A New Maximum Entropy Method For Estimation Of Multimodal Probability Density Function
APPLIED MATHEMATICAL MODELLING(2022)
摘要
The high-precision estimation of a multimodal probability density function is a difficult problem in many engineering fields. We propose a new method to improve the estimation accuracy based on the fractional moment-based maximum entropy method with a nonlinear transformation and a multi-peak recognition method. For the translation parameters in the nonlinear transformation, three approaches, such as sample-based least square polynomial fitting technique, sample-based kernel density estimation and classical maximum entropy method, are presented to determine the parameters. By adjusting the translation parameter, the valley of the probability density function curve can be translated to the position with a larger slope, and the distance between adjacent peaks is enlarged to avoid the wrong fitting form of the probability density function curve with multiple peaks. After the parameters of the transformation are obtained, the fractional moment-based maximum entropy method is applied to predict the probability density function of the transformed performance function. Two numerical examples are used to verify the accuracy and stability of the proposed method. Two engineering examples are introduced to illustrate the applicability and efficiency of the proposed method in the real-life engineering setting. It is concluded that the proposed method uses fewer moments with less additional calculation costs, and has good computational efficiency and applicability for the modeling of multi modal probability density functions, compared with the classical fractional moment-based maximum entropy method. (c) 2021 Elsevier Inc. All rights reserved.
更多查看译文
关键词
Maximum entropy method, Fractional moment, Nonlinear transformation, Multimodal probability density estimation
AI 理解论文
溯源树
样例
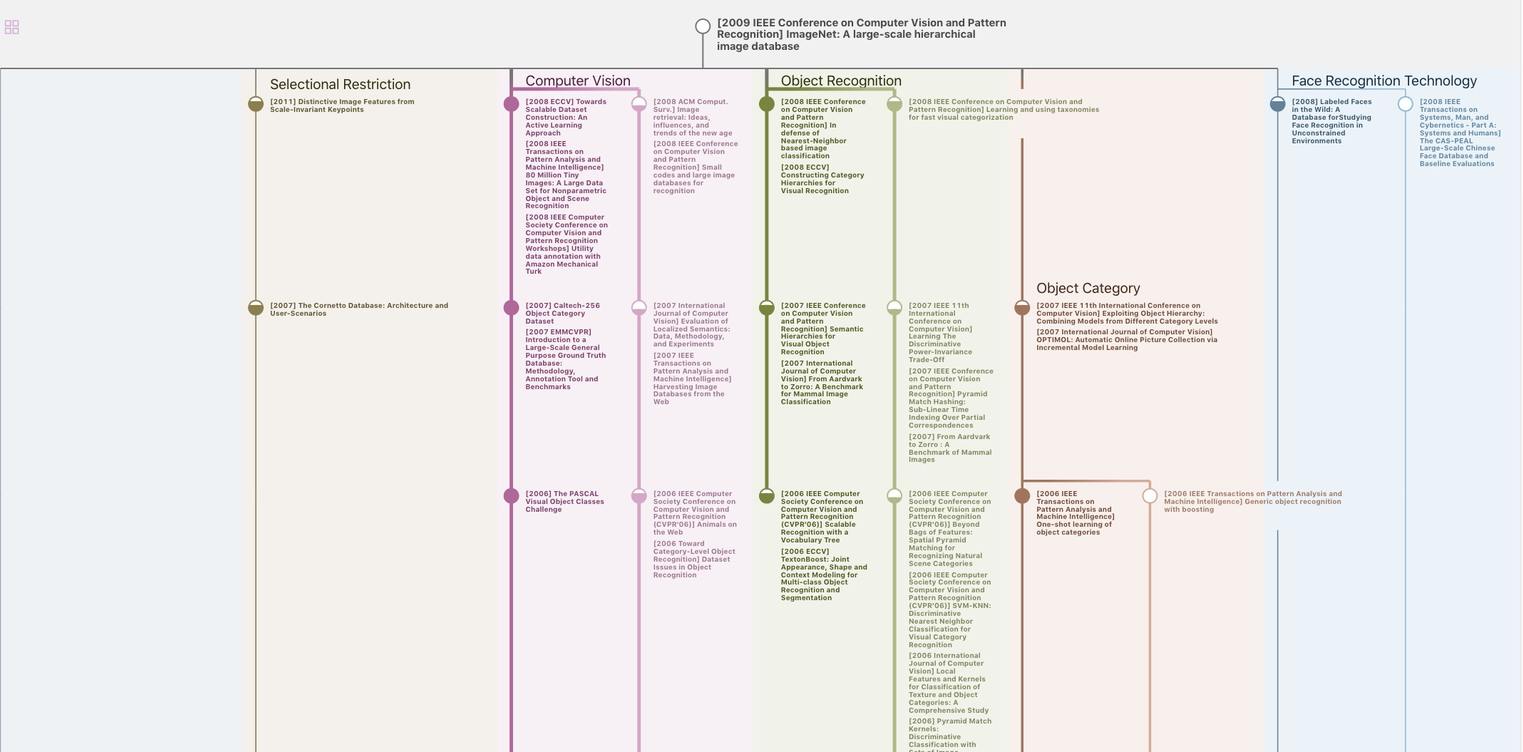
生成溯源树,研究论文发展脉络
Chat Paper
正在生成论文摘要