GCR: Gradient Coreset based Replay Buffer Selection for Continual Learning
2022 IEEE/CVF Conference on Computer Vision and Pattern Recognition (CVPR)(2022)
摘要
Continual learning (CL) aims to develop techniques by which a single model adapts to an increasing number of tasks encountered sequentially, thereby potentially leveraging learnings across tasks in a resource-efficient manner. A major challenge for CL systems is catastrophic forgetting, where earlier tasks are forgotten while learning a new task. To address this, replay-based CL approaches maintain and repeatedly retrain on a small buffer of data selected across encountered tasks. We propose Gradient Coreset Replay (GCR), a novel strategy for replay buffer selection and update using a carefully designed optimization criterion. Specifically, we select and maintain a ‘coreset’ that closely approximates the gradient of all the data seen so far with respect to current model parameters, and discuss key strategies needed for its effective application to the continual learning setting. We show significant gains (2%-4% absolute) over the state-of-the-art in the well-studied offline continual learning setting. Our findings also effectively transfer to online / streaming CL settings, showing up to 5% gains over existing approaches. Finally, we demonstrate the value of supervised contrastive loss for continual learning, which yields a cumulative gain of up to 5% accuracy when combined with our subset selection strategy.
更多查看译文
关键词
Machine learning, Computer vision theory, Efficient learning and inferences, Optimization methods
AI 理解论文
溯源树
样例
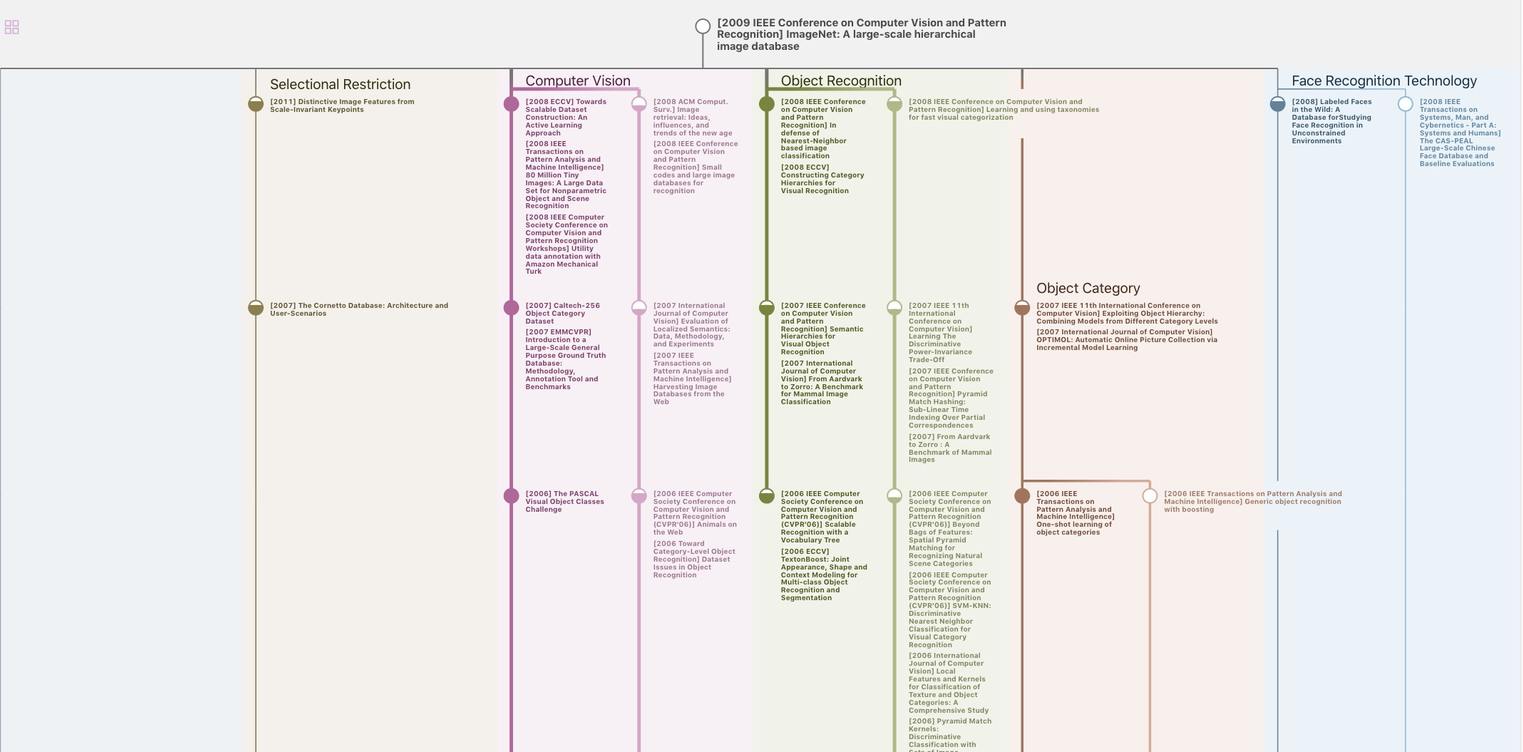
生成溯源树,研究论文发展脉络
Chat Paper
正在生成论文摘要