HybNet: A Hybrid Deep Learning -- Matched Filter Approach for IoT Signal Detection
CoRR(2022)
摘要
Random access schemes are widely used in IoT wireless access networks to accommodate simplicity and power consumption constraints. As a result, the interference arising from overlapping IoT transmissions is a significant issue in such networks. Traditional signal detection methods are based on the well-established matched filter using the complex conjugate of the signal, which is proven as the optimal filter under additive white Gaussian noise. However, with the colored interference arising from the overlapping IoT transmissions, deep learning approaches are being considered as a better alternative. In this paper, we present a hybrid framework, HybNet, that switches between deep learning and match filter pathways based on the detected interference level. This helps the detector work in a broader range of conditions, optimally leveraging the matched filter and deep learning robustness. We compare the performance of several possible data modalities and detection architectures concerning the interference-to-noise ratio, demonstrating that the proposed HybNet surpasses the complex conjugate matched filter performance under interference-limited scenarios.
更多查看译文
关键词
Internet of Things,LoRa,deep learning,chirp spread spectrum,convolutional neural network,matched filter,signal detection,interference
AI 理解论文
溯源树
样例
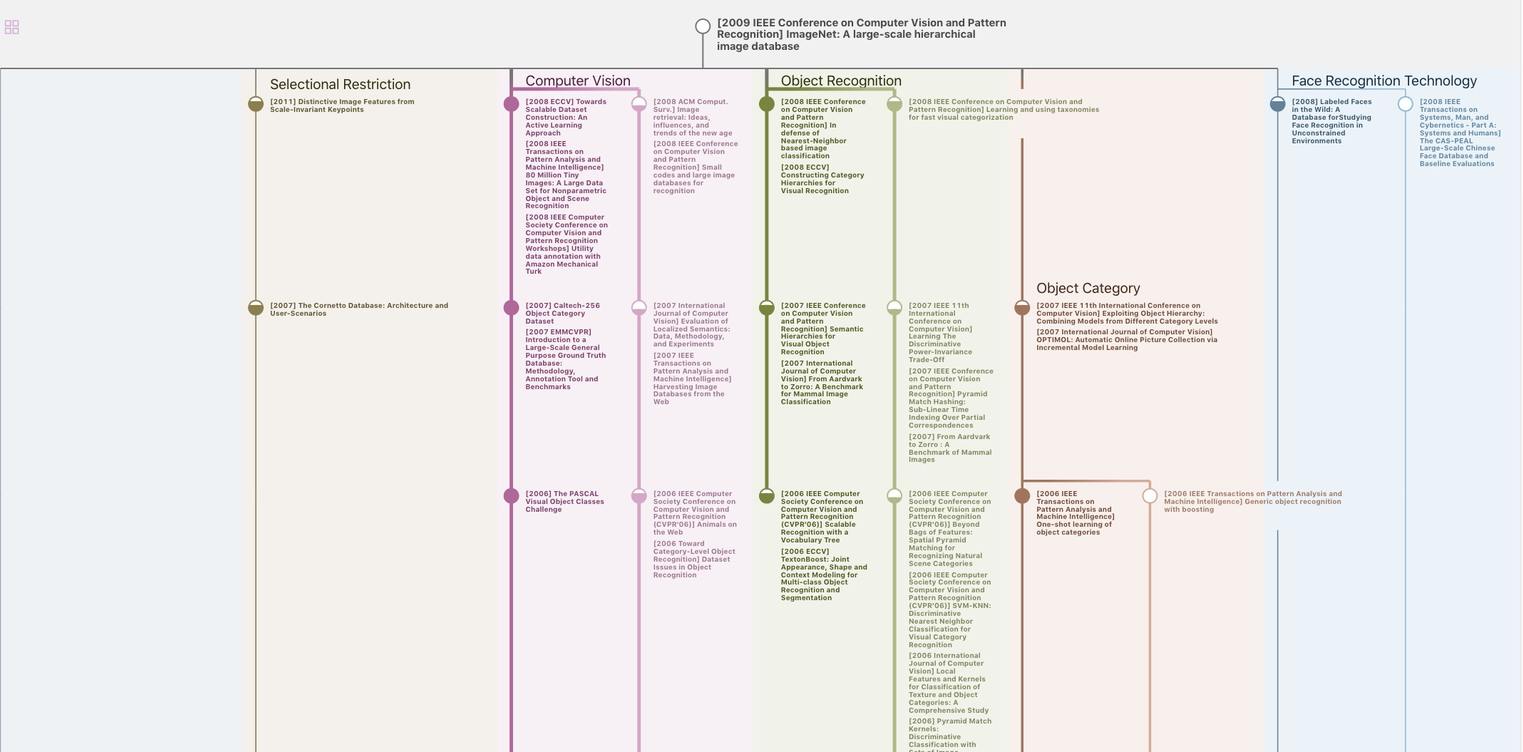
生成溯源树,研究论文发展脉络
Chat Paper
正在生成论文摘要