Multi-Modal Deep Learning for Weeds Detection in Wheat Field Based on RGB-D Images
FRONTIERS IN PLANT SCIENCE(2021)
摘要
Single-modal images carry limited information for features representation, and RGB images fail to detect grass weeds in wheat fields because of their similarity to wheat in shape. We propose a framework based on multi-modal information fusion for accurate detection of weeds in wheat fields in a natural environment, overcoming the limitation of single modality in weeds detection. Firstly, we recode the single-channel depth image into a new three-channel image like the structure of RGB image, which is suitable for feature extraction of convolutional neural network (CNN). Secondly, the multi-scale object detection is realized by fusing the feature maps output by different convolutional layers. The three-channel network structure is designed to take into account the independence of RGB and depth information, respectively, and the complementarity of multi-modal information, and the integrated learning is carried out by weight allocation at the decision level to realize the effective fusion of multi-modal information. The experimental results show that compared with the weed detection method based on RGB image, the accuracy of our method is significantly improved. Experiments with integrated learning shows that mean average precision (mAP) of 36.1% for grass weeds and 42.9% for broad-leaf weeds, and the overall detection precision, as indicated by intersection over ground truth (IoG), is 89.3%, with weights of RGB and depth images at alpha = 0.4 and beta = 0.3. The results suggest that our methods can accurately detect the dominant species of weeds in wheat fields, and that multi-modal fusion can effectively improve object detection performance.
更多查看译文
关键词
weeds detection,RGB-D image,multi-modal deep learning,machine learning,three-channel network
AI 理解论文
溯源树
样例
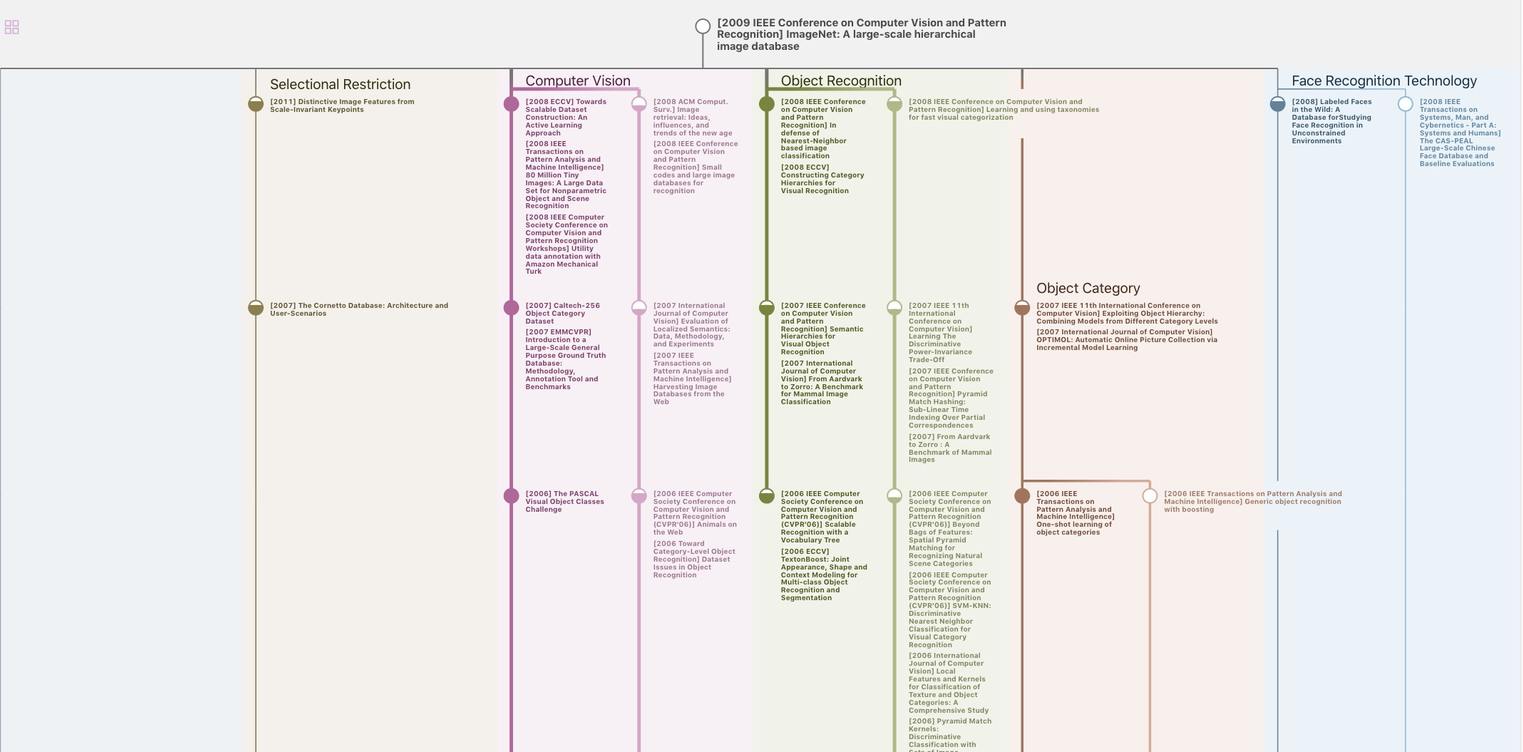
生成溯源树,研究论文发展脉络
Chat Paper
正在生成论文摘要