Transfer Learning with Gaussian Processes for Bayesian Optimization
INTERNATIONAL CONFERENCE ON ARTIFICIAL INTELLIGENCE AND STATISTICS, VOL 151(2022)
摘要
Bayesian optimization is a powerful paradigm to optimize black-box functions based on scarce and noisy data. Its data efficiency can be further improved by transfer learning from related tasks. While recent transfer models meta-learn a prior based on large amount of data, in the low-data regime methods that exploit the closed-form posterior of Gaussian processes (GPs) have an advantage. In this setting, several analytically tractable transfer-model posteriors have been proposed, but the relative advantages of these methods are not well understood. In this paper, we provide a unified view on hierarchical GP models for transfer learning, which allows us to analyze the relationship between methods. As part of the analysis, we develop a novel closed-form boosted GP transfer model that fits between existing approaches in terms of complexity. We evaluate the performance of the different approaches in large-scale experiments and highlight strengths and weaknesses of the different transfer-learning methods.
更多查看译文
关键词
bayesian optimization,transfer learning,gaussian processes
AI 理解论文
溯源树
样例
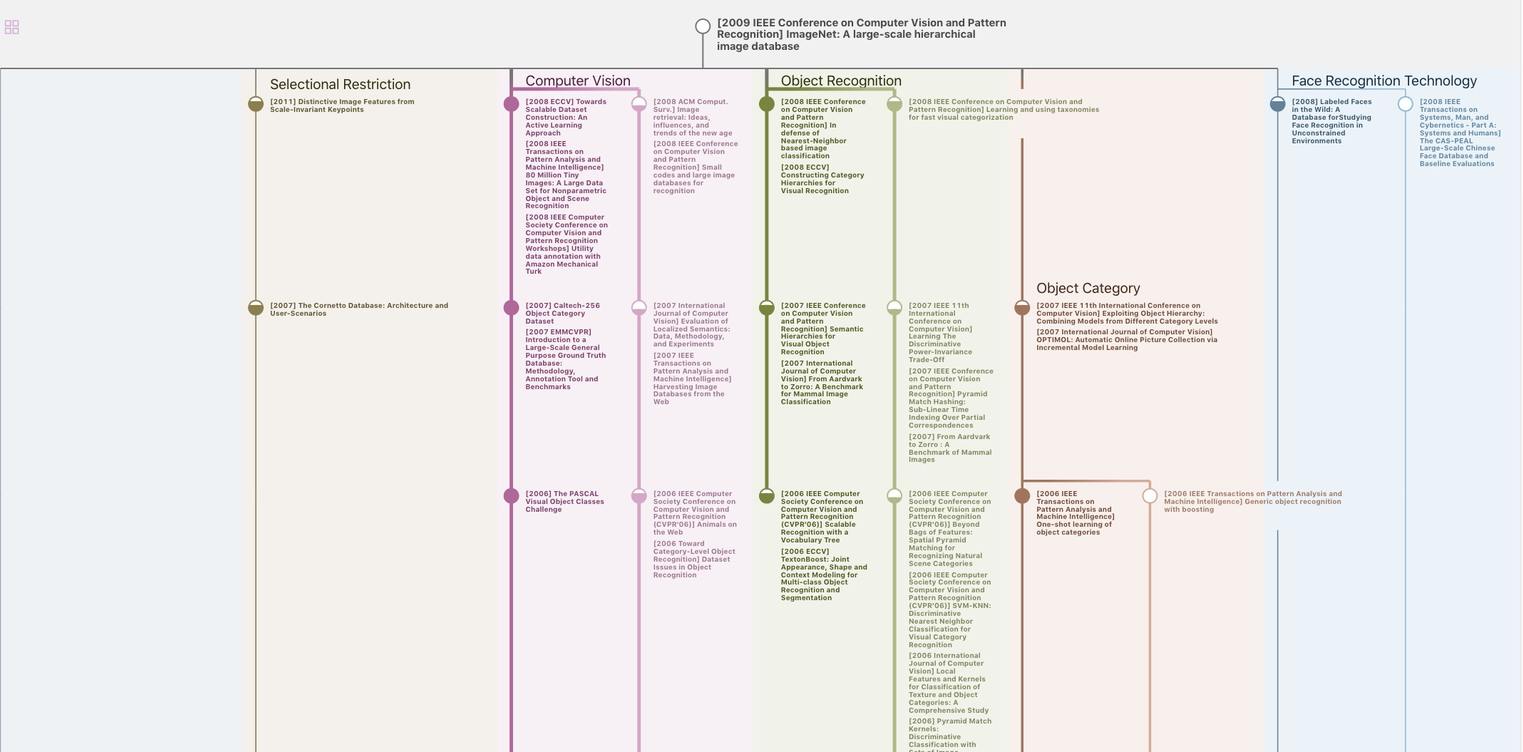
生成溯源树,研究论文发展脉络
Chat Paper
正在生成论文摘要