Decorrelated Variable Importance
JOURNAL OF MACHINE LEARNING RESEARCH(2024)
摘要
Because of the widespread use of black box prediction methods such as random forests and neural nets, there is renewed interest in developing methods for quantifying variable importance as part of the broader goal of interpretable prediction. A popular approach is to define a variable importance parameter - known as LOCO (Leave Out COvariates) - based on dropping covariates from a regression model. This is essentially a nonparametric version of R-2. This parameter is very general and can be estimated nonparametrically, but it can be hard to interpret because it is affected by correlation between covariates. We propose a method for mitigating the effect of correlation by defining a modified version of LOCO. This new parameter is difficult to estimate nonparametrically, but we show how to estimate it using semiparametric models.
更多查看译文
关键词
Correlation,Nonparametric Estimators,Prediction,Variable Importance
AI 理解论文
溯源树
样例
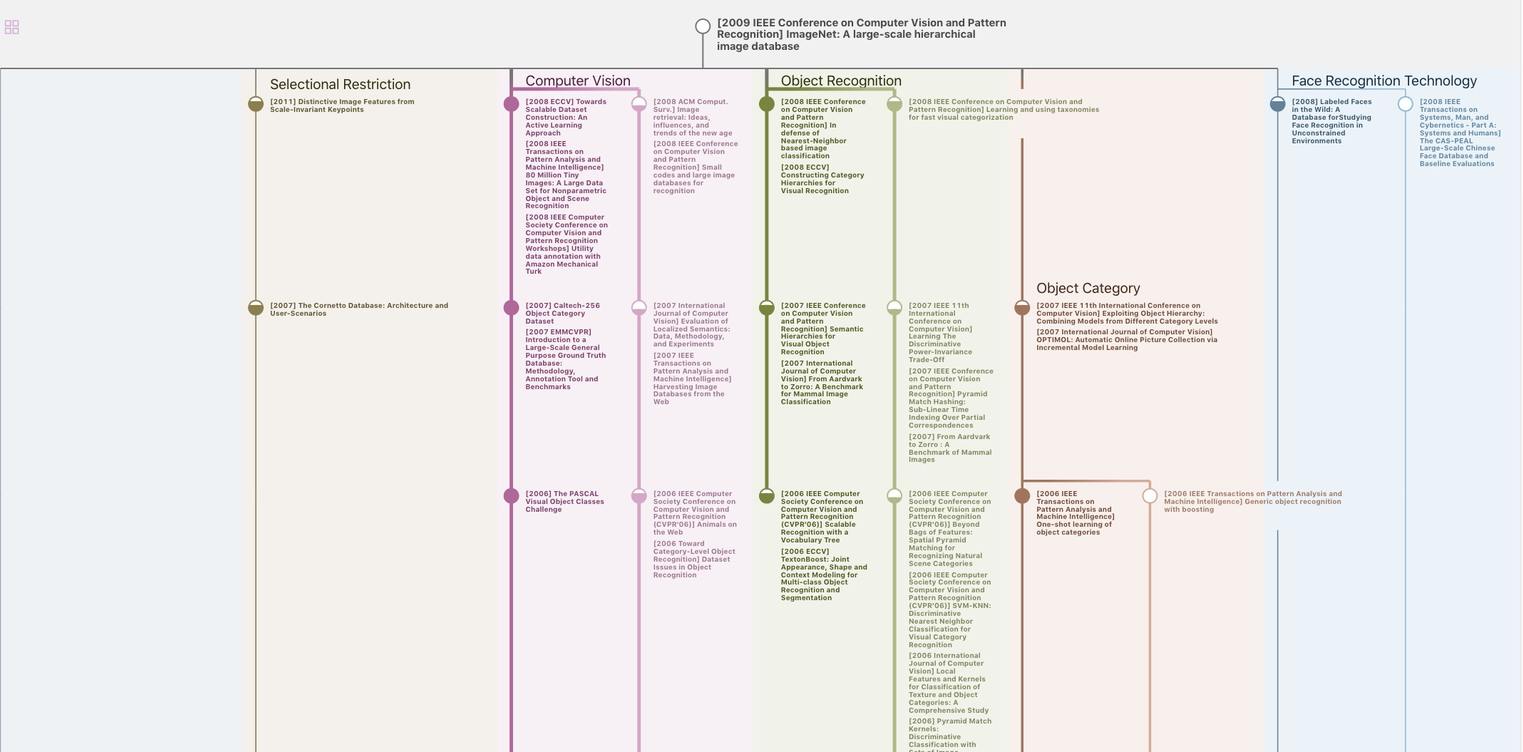
生成溯源树,研究论文发展脉络
Chat Paper
正在生成论文摘要