Bootstrapping Multivariate Portmanteau Tests For Vector Autoregressive Models With Weak Assumptions On Errors
COMPUTATIONAL STATISTICS & DATA ANALYSIS(2022)
Abstract
This article discusses diagnostic checking for vector autoregressive models with uncorrelated but not independent innovations. In this situation, the multivariate portmanteau tests are severely over-sized due to the misspecification of critical values obtained from the Chi(2) distribution. To address this issue, a random weighting bootstrap procedure is proposed to approximate the null distribution when the error is assumed to be martingale difference sequence. When this assumption is violated, a blockwise random weighting is further applied to replicate the dependence structure of innovations. The first-order asymptotic validity of these bootstrap procedures is derived. Monte Carlo experiments under various scenarios suggest the effectiveness of the random weighting bootstrap approaches in comparison with existing approaches. Finally, the proposed testing procedure is illustrated in an application to analyze feedback dynamics between the real GNP growth and the unemployment rate in the US. (C) 2021 Elsevier B.V. All rights reserved.
MoreTranslated text
Key words
Weak VAR model, Multivariate white noise checking, Goodness-of-fit test, Random weighting bootstrap
AI Read Science
Must-Reading Tree
Example
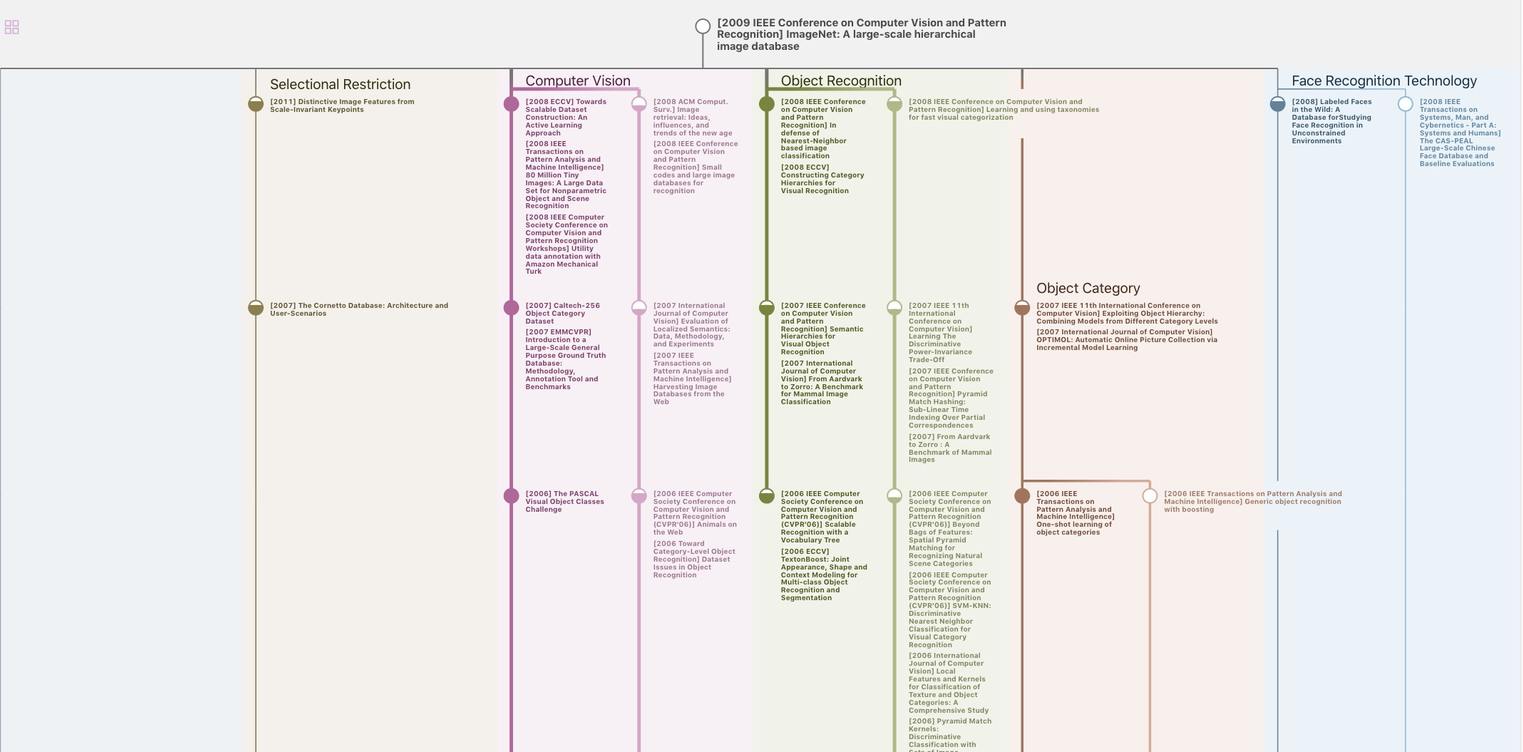
Generate MRT to find the research sequence of this paper
Chat Paper
Summary is being generated by the instructions you defined