OH-Former: Omni-Relational High-Order Transformer for Person Re-Identification
arxiv(2021)
摘要
Transformers have shown preferable performance on many vision tasks. However, for the task of person re-identification (ReID), vanilla transformers leave the rich contexts on high-order feature relations under-exploited and deteriorate local feature details, which are insufficient due to the dramatic variations of pedestrians. In this work, we propose an Omni-Relational High-Order Transformer (OH-Former) to model omni-relational features for ReID. First, to strengthen the capacity of visual representation, instead of obtaining the attention matrix based on pairs of queries and isolated keys at each spatial location, we take a step further to model high-order statistics information for the non-local mechanism. We share the attention weights in the corresponding layer of each order with a prior mixing mechanism to reduce the computation cost. Then, a convolution-based local relation perception module is proposed to extract the local relations and 2D position information. The experimental results of our model are superior promising, which show state-of-the-art performance on Market-1501, DukeMTMC, MSMT17 and Occluded-Duke datasets.
更多查看译文
关键词
transformer,person
AI 理解论文
溯源树
样例
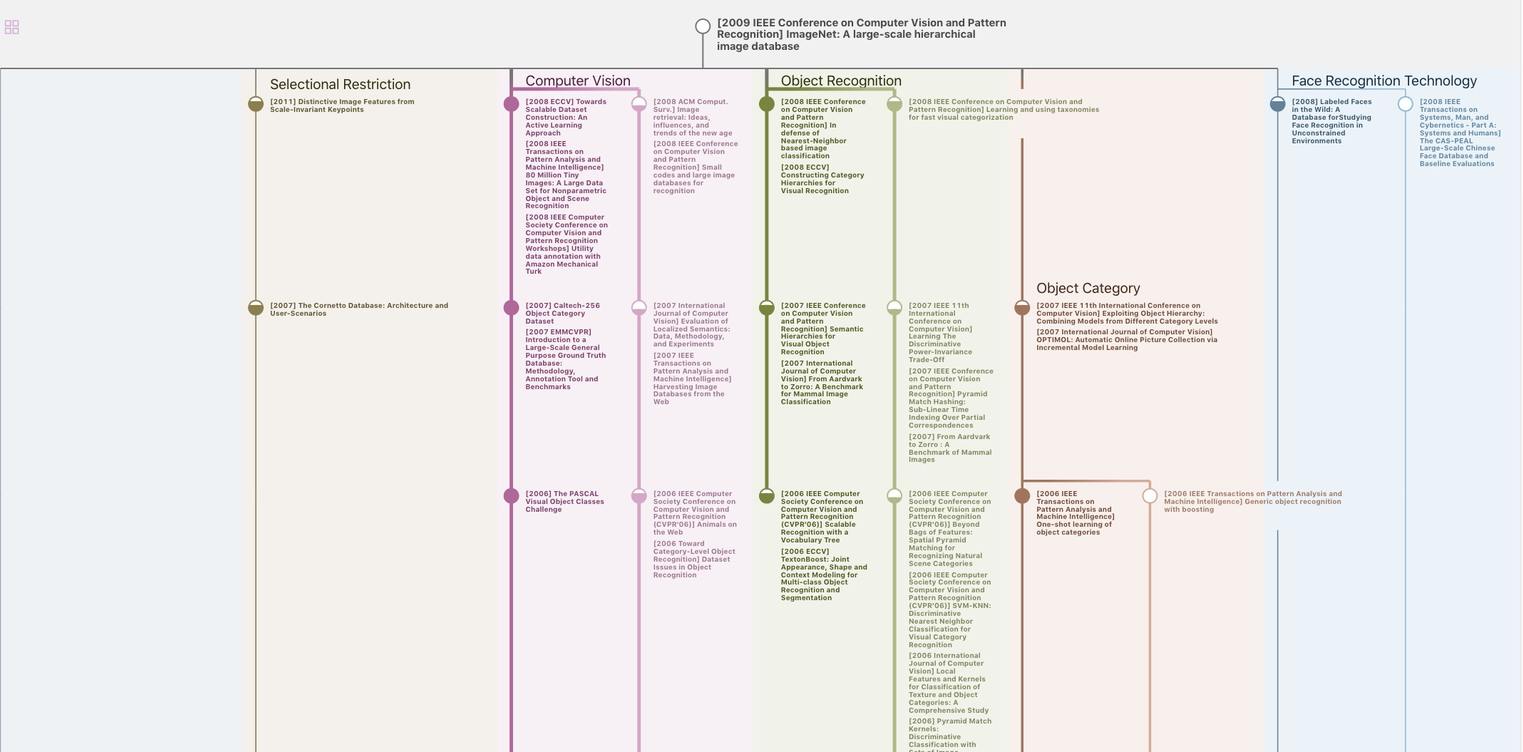
生成溯源树,研究论文发展脉络
Chat Paper
正在生成论文摘要