Sparsity-Promoting Least-Squares Reverse Time Migration Via Preconditioned Bregmanized Operator Splitting
GEOPHYSICAL PROSPECTING(2021)
摘要
Nowadays the least-squares reverse time migration has become the most used migration method because of its accuracy in amplitude recovery and high-resolution imaging, specifically its priority to image the beneath of structural domes such as salt domes. However, errors in the migration velocity model, inadequate physics of modelling/migration and too sparse data decrease the quality of the migrated image. Sparsity constraints help to mitigate the shortcomings of the least-squares reverse time migration and stabilize the migration image, but the computational burden of sparse solvers is still a big challenge. In this paper, we propose a fast sparsity-promoting least-squares reverse time migration algorithm based on the Bregmanized operator splitting algorithm. In particular, we solve the least-squares reverse time migration with l(1)-norm regularization to increase the image resolution while removing the artefacts which cannot be suppressed by the traditional least-squares reverse time migration. Also we develop a preconditioned Bregmanized operator splitting algorithm where iteratively using of a preconditioner decreases the computational burden. The proposed method is applied to a few sets of synthetic data, and results are compared with reverse time migration or least-squares reverse time migration to verify its superiority. Numerical tests demonstrate that the proposed preconditioned Bregmanized operator splitting algorithm converges to the desired migration image in a small number of iterations.
更多查看译文
关键词
Constrained regularization, Bregmanized operator splitting, Least-squares reverse time migration, l(1) norm, Preconditioner
AI 理解论文
溯源树
样例
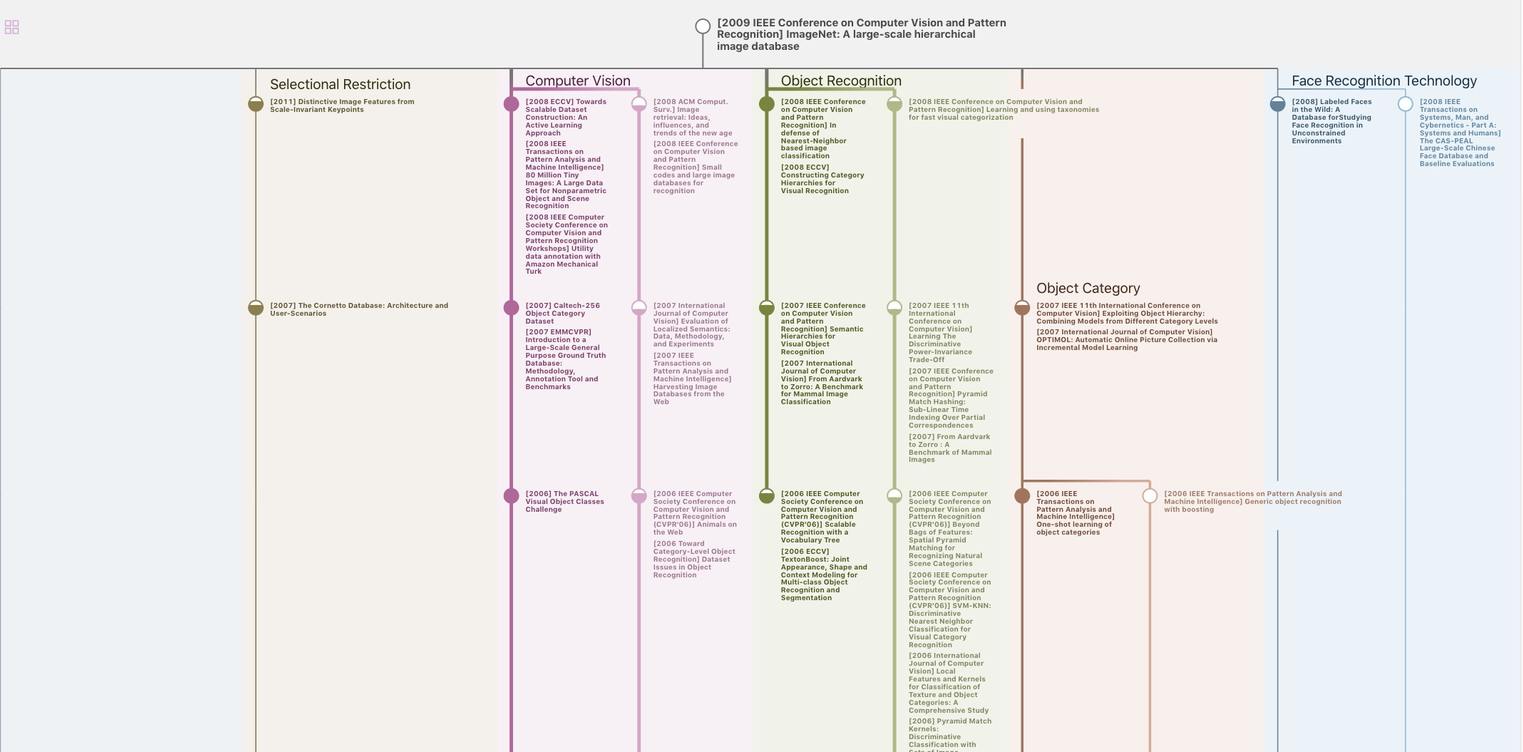
生成溯源树,研究论文发展脉络
Chat Paper
正在生成论文摘要