Representing Knowledge Graphs With Gaussian Mixture Embedding
KNOWLEDGE SCIENCE, ENGINEERING AND MANAGEMENT, PT I(2021)
摘要
Knowledge Graph Embedding (KGE) has attracted more and more attention and has been widely used in downstream AI tasks. Some proposed models learn the embeddings of Knowledge Graph (KG) into a low-dimensional continuous vector space by optimizing a customized loss function. However, these methods either ignore the polysemy of entities/relations in KG, or cannot model the uncertainty of them. Therefore, we propose KG2GM (Knowledge Graph to Gaussian Mixture), a density-based KGE method, for modeling the polysemy and uncertainty of KG simultaneously. Each entity/relation in KG is represented by a Gaussian mixture probability distribution. Each Gaussian component of the mixed distribution represents one kind of semantics, where the mean vector denotes its space position, and the covariance matrix denotes its uncertainty. In this way we can model the polysemy and uncertainty of KG simultaneously. We employ symmetrized KL divergence and EL (Expected Likelihood) to measure the component similarities and make their probabilistic combination as the score function of the triplet. We conduct experiments on link prediction with two benchmark datasets (WN18RR and FB15k-237). The results show that our method can effectively model the polysemy and uncertainty of entities and relations in KG.
更多查看译文
关键词
Knowledge Graph Embedding, Gaussian Mixture Model, Distributed representation, Knowledge graph completion, Link prediction
AI 理解论文
溯源树
样例
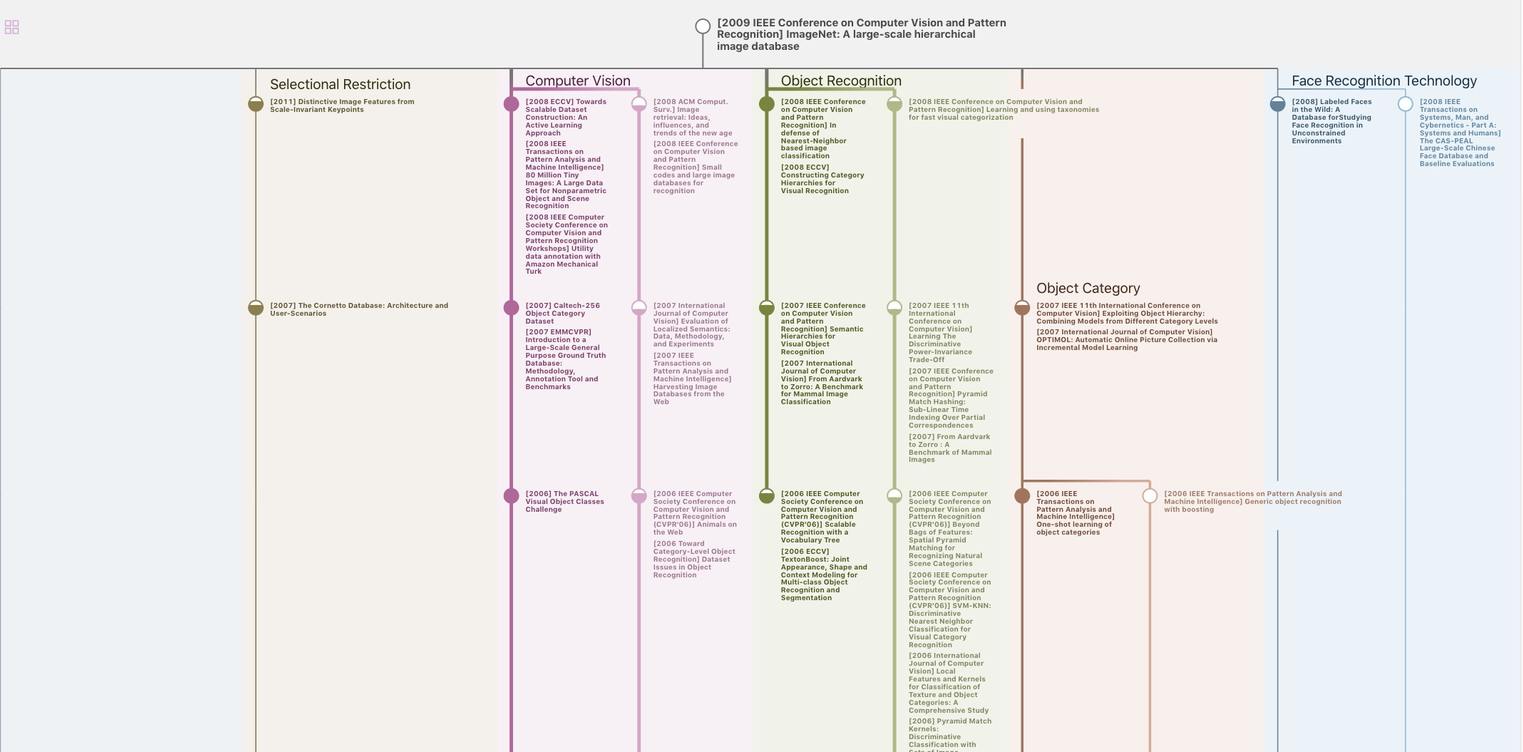
生成溯源树,研究论文发展脉络
Chat Paper
正在生成论文摘要