Analysis Of Perceptual Hashing Algorithms In Image Manipulation Detection
BIG DATA, IOT, AND AI FOR A SMARTER FUTURE(2021)
摘要
Perceptual image hashing is a family of algorithms that generate content-based image hashes. Unlike cryptographic hashes, perceptual hashes are designed to not change much when an image undergoes minor modifications such as compression, color-correction, and brightness. Therefore, these algorithms have been found useful in detecting redundant images, perform reverse image search, and flagging/filtering inappropriate imagery by comparing image hashes with a dataset of known perceptual hashes. While these applications have been served well, there is a new threat to visual information in the form of misinformation through content changing image manipulation using techniques such as object addition/removal and copy-move. While perceptual hashing algorithms can very accurately identify copies of similar images and their legitimately modified versions, their effectiveness in detecting content changing manipulations is not studied well. Existing research and advanced image libraries propose various image hashing algorithms, and several datasets of manipulated images are available in the public domain. In this work, a review and performance analysis of several perceptual hashing algorithms against two such datasets is presented. Results show less than ideal performance in distinguishing maliciously manipulated images from legitimate ones. Based on the results, limitations of perceptual hashing algorithms are identified, and future research directions are proposed. (c) 2021 The Authors. Published by Elsevier B.V. This is an open access article under the CC BY-NC-ND license (https://creativecommons.org/licenses/by-nc-nd/4.0) Peer-review under responsibility of the scientific committee of the Complex Adaptive Systems Conference, June 2021.
更多查看译文
关键词
Perceptual hashing, Image manipulation detection
AI 理解论文
溯源树
样例
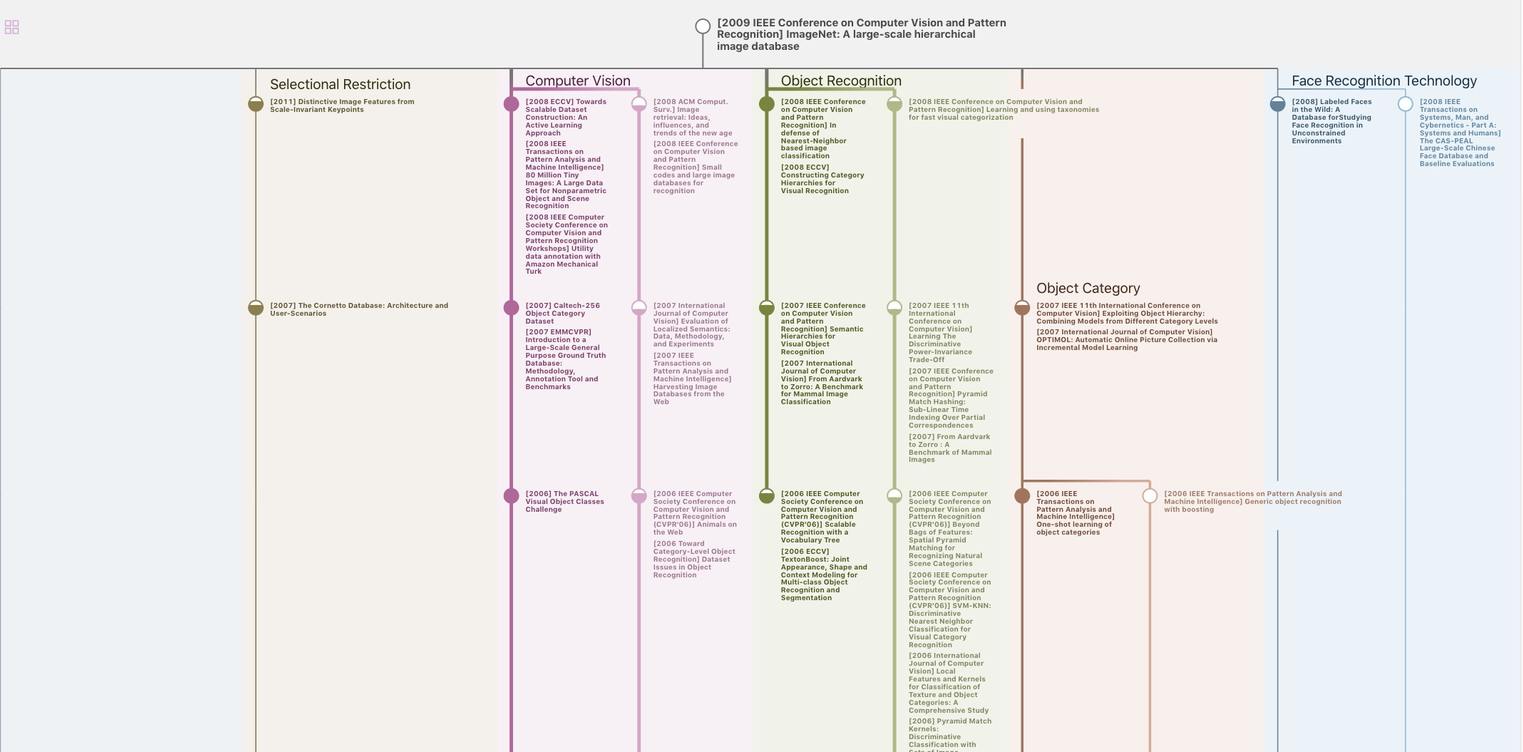
生成溯源树,研究论文发展脉络
Chat Paper
正在生成论文摘要