Effective Feature Selection Based on Fisher Ratio for Snoring Recognition Using Different Validation Methods
Applied Acoustics(2021)
关键词
Snoring recognition,Feature selection,Fisher Ratio,Acoustic feature,XGBoost
AI 理解论文
溯源树
样例
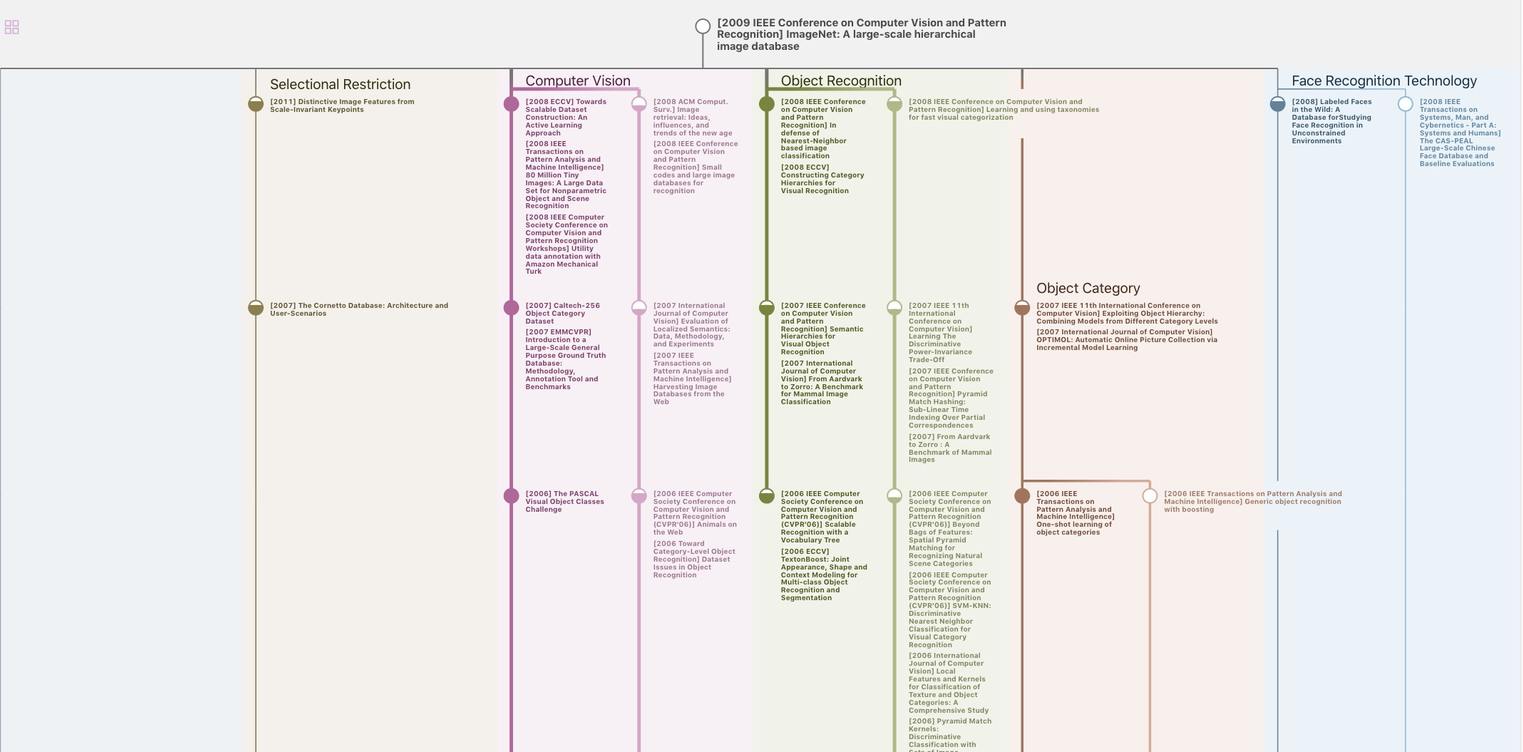
生成溯源树,研究论文发展脉络
Chat Paper
正在生成论文摘要