Deep reinforcement learning in production systems: a systematic literature review
INTERNATIONAL JOURNAL OF PRODUCTION RESEARCH(2022)
摘要
Shortening product development cycles and fully customisable products pose major challenges for production systems. These not only have to cope with an increased product diversity but also enable high throughputs and provide a high adaptability and robustness to process variations and unforeseen incidents. To overcome these challenges, deep Reinforcement Learning (RL) has been increasingly applied for the optimisation of production systems. Unlike other machine learning methods, deep RL operates on recently collected sensor-data in direct interaction with its environment and enables real-time responses to system changes. Although deep RL is already being deployed in production systems, a systematic review of the results has not yet been established. The main contribution of this paper is to provide researchers and practitioners an overview of applications and to motivate further implementations and research of deep RL supported production systems. Findings reveal that deep RL is applied in a variety of production domains, contributing to data-driven and flexible processes. In most applications, conventional methods were outperformed and implementation efforts or dependence on human experience were reduced. Nevertheless, future research must focus more on transferring the findings to real-world systems to analyse safety aspects and demonstrate reliability under prevailing conditions.
更多查看译文
关键词
Machine learning, reinforcement learning, production control, production planning, manufacturing processes, systematic literature review
AI 理解论文
溯源树
样例
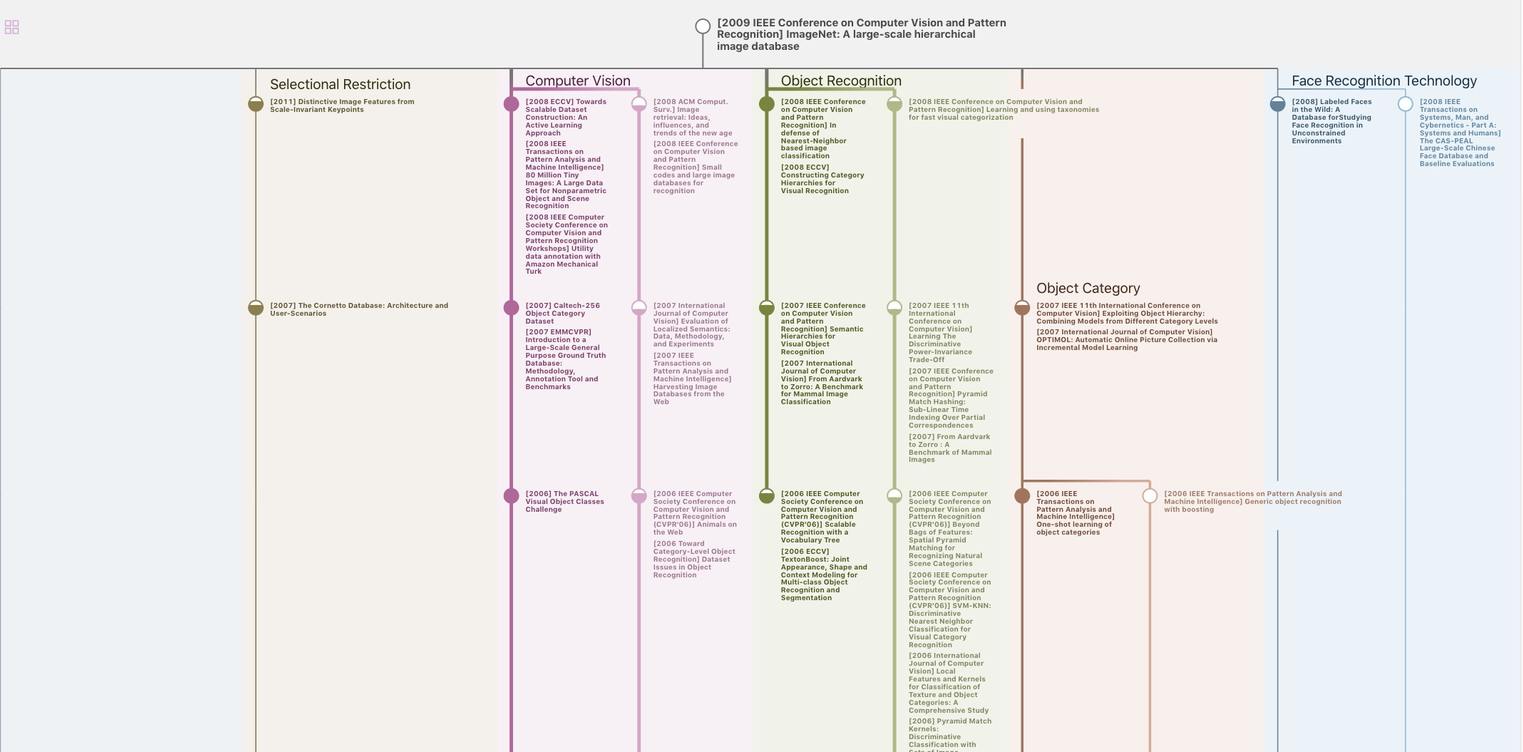
生成溯源树,研究论文发展脉络
Chat Paper
正在生成论文摘要