Leveraging Batch Normalization for Vision Transformers.
2021 IEEE/CVF International Conference on Computer Vision Workshops (ICCVW)(2021)
Abstract
Transformer-based vision architectures have attracted great attention because of the strong performance over the convolutional neural networks (CNNs). Inherited from the NLP tasks, the architectures take Layer Normalization (LN) as a default normalization technique. On the other side, previous vision models, i.e., CNNs, treat Batch Normalization (BN) as a de facto standard, with the merits of faster inference than other normalization layers due to an avoidance of calculating the mean and variance statistics during inference, as well as better regularization effects during training. In this paper, we aim to introduce Batch Normalization to Transformer-based vision architectures. Our initial exploration reveals frequent crashes in model training when directly replacing all LN layers with BN, contributing to the un-normalized feed forward network (FFN) blocks. We therefore propose to add a BN layer in-between the two linear layers in the FFN block where stabilized training statistics are observed, resulting in a pure BN-based architecture. Our experiments proved that our resulting approach is as effective as the LN-based counterpart and is about 20% faster.
MoreTranslated text
Key words
Training,Computer vision,Conferences,Computer architecture,Transformers,Computer crashes,Feeds
AI Read Science
Must-Reading Tree
Example
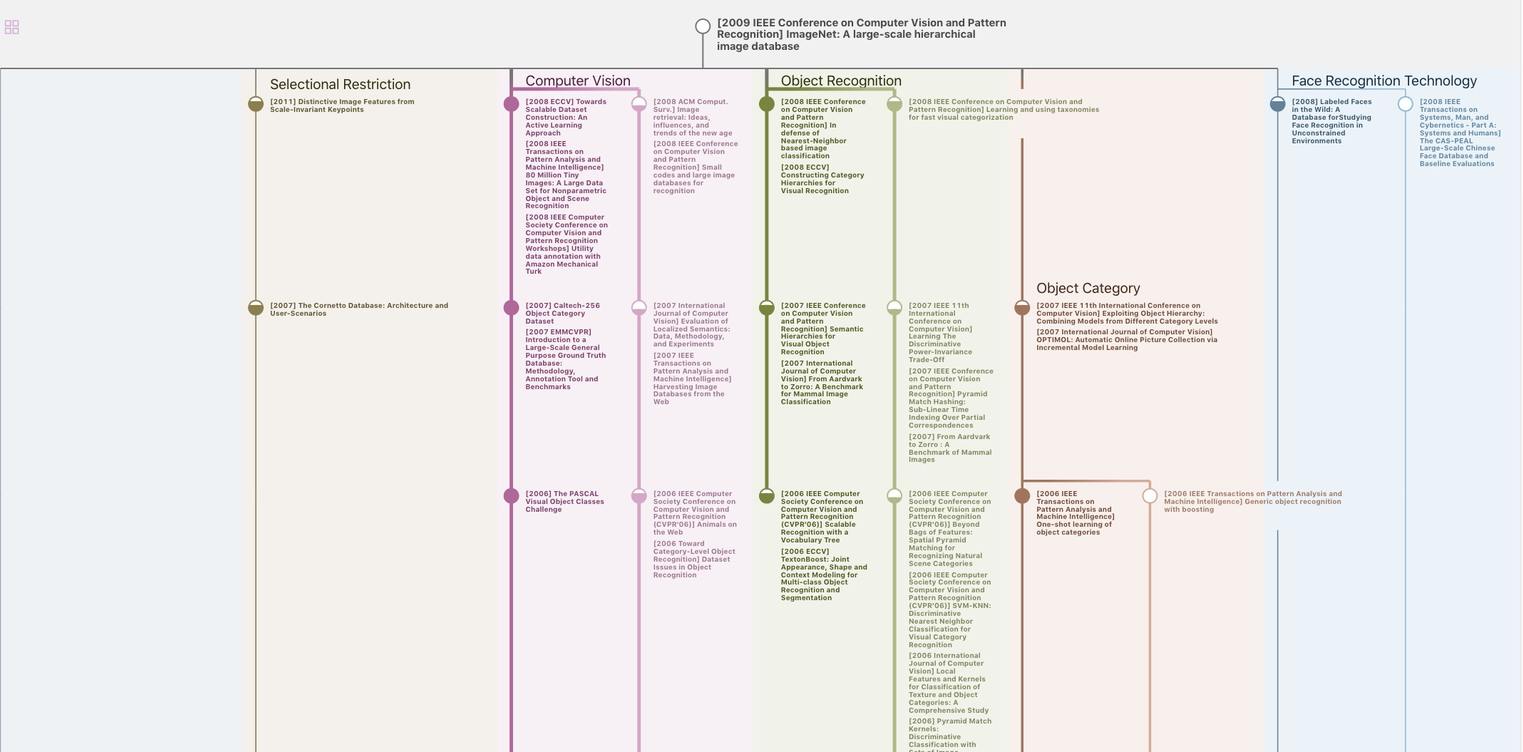
Generate MRT to find the research sequence of this paper
Chat Paper
Summary is being generated by the instructions you defined