Estimation of Water Stress in Potato Plants Using Hyperspectral Imagery and Machine Learning Algorithms
HORTICULTURAE(2021)
Abstract
This work presents quantitative detection of water stress and estimation of the water stress level: none, light, moderate, and severe on potato crops. We use hyperspectral imagery and state of the art machine learning algorithms: random decision forest, multilayer perceptron, convolutional neural networks, support vector machines, extreme gradient boost, and AdaBoost. The detection and estimation of water stress in potato crops is carried out on two different phenological stages of the plants: tubers differentiation and maximum tuberization. The machine learning algorithms are trained with a small subset of each hyperspectral image corresponding to the plant canopy. The results are improved using majority voting to classify all the canopy pixels in the hyperspectral images. The results indicate that both detection of water stress and estimation of the level of water stress can be obtained with good accuracy, improved further by majority voting. The importance of each band of the hyperspectral images in the classification of the images is assessed by random forest and extreme gradient boost, which are the machine learning algorithms that perform best overall on both phenological stages and detection and estimation of water stress in potato crops.
MoreTranslated text
Key words
water stress,potato,hyperspectral image,machine learning,band importance
AI Read Science
Must-Reading Tree
Example
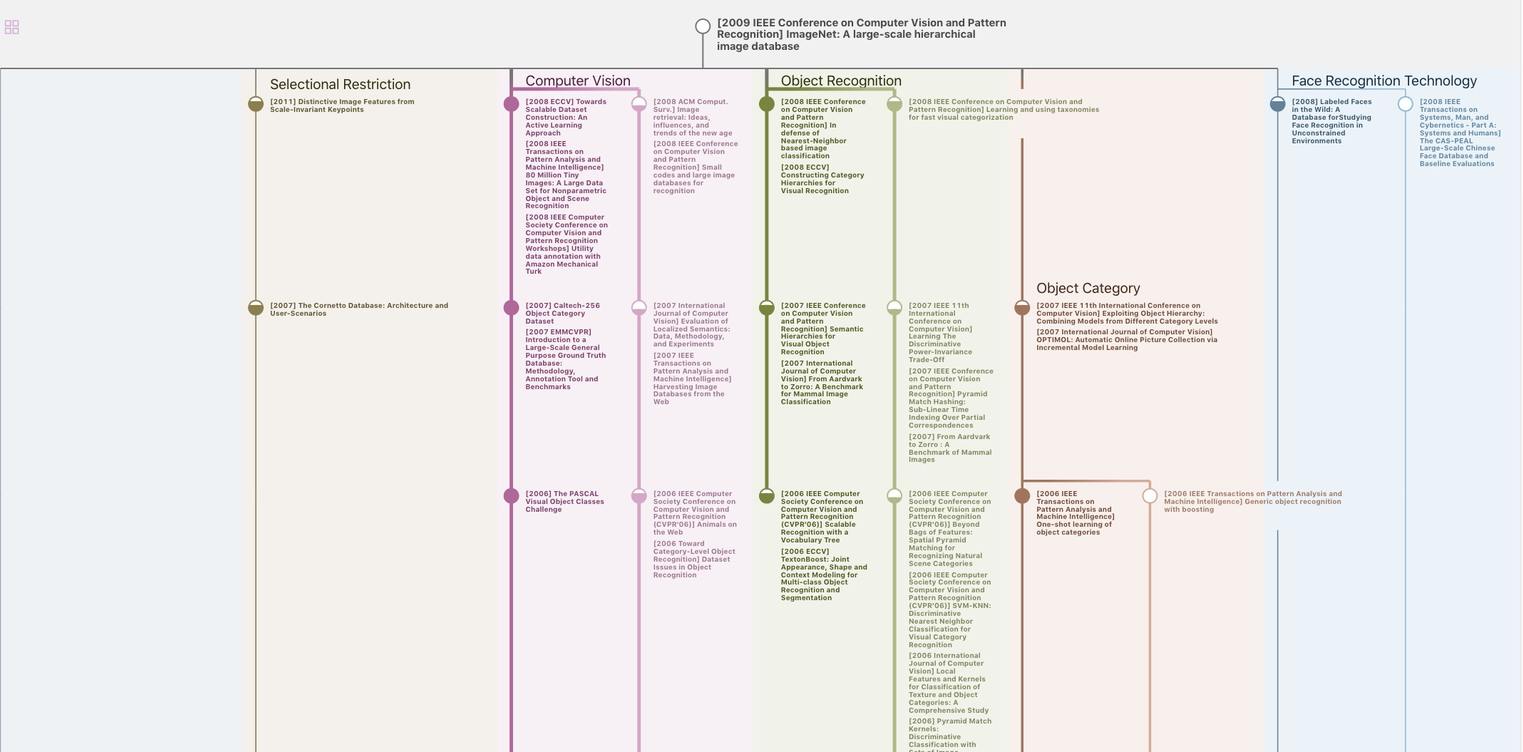
Generate MRT to find the research sequence of this paper
Chat Paper
Summary is being generated by the instructions you defined