Ensemble Clustering-Based Fault Diagnosis Method Incorporating Traditional And Deep Representation Features
MEASUREMENT SCIENCE AND TECHNOLOGY(2021)
摘要
The traditional and deep representation features have been successfully employed in the clustering-based fault diagnosis, however, current studies have ignored their heterogeneity and complementarity. Besides, these studies have rarely explored the superiority of ensemble clustering, leading to unstable diagnostic results. To address these issues, a novel ensemble clustering-based fault diagnosis method, namely ECFD, is proposed based on the collaboration of traditional and deep representation features. To the best of our knowledge, this is the first attempt to develop an ensemble clustering method with these types of features for fault diagnosis of rotating machinery. Initially, features are extracted by different approaches, among which the traditional features are obtained by time-domain, frequency-domain, time-frequency-domain approaches, and the deep representation features are extracted by the gated recurrent units-based autoencoders. Then, the heterogeneous and complementary information among these features is explored by the adaptive group regularization method, which is also developed to generate high-quality feature subspaces for constructing the base learners of the ensemble clustering. Finally, the clustering results of each base learner are integrated by a weighted voting method. The effectiveness of the proposed ECFD was verified by the experiments on the real-world bearing vibration dataset. The results indicate that the proposed ECFD can explore the heterogeneity and complementarity among different kinds of features and take advantage of the ensemble clustering, which is superior to other existing benchmark clustering methods in unsupervised fault diagnosis.
更多查看译文
关键词
fault diagnosis, autoencoder, ensemble clustering, unsupervised feature selection, group sparsity, feature fusion
AI 理解论文
溯源树
样例
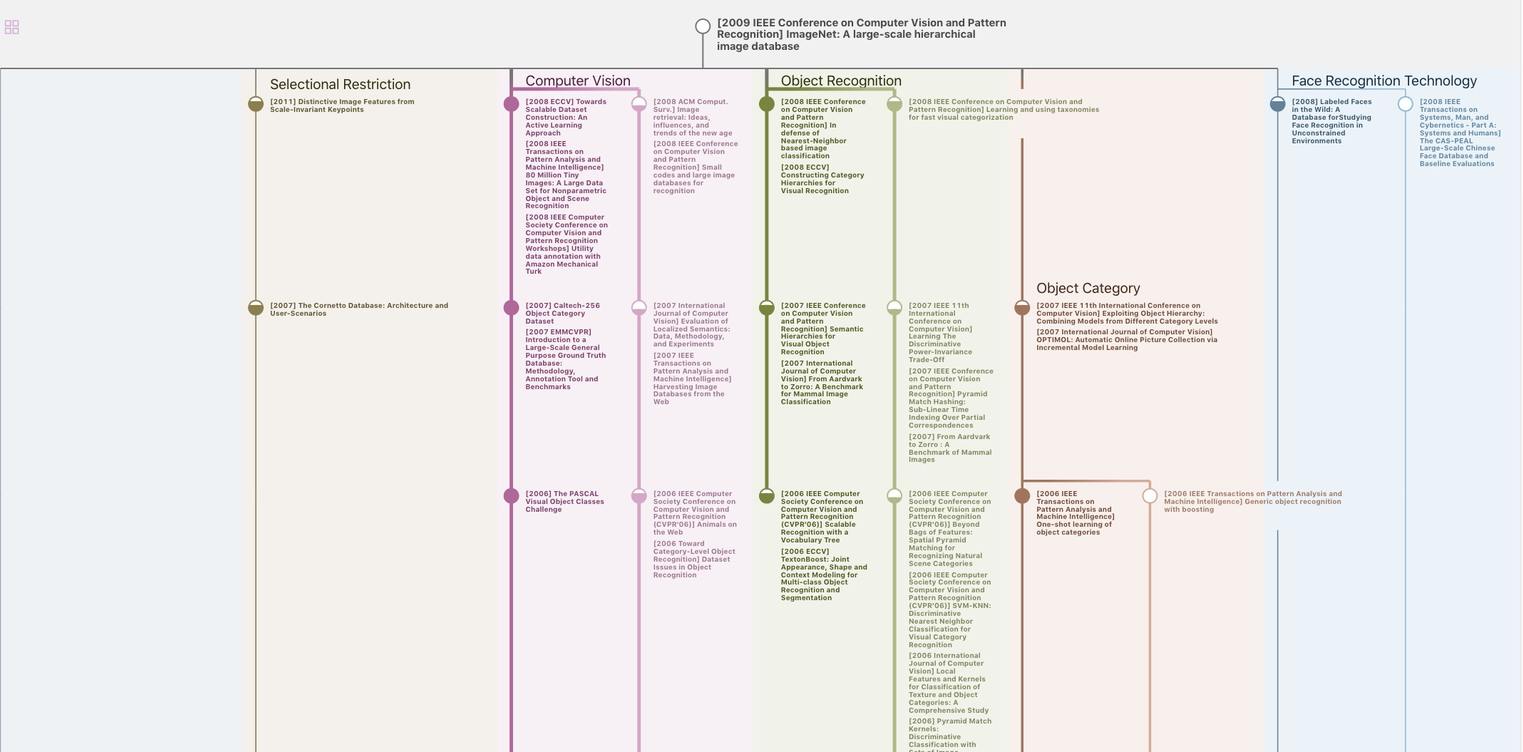
生成溯源树,研究论文发展脉络
Chat Paper
正在生成论文摘要