Limitations and performance of three approaches to Bayesian inference for Gaussian copula regression models of discrete data
Computational Statistics(2021)
摘要
Gaussian copula regression models provide a flexible, intuitive framework in which to model dependent responses with a variety of marginal distributions. With non-continuous outcomes, the time required to compute the likelihood directly grows exponentially with sample size. What alternatives exist rarely have been considered in a Bayesian framework. We conduct inference for Gaussian copula regression models of non-continuous outcomes using three distinct approaches in a Bayesian setting: the continuous extension, the distributional transform, and the composite likelihood. The latter two include curvature correction. We consider the posterior distributional shapes and computational performance as well. We consider both simulations of several types of non-continuous data and analyses of real data. Data sets and types were chosen to challenge the performance of these approaches. Using frequentist methods, we evaluate the inference resulting from these three approaches. The distributional transform with curvature correction has good to excellent coverage for discrete variables with numerous levels. It also offers considerably faster performance than the other options considered, making it attractive for evaluating models of mutually dependent non-continuous responses. For responses with fewer levels, composite likelihood may be the only viable option.
更多查看译文
关键词
Composite likelihood, Continuous extension, Curvature correction, Discrete data, Distributional transform
AI 理解论文
溯源树
样例
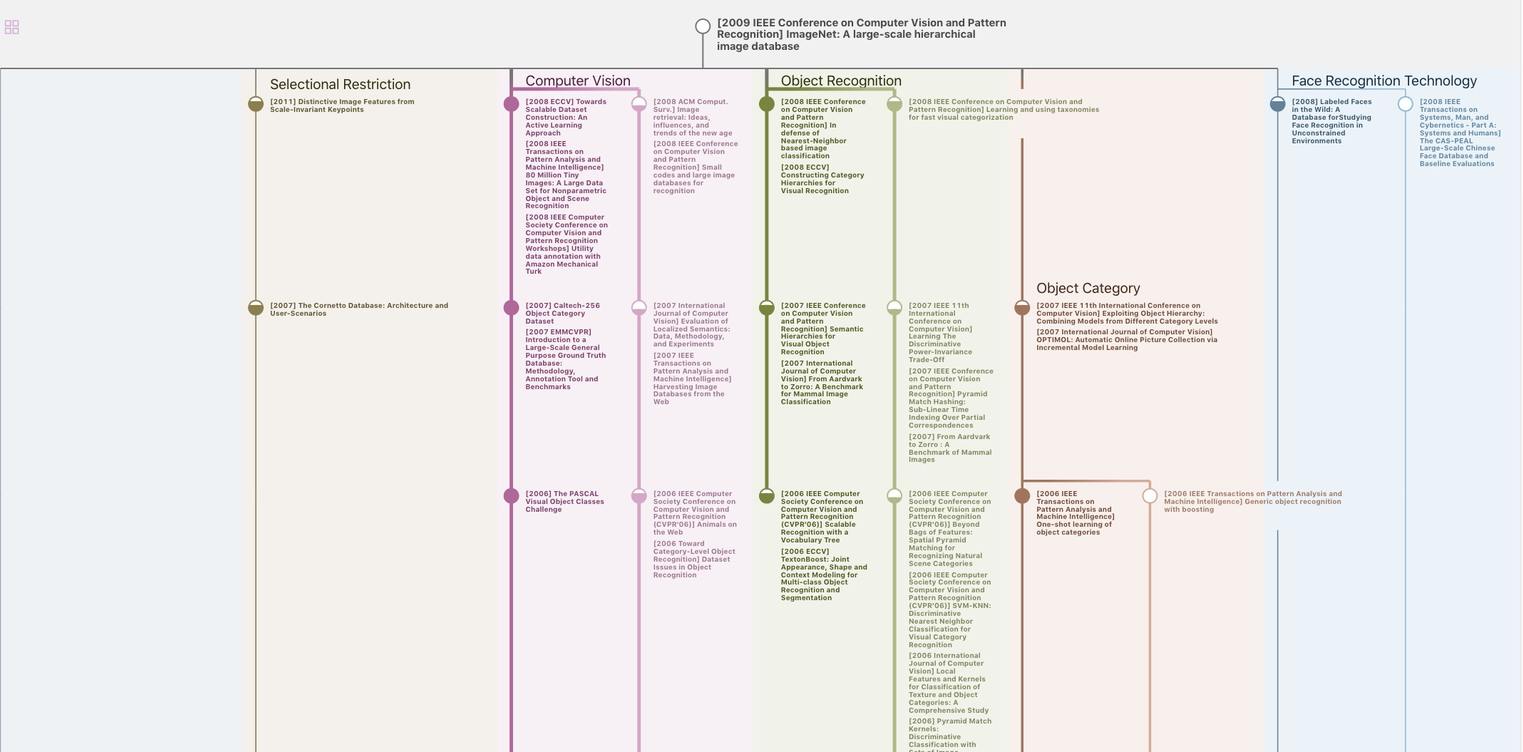
生成溯源树,研究论文发展脉络
Chat Paper
正在生成论文摘要