Detecting The Absence Of Lung Sliding In Lung Ultrasounds Using Deep Learning
APPLIED SCIENCES-BASEL(2021)
摘要
Certain post-thoracic surgery complications are monitored in a standard manner using methods that employ ionising radiation. A need to automatise the diagnostic procedure has now arisen following the clinical trial of a novel lung ultrasound examination procedure that can replace X-rays. Deep learning was used as a powerful tool for lung ultrasound analysis. We present a novel deep-learning method, automated M-mode classification, to detect the absence of lung sliding motion in lung ultrasound. Automated M-mode classification leverages semantic segmentation to select 2D slices across the temporal dimension of the video recording. These 2D slices are the input for a convolutional neural network, and the output of the neural network indicates the presence or absence of lung sliding in the given time slot. We aggregate the partial predictions over the entire video recording to determine whether the subject has developed post-surgery complications. With a 64-frame version of this architecture, we detected lung sliding on average with a balanced accuracy of 89%, sensitivity of 82%, and specificity of 92%. Automated M-mode classification is suitable for lung sliding detection from clinical lung ultrasound videos. Furthermore, in lung ultrasound videos, we recommend using time windows between 0.53 and 2.13 s for the classification of lung sliding motion followed by aggregation.
更多查看译文
关键词
deep learning, convolutional neural network, lung ultrasound, lung sliding, pneumothorax
AI 理解论文
溯源树
样例
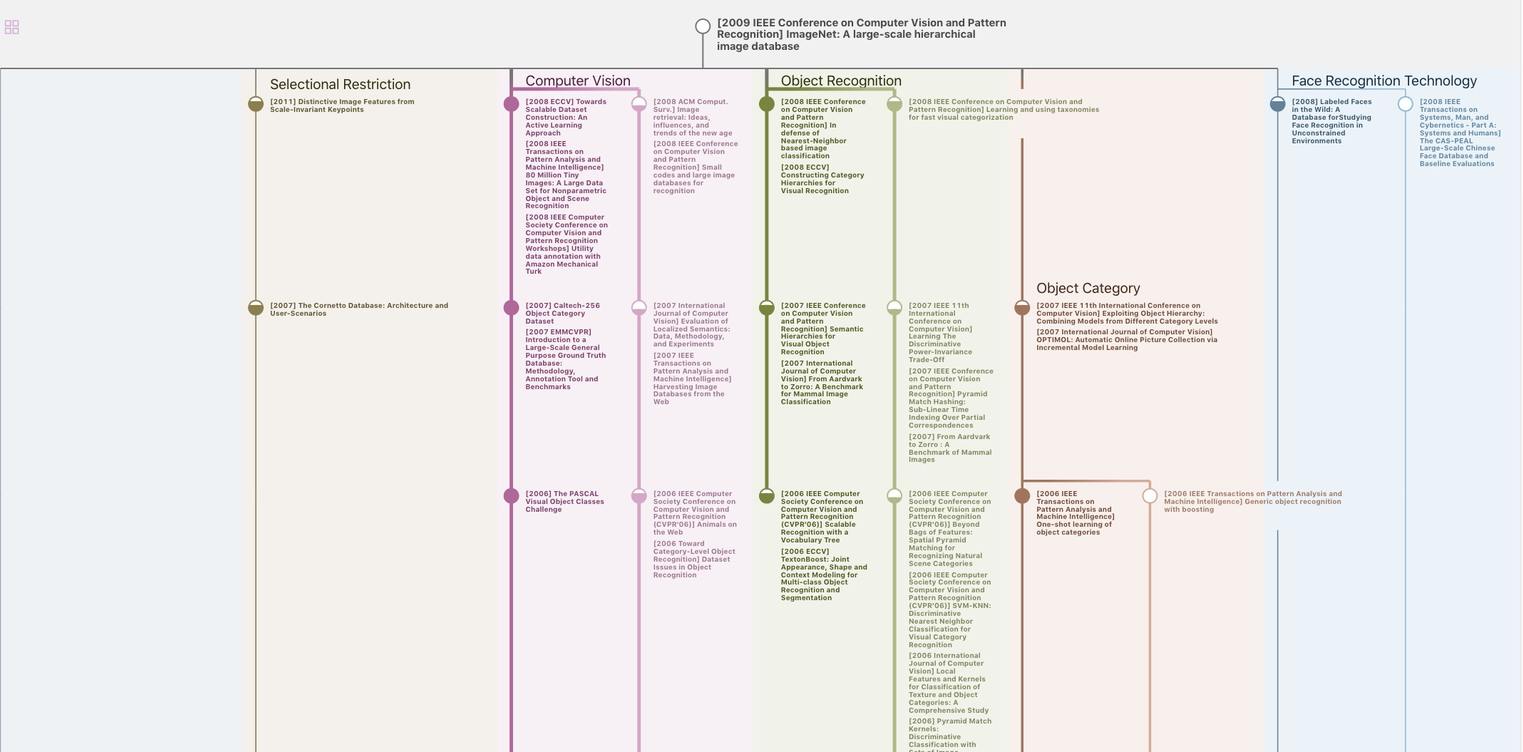
生成溯源树,研究论文发展脉络
Chat Paper
正在生成论文摘要