Residual Dilated U-net For The Segmentation Of COVID-19 Infection From CT Images.
2021 IEEE/CVF International Conference on Computer Vision Workshops (ICCVW)(2021)
摘要
Medical imaging such as computed tomography (CT) plays a critical role in the global fight against COVID19. Computer-aided platforms have emerged to help radiologists diagnose and track disease prognosis. In this paper, we introduce an automated deep-learning segmentation model, which builds upon the current U-net model, however, leverages the strengths of long and short skip connections. We complemented the long skip connections with a cascaded dilated convolution module that learns multi-scale context information, compensates the reduction in receptive fields, and reduces the disparity between encoded and decoded features. The short connections are considered in utilizing residual blocks as the basic building blocks for our model. They ease the training process, reduce the degradation problem, and propagate the low fine details. This enables the model to perform well in capturing smaller regions of interest. Furthermore, each residual block is followed by a squeeze and excitation unit, which stimulates informative features and suppresses less important ones, thus improving the overall feature representation. After extensive experimentation with a dataset of 1705 COVID-19 axial CT images, we demonstrate that performance gains can be achieved when deep learning modules are integrated with the basic U-net model. Experimental results show that our model outperformed the basic U-net and ResDUnet model by 8.1% and 1.9% in dice similarity, respectively. Our model provided a dice similarity measure of 85.3%, with a slight increase in trainable parameters, thus demonstrating a huge potential for use in the clinical domain.
更多查看译文
关键词
COVID-19,Deep learning,Training,Image segmentation,Convolution,Shape,Computed tomography
AI 理解论文
溯源树
样例
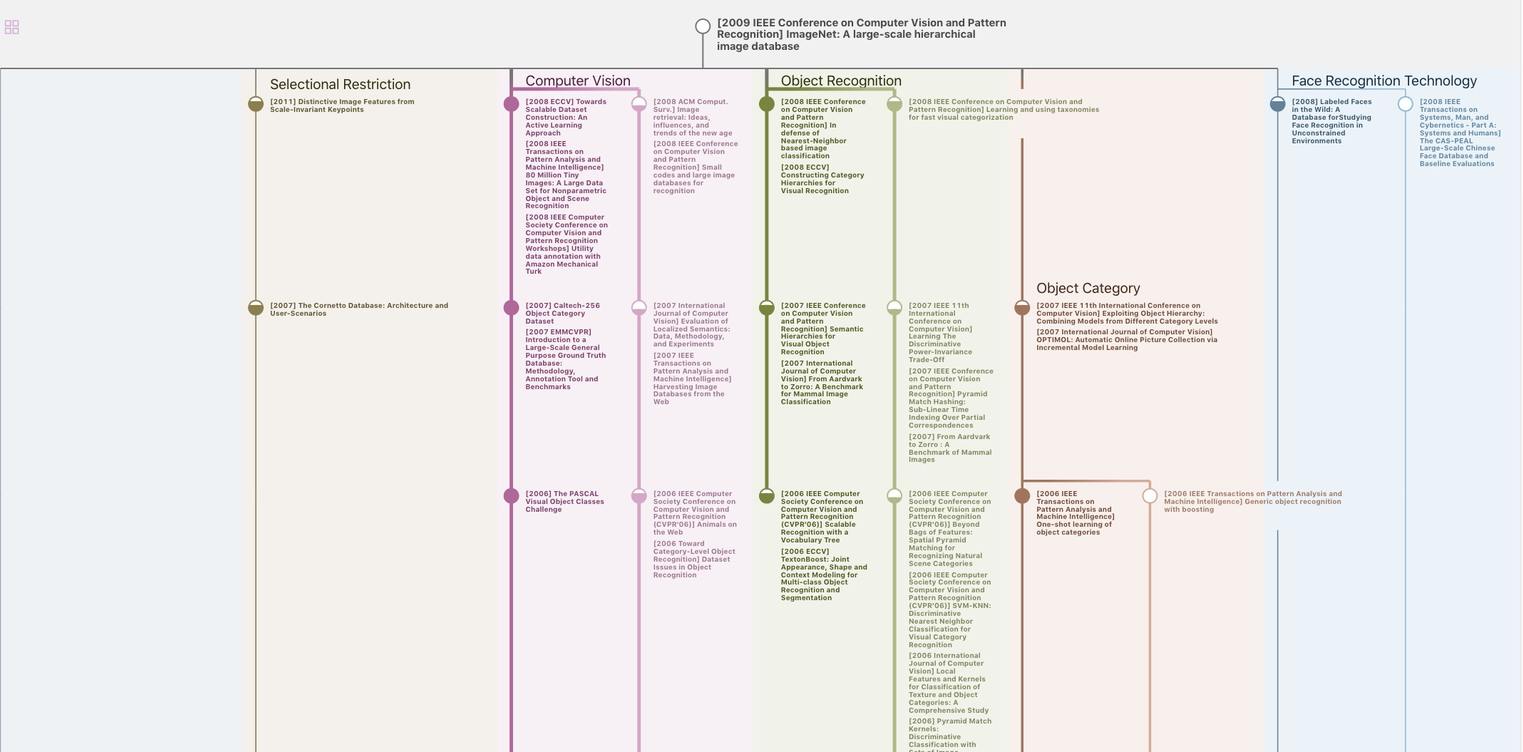
生成溯源树,研究论文发展脉络
Chat Paper
正在生成论文摘要