Hierarchical Graph Networks for 3D Human Pose Estimation.
British Machine Vision Conference(2021)
摘要
Recent 2D-to-3D human pose estimation works tend to utilize the graph structure formed by the topology of the human skeleton. However, we argue that this skeletal topology is too sparse to reflect the body structure and suffer from serious 2D-to-3D ambiguity problem. To overcome these weaknesses, we propose a novel graph convolution network architecture, Hierarchical Graph Networks (HGN). It is based on denser graph topology generated by our multi-scale graph structure building strategy, thus providing more delicate geometric information. The proposed architecture contains three sparse-to-fine representation subnetworks organized in parallel, in which multi-scale graph-structured features are processed and exchange information through a novel feature fusion strategy, leading to rich hierarchical representations. We also introduce a 3D coarse mesh constraint to further boost detail-related feature learning. Extensive experiments demonstrate that our HGN achieves the state-of-the art performance with reduced network parameters
更多查看译文
关键词
3d human pose estimation,hierarchical graph networks
AI 理解论文
溯源树
样例
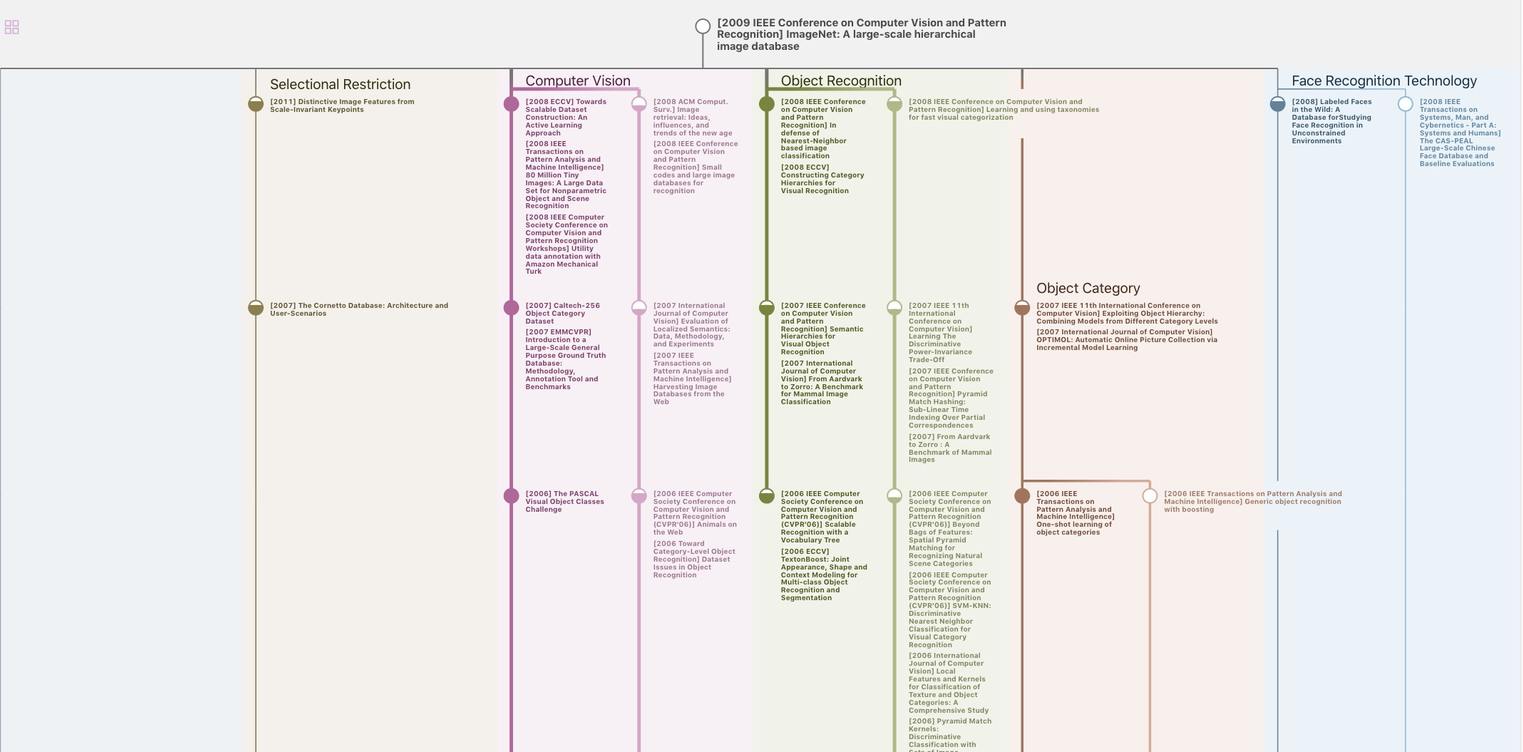
生成溯源树,研究论文发展脉络
Chat Paper
正在生成论文摘要