Estimation Of Soil Texture Using Sentinel-2 Multispectral Imaging Data: An Ensemble Modeling Approach
SOIL & TILLAGE RESEARCH(2021)
Abstract
Soil texture is an important compositional property where the separate estimates of sand, silt, and clay often does not add up to 100 % when predicted independently using digital soil mapping approach. An alternative is to use Remote sensing (RS) data, however, varying soil surface conditions, satellite pass day, and cloud cover on imagery often limit its estimation accuracies. Here, we examined an ensemble modeling approach to improve the estimation accuracy of soil textural fractions using Sentinel-2 (S2) data. Multispectral images (cloud free) from S2 mission for 63 different acquisition dates (between Jan. 2017 to Dec. 2020) were used as the RS data source. Spectral reflectance data over visible to near-infrared region (350-2500 nm at 1 nm interval) were also collected for each soil sample under laboratory conditions for comparison. Partial-least-square regression (PLSR), random forest (RF) and support vector regression (SVR) models were used to estimate sand, silt, and clay contents for both the laboratory-measured and S2 spectra. Resulting coefficient of determination (R-2) and root-mean-squared error (RMSE) values for the laboratory-measured spectra were 0.44 and 14.9 % for sand contents, 0.32 and 16.23 % for silt contents, and 0.69 and 5.5 % for clay contents, respectively. The S2 datasets collected during Apr. 13, 2019 and Nov. 24, 2019 yielded best performance statistics for sand (R-2 = 0.50, RMSE = 11.6 %) and silt (R-2 = 0.46, RMSE = 5.8 %) contents, respectively. Similarly, the dataset collected on Jan. 3, 2018 yielded best performance for the clay contents (R-2 = 0.44, RMSE = 6.9 %). Compared to S2 data of a single acquisition date, combination of S2 data from multiple acquisition dates through ensemble modeling could significantly improve the prediction accuracies for sand (R-2 = 0.62, RMSE = 9.9 %), silt (R-2 = 0.54, RMSE = 5.4 %), and clay contents (R-2 = 0.54, RMSE = 6.5 %). These developed models allowed for the estimation of textural fractions at a resolution of 30 m.
MoreTranslated text
Key words
Sentinel 2 data, Soil texture, Ensemble approach
AI Read Science
Must-Reading Tree
Example
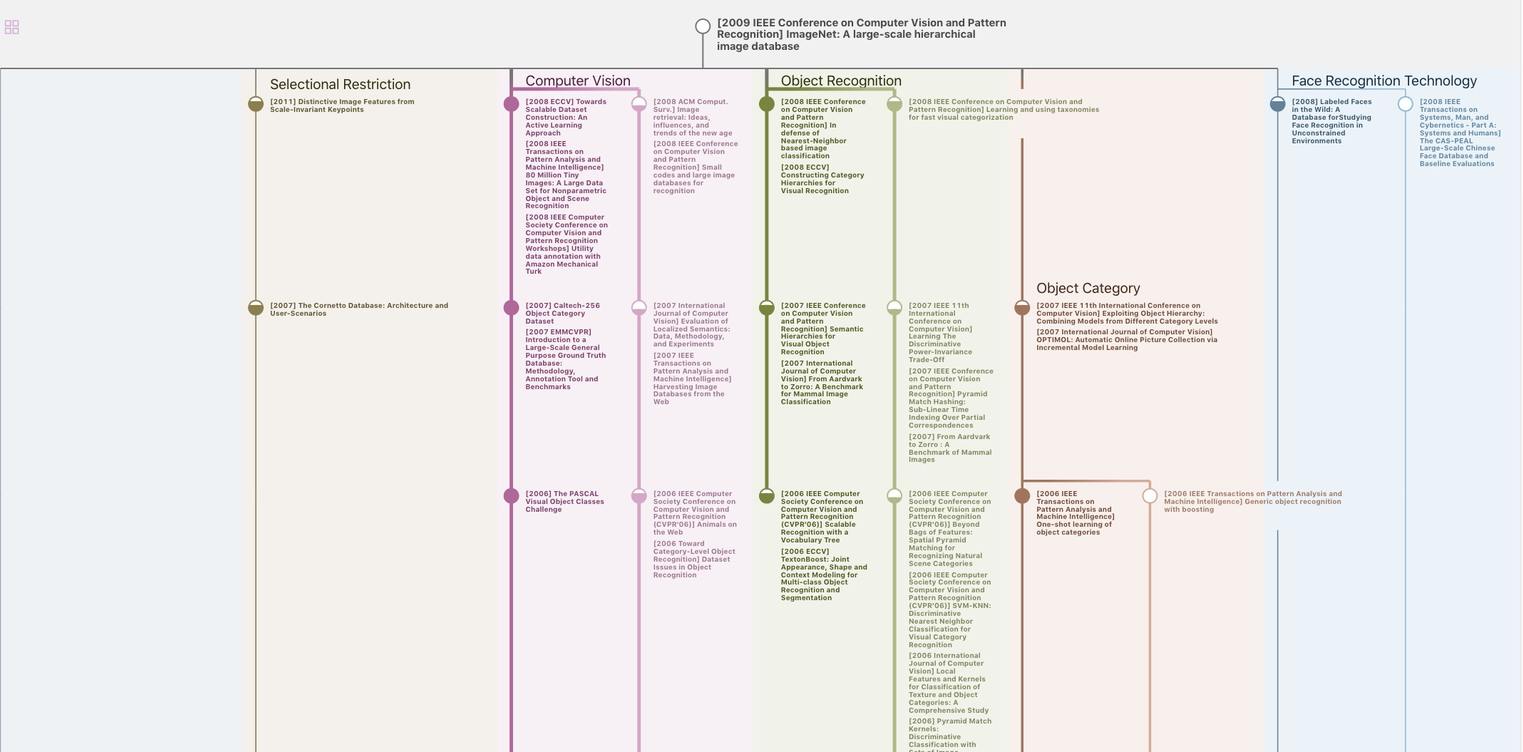
Generate MRT to find the research sequence of this paper
Chat Paper
Summary is being generated by the instructions you defined