Stock Price Manipulation Detection Using Deep Unsupervised Learning: The Case Of Thailand
IEEE ACCESS(2021)
摘要
Detecting stock price manipulation is a cat-and-mouse game. Manipulators have constantly devised new techniques to avoid detection. The majority of the related work employed supervised learning techniques, which necessitated known manipulation patterns as examples for their models to recognize. To catch unknown and never-before-seen manipulation, we used unsupervised learning to train deep neural networks for detecting stock price manipulation in order to detect unknown and previously unseen manipulation. The models were trained to recognize normal trading behaviors that were expressed in a limit order book. Anomaly trading actions that did not follow to the learned patterns were identified as manipulated. The strength of our method is that it does not require prior knowledge about the characteristics of manipulation. As a result, it is best suited for detecting new or unknown types of manipulation. Two model architectures were evaluated: autoencoder (AE) and generative adversarial networks (GANs). They were put to the test on six prosecuted real manipulation cases from the Stock Exchange of Thailand (SET). With a low false-positive rate, both models could identify five out of six cases. For practical application of the models, a strategy called "MinManiMax" was also proposed to optimize the decision boundary.
更多查看译文
关键词
Stock markets, Regulators, Unsupervised learning, Manipulators, Security, Machine learning algorithms, Companies, Anomaly detection, market abuse, stock markets, stock price manipulation, unsupervised learning
AI 理解论文
溯源树
样例
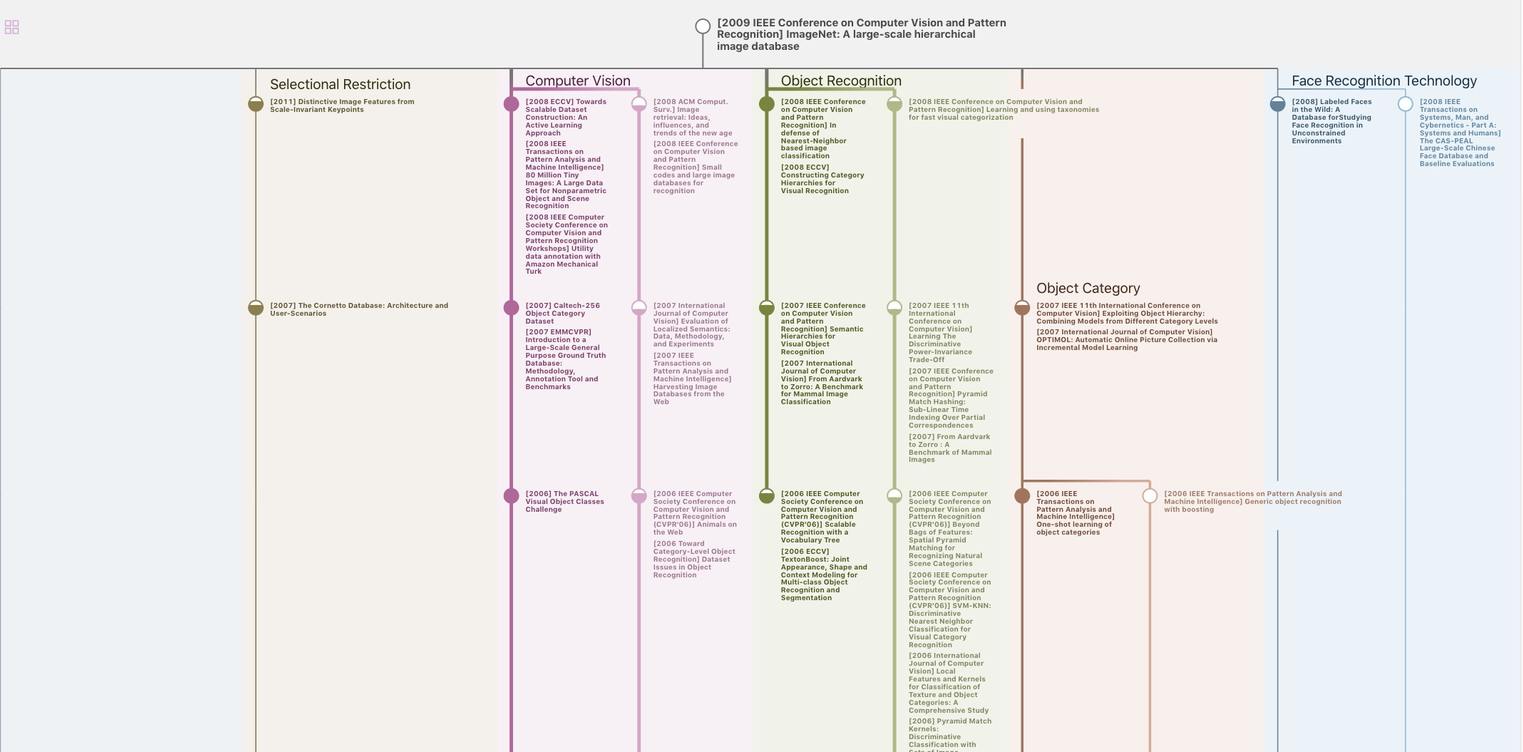
生成溯源树,研究论文发展脉络
Chat Paper
正在生成论文摘要