Measurement That Matches Theory: Theory-Driven Identification in IRT Models
arxiv(2022)
摘要
Measurement bridges theory and empirics. Without measures that appropriately capture theoretical concepts, description will fail to represent reality and true causal inference will be impossible. Yet, the social sciences traffic in complex concepts and their measurement is difficult. Item Response Theory (IRT) models reduce variation in multiple variables to continuous variation along one or more latent dimensions intended to capture key theoretical concepts. Unfortunately, those latent dimensions have no intrinsic conceptual meaning. Partial solutions to that problem include limiting the number of dimensions to one or assigning meaning post-analysis, but either can lead to potential bias and a lack of reliability across data sources. We propose, detail, and validate a semi-supervised approach employing Bayesian Item Response Theory on multiple latent dimensions and binary data. Our approach, which we validate on simulated and real data, yields conceptually meaningful latent dimensions that are reliable across different data sources without additional exogenous assumptions.
更多查看译文
关键词
irt models,measurement,theory-driven
AI 理解论文
溯源树
样例
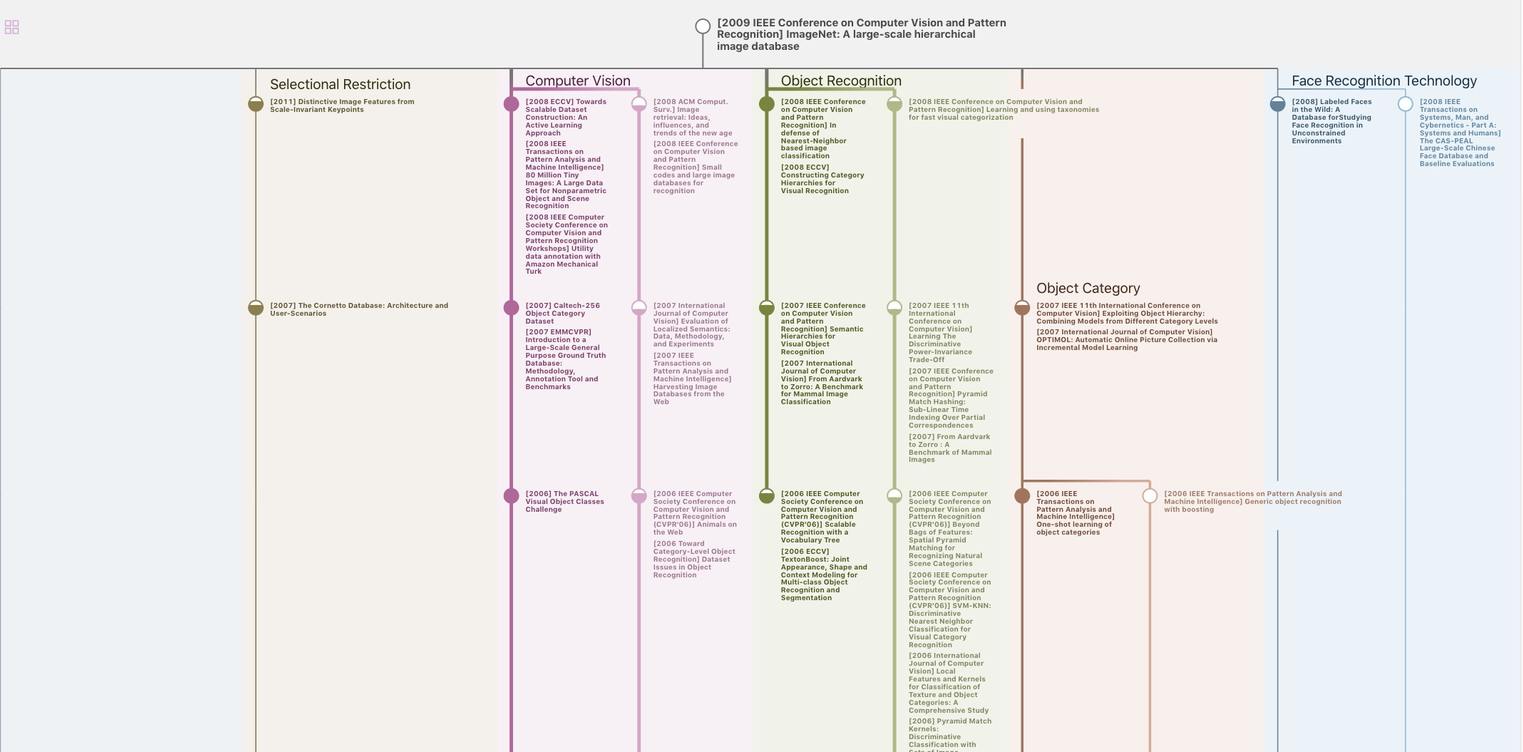
生成溯源树,研究论文发展脉络
Chat Paper
正在生成论文摘要