Alfo: Adaptive Light Field Over-Segmentation
IEEE ACCESS(2021)
摘要
Automatic image over-segmentation into superpixels has attracted increasing attention from researchers to apply it as a pre-processing step for several computer vision applications. In 4D Light Field (LF) imaging, image over-segmentation aims at achieving not only superpixel compactness and accuracy but also cross-viewconsistency. Due to the high dimensionality of 4D LF images, depth information can be estimated and exploited during the over-segmentation along with spatial and visual appearance features. However, balancing between several hybrid features to generate robust superpixels for different 4D LF images is challenging and not adequately solved in existing solutions. In this paper, an automatic, adaptive, and view-consistent LF over-segmentation method based on normalized LF cues and K-means clustering is proposed. Initially, disparity maps for all LF views are estimated entirely to improve superpixel accuracy and consistency. Afterwards, by using K-means clustering, a 4D LF image is iteratively divided into regular superpixels that adhere to object boundaries and ensure cross-view consistency. Our proposed method can automatically adjust the clustering weights of the various features that characterize each superpixel based on the image content. Quantitative and qualitative results on several 4D LF datasets demonstrate outperforming performance of the proposed method in terms of superpixel accuracy, shape regularity and view consistency when using adaptive clustering weights, compared to the state-of-the-art 4D LF over-segmentation methods.
更多查看译文
关键词
Automatic segmentation, adaptive light field over-segmentation, superpixels
AI 理解论文
溯源树
样例
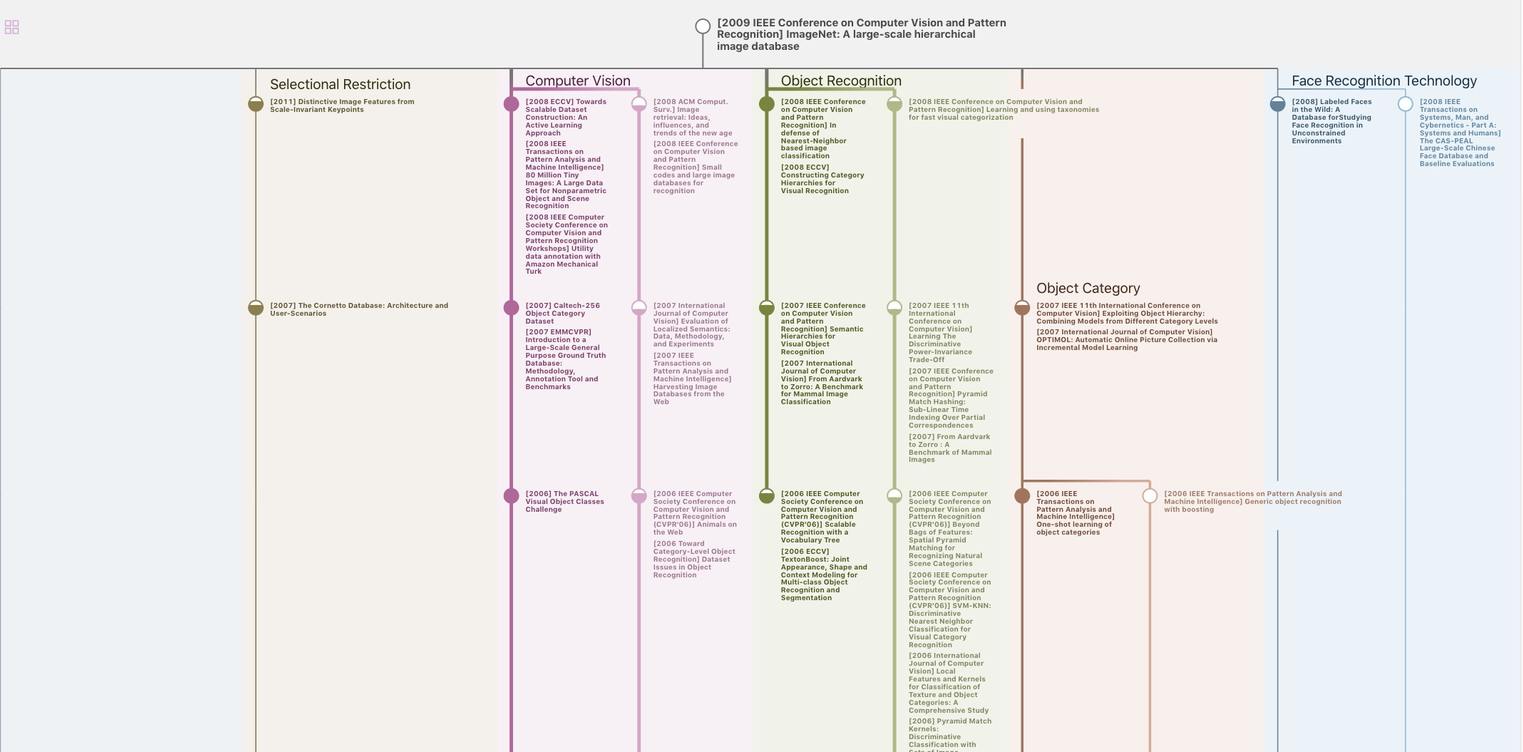
生成溯源树,研究论文发展脉络
Chat Paper
正在生成论文摘要