from the year 2000 to the present. Our results showed that the application of ML and DL in both mental workload and motor imagery tasks has received substantial attention in recent years. A total of 75% of DL studies applied convolutional neural networks with various learning algorithms, and 36% of ML studies achieved competitive accuracy by using a support vector machine algorithm. Wavelet transform was found to be the most common feature extraction method used for all types of tasks. We further examined the specific feature extraction methods and end classifier recommendations discovered in this systematic review.
更多查看译文
关键词
brain signals classification, EEG, deep learning, machine learning, review
AI 理解论文
溯源树
样例
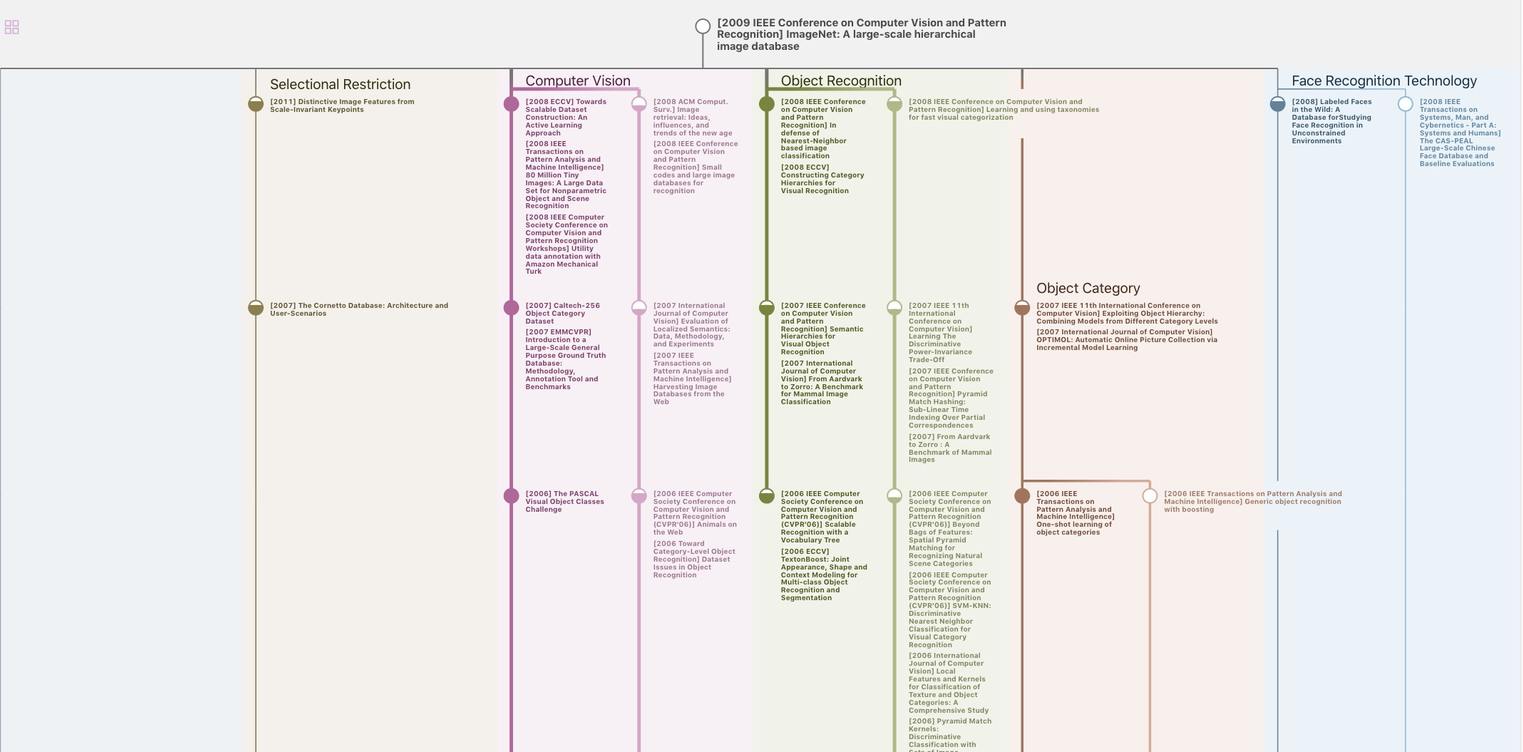
生成溯源树,研究论文发展脉络
Chat Paper
正在生成论文摘要