Well logging prediction and uncertainty analysis based on recurrent neural network with attention mechanism and Bayesian theory
Journal of Petroleum Science and Engineering(2022)
摘要
Deep learning technology can fit the nonlinear relations between different logging sequences. It solves the prediction problems that cannot be effectively disposed by traditional physical or empirical models. However, these deep learning models lack uncertainty analysis, which affects the popularity of logging prediction models in the petroleum engineering application. In this study, we investigate the nonlinear well logging prediction and uncertainty analysis methods based on recurrent neural network (RNN) with attention mechanism and Bayesian theory. A codec structure model based on gated recurrent unit (GRU) neural network and attention mechanism is established for data prediction. Integrating Bayesian theory into GRU neural network, a GRU Bayesian framework is presented to capture model uncertainty and data uncertainty. The importance of different logging sequences to the predicted goal at the same depth and a certain depth range is considered by using the attention mechanism, which improves the prediction accuracy and reduces the uncertainty of the prediction. Compressional waves sonic log (DTC) data of carbonate reservoir is predicted by using the existing logging attributes (density, spontaneous potential, natural gamma ray and deep investigate double lateral resistivity). Compared with the traditional RNN models, the accuracy of the constructed model has increased by 7.95% (R2 = 93.07%) and the error decreased by 1.7166% (RMSE = 2.9261%) in the field data. More importantly, the proposed method can quantitatively analyze the uncertainties of the predicted results, which effectively heightens the application of the logging prediction model in the petroleum engineering field.
更多查看译文
关键词
GRU neural Network,Attention mechanism,Bayesian theory,Uncertainty analysis,Well logging prediction
AI 理解论文
溯源树
样例
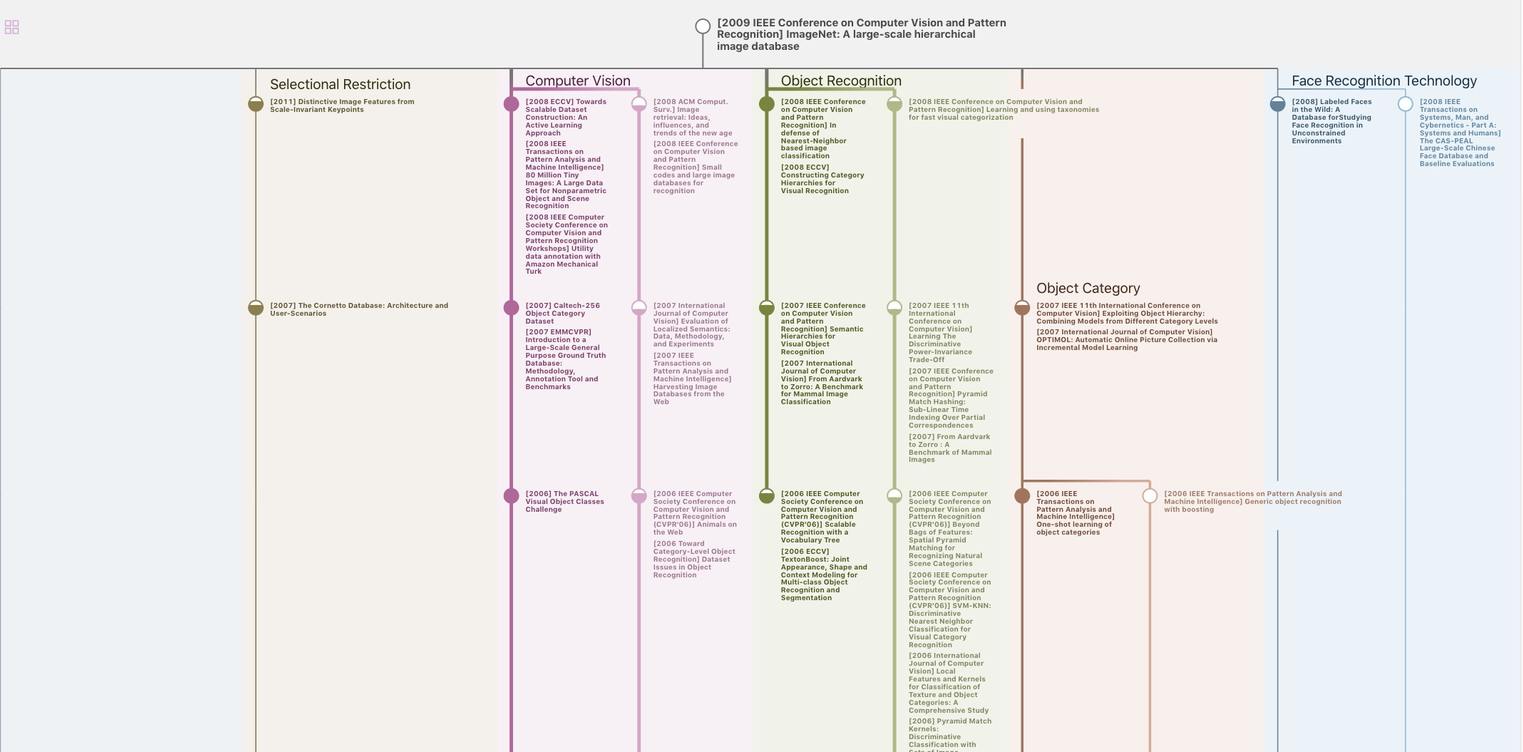
生成溯源树,研究论文发展脉络
Chat Paper
正在生成论文摘要