Rgb Pixel-Block Point-Cloud Fusion For Object Detection
AUTONOMOUS SYSTEMS: SENSORS, PROCESSING, AND SECURITY FOR VEHICLES AND INFRASTRUCTURE 2021(2021)
摘要
This paper explores a multimodal deep learning network based on SqueezeSeg. We extend the standard SqueezeSeg architecture to enable camera and lidar fusion. The sensor processing method is termed pixel-block point-cloud fusion. Using co-registered camera and lidar sensors, the input section of the proposed network creates a feature vector by extracting information from a block of RGB pixels from each point in the point-cloud that is also in the camera's field of view. Essentially, each lidar point is paired with neighboring RGB data so the feature extractor has more meaningful information from the image. This fusion method adds rich information on object color and texture from the camera data to enhance the overall performance. The image pixel blocks will not only add color information to the lidar data, but it will also add information about texture. The proposed pixel-block point-cloud fusion method yields better results than single-pixel fusion.
更多查看译文
关键词
object detection, SqueezeSeg, lidar, camera, sensor fusion, computer vision, deep learning, machine learning
AI 理解论文
溯源树
样例
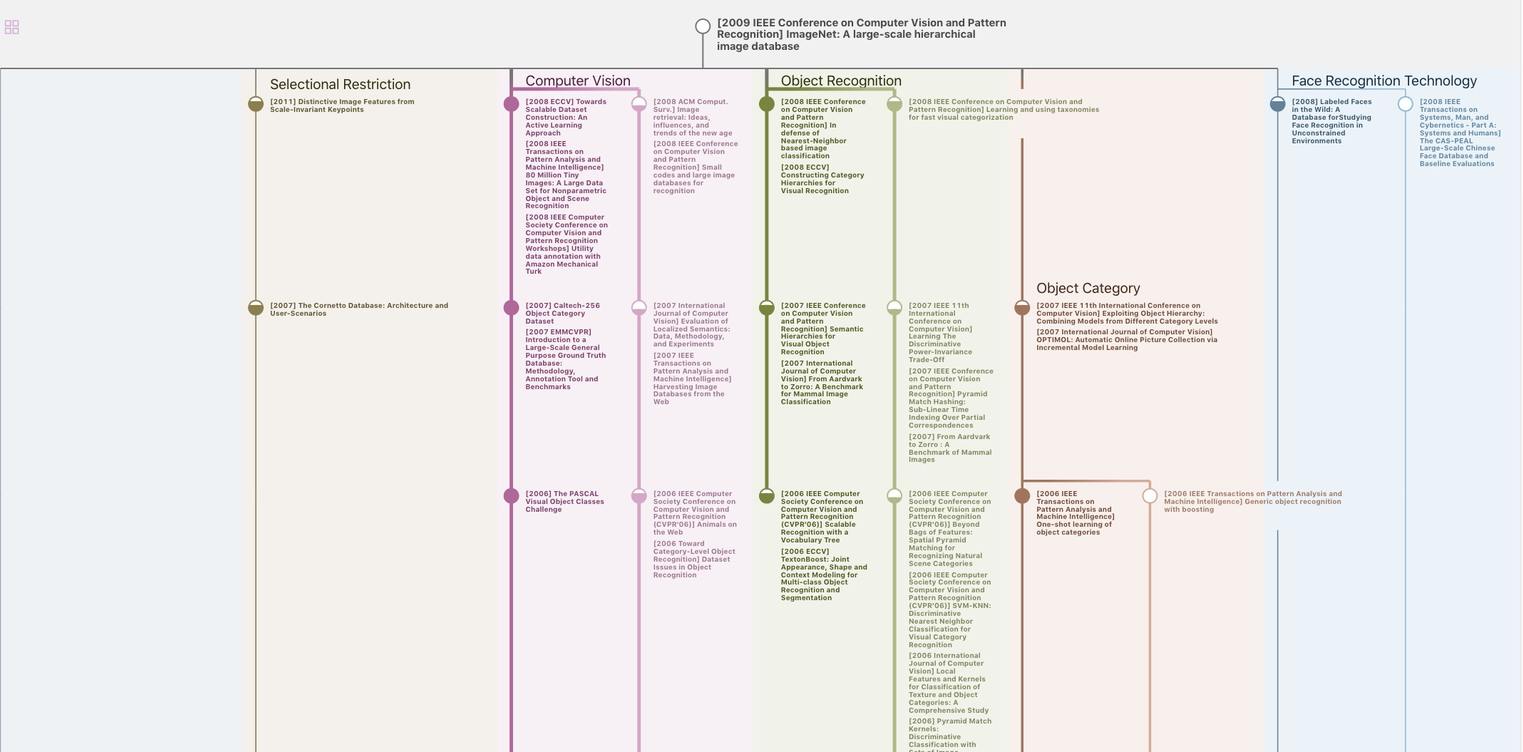
生成溯源树,研究论文发展脉络
Chat Paper
正在生成论文摘要