Finetuned Yolov3 For Getting Four Times The Detection Speed
KNOWLEDGE SCIENCE, ENGINEERING AND MANAGEMENT, PT III(2021)
摘要
Object detection is an exciting research area in computer vision, widely used in autonomous driving, face recognition, and drones. Due to the limitation of mobile devices, the size of object detection models is limited, and it is not easy to achieve the ideal balance between detection accuracy and detection speed. So reducing the model size and increasing the model detection speed becomes a trendy research topic. The Slice-Concat structure proposed in this paper does not require too many changes to YOLOv3 and YOLOv3-SPP, and the four times improvement in detection speed is obtained only by changing the width and height of the input feature map. While getting a 4-fold FPS improvement, the network model parameters remain essentially unchanged, and the computational effort becomes a quarter of that of the original network. The improved YOLO model can also obtain a larger batch size with the same image size, providing an excellent solution for training models on hardware-constrained devices.
更多查看译文
关键词
Object detection, YOLOv3, YOLOv3-SPP, Lightweight, Real-time detection
AI 理解论文
溯源树
样例
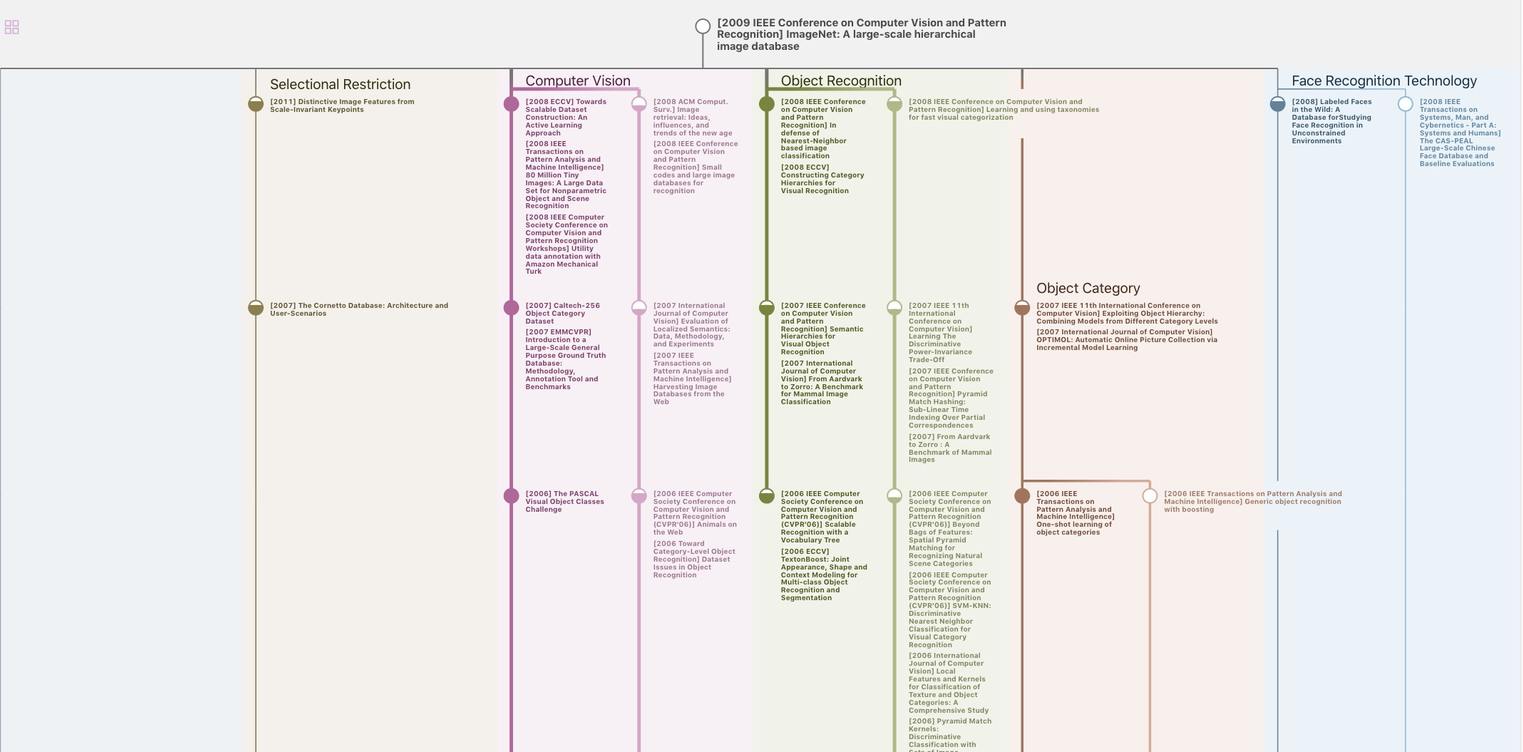
生成溯源树,研究论文发展脉络
Chat Paper
正在生成论文摘要