Effective End-to-End Deep Learning Process in Medical Imaging Using Independent Task Learning: Application for Diagnosis of Maxillary Sinusitis.
Yonsei medical journal(2021)
Abstract
PURPOSE:This study aimed to propose an effective end-to-end process in medical imaging using an independent task learning (ITL) algorithm and to evaluate its performance in maxillary sinusitis applications.
MATERIALS AND METHODS:For the internal dataset, 2122 Waters' view X-ray images, which included 1376 normal and 746 sinusitis images, were divided into training (n=1824) and test (n=298) datasets. For external validation, 700 images, including 379 normal and 321 sinusitis images, from three different institutions were evaluated. To develop the automatic diagnosis system algorithm, four processing steps were performed: 1) preprocessing for ITL, 2) facial patch detection, 3) maxillary sinusitis detection, and 4) a localization report with the sinusitis detector.
RESULTS:The accuracy of facial patch detection, which was the first step in the end-to-end algorithm, was 100%, 100%, 99.5%, and 97.5% for the internal set and external validation sets #1, #2, and #3, respectively. The accuracy and area under the receiver operating characteristic curve (AUC) of maxillary sinusitis detection were 88.93% (0.89), 91.67% (0.90), 90.45% (0.86), and 85.13% (0.85) for the internal set and external validation sets #1, #2, and #3, respectively. The accuracy and AUC of the fully automatic sinusitis diagnosis system, including site localization, were 79.87% (0.80), 84.67% (0.82), 83.92% (0.82), and 73.85% (0.74) for the internal set and external validation sets #1, #2, and #3, respectively.
CONCLUSION:ITL application for maxillary sinusitis showed reasonable performance in internal and external validation tests, compared with applications used in previous studies.
MoreTranslated text
AI Read Science
Must-Reading Tree
Example
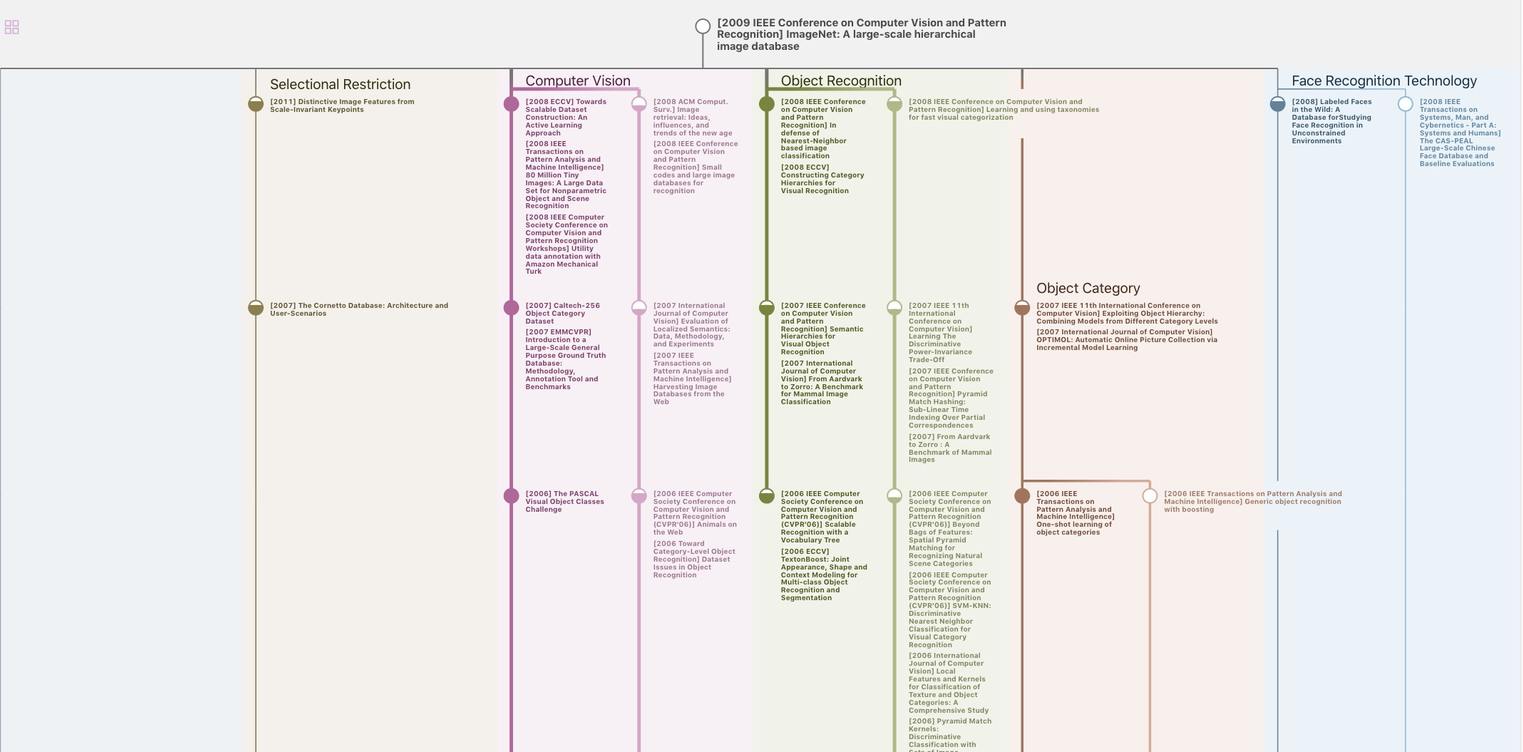
Generate MRT to find the research sequence of this paper
Chat Paper
Summary is being generated by the instructions you defined