Improving Semantic Image Segmentation via Label Fusion in Semantically Textured Meshes
PROCEEDINGS OF THE 17TH INTERNATIONAL JOINT CONFERENCE ON COMPUTER VISION, IMAGING AND COMPUTER GRAPHICS THEORY AND APPLICATIONS (VISAPP), VOL 5(2022)
摘要
Models for semantic segmentation require a large amount of hand-labeled training data which is costly and time-consuming to produce. For this purpose, we present a label fusion framework that is capable of improving semantic pixel labels of video sequences in an unsupervised manner. We make use of a 3D mesh representation of the environment and fuse the predictions of different frames into a consistent representation using semantic mesh textures. Rendering the semantic mesh using the original intrinsic and extrinsic camera parameters yields a set of improved semantic segmentation images. Due to our optimized CUDA implementation, we are able to exploit the entire c-dimensional probability distribution of annotations over c classes in an uncertainty-aware manner. We evaluate our method on the Scannet dataset where we improve annotations produced by the state-of-the-art segmentation network ESANet from 52.05% to 58.25% pixel accuracy. We publish the source code of our framework online to foster future research in this area (https://github.com/fferflo/semantic-meshes). To the best of our knowledge, this is the first publicly available label fusion framework for semantic image segmentation based on meshes with semantic textures.
更多查看译文
关键词
Semantic Segmentation, Mesh Reconstruction, Label Fusion
AI 理解论文
溯源树
样例
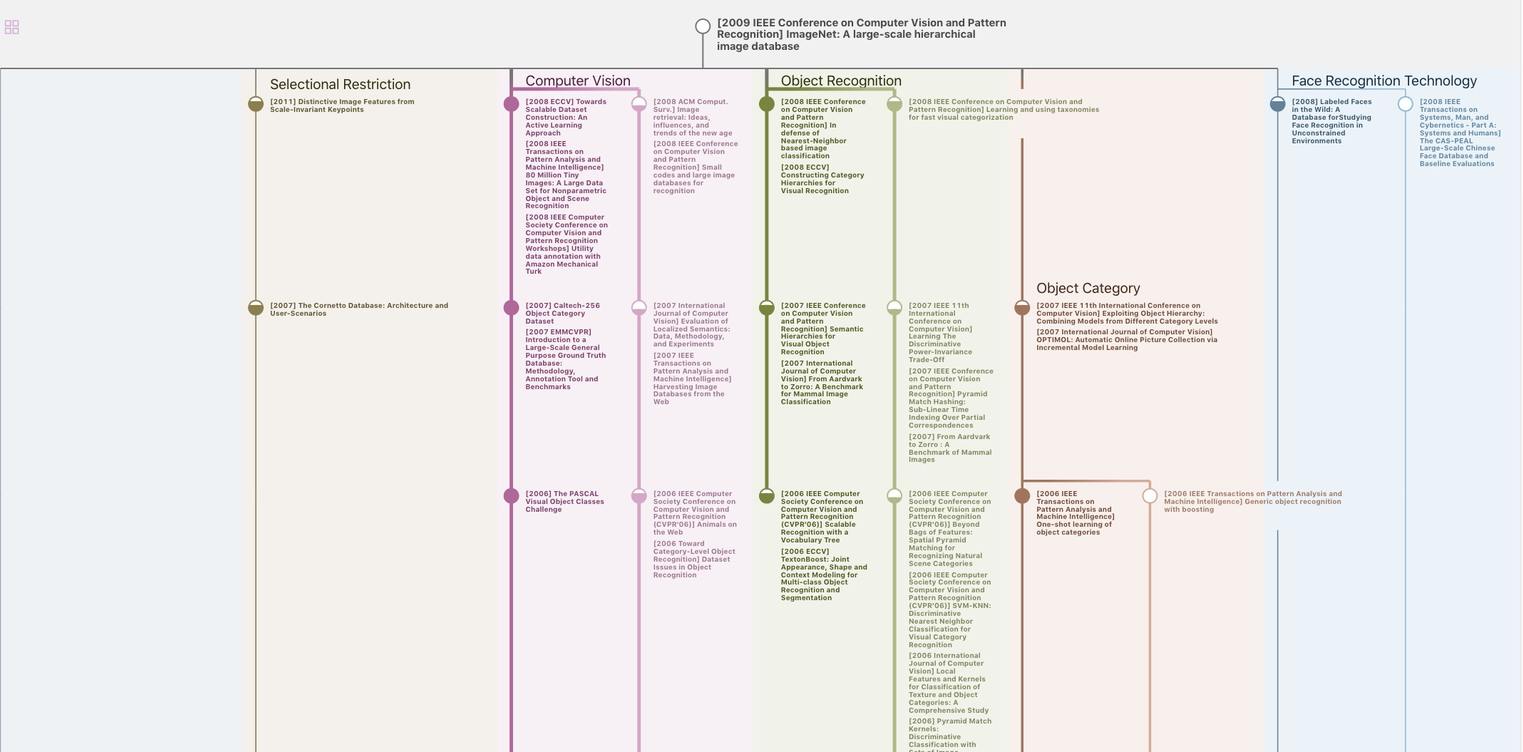
生成溯源树,研究论文发展脉络
Chat Paper
正在生成论文摘要