Deep Learning-Based Diagnosis Of Peripheral Artery Disease Via Continuous Property-Adversarial Regularization: Preliminary In Silico Study
IEEE ACCESS(2021)
Abstract
This paper presents a novel deep learning-based arterial pulse wave analysis (PWA) approach to diagnosis of peripheral artery occlusive disease (PAD). Naive application of deep learning to PAD diagnosis can be hampered by the fact that securing a large amount of longitudinal dataset encompassing diverse PAD severity as well as anatomical and physiological variability presents formidable challenge. Training of a deep neural network (DNN) to a small training dataset raises the risk of overfitting the PAD diagnosis algorithm only to the individuals in the training dataset while deteriorating its ability to generalize also to other individuals who may exhibit a large variability in anatomical and physiological characteristics beyond the training dataset. To overcome these obstacles, we propose a continuous property-adversarial regularization (CPAR) approach to robust generalization of a DNN against scarce datasets. Our approach fosters the exploitation of latent features that can facilitate the intended task independently of confounding property-induced disturbances. by regularizing the extraction of disturbance-dependent latent features in the network's feature extraction layer. By training and testing a deep convolutional neural network (CNN) for PAD diagnosis using scarce virtual datasets, we illustrated that the CNN trained by our approach was superior to a conventionally trained CNN in detecting and assessing the severity of PAD against disturbances originating from diversity in the patients' height and arterial stiffness: when trained with one-time pulse wave signal measurement at ankle and brachial arteries in a small number of patients, our approach achieved detection accuracy of >90% and severity assessment of 0.83 in r(2) value, which were >15% and >40% improvement over conventional approach without CPAR. In addition, we ascertained the advantage of our approach in efficient training and robust generalization of DNN by contrasting it to multi-task learning which promotes the exploitation (as opposed to regularization in CPAR) of disturbance-dependent latent features in fulfilling the intended tasks.
MoreTranslated text
Key words
Training, Feature extraction, Convolutional neural networks, Physiology, Task analysis, Diseases, Arteries, Deep learning, continuous domain-adversarial learning, peripheral artery disease, cardiovascular disease, arterial pulse wave analysis, convolutional neural network
AI Read Science
Must-Reading Tree
Example
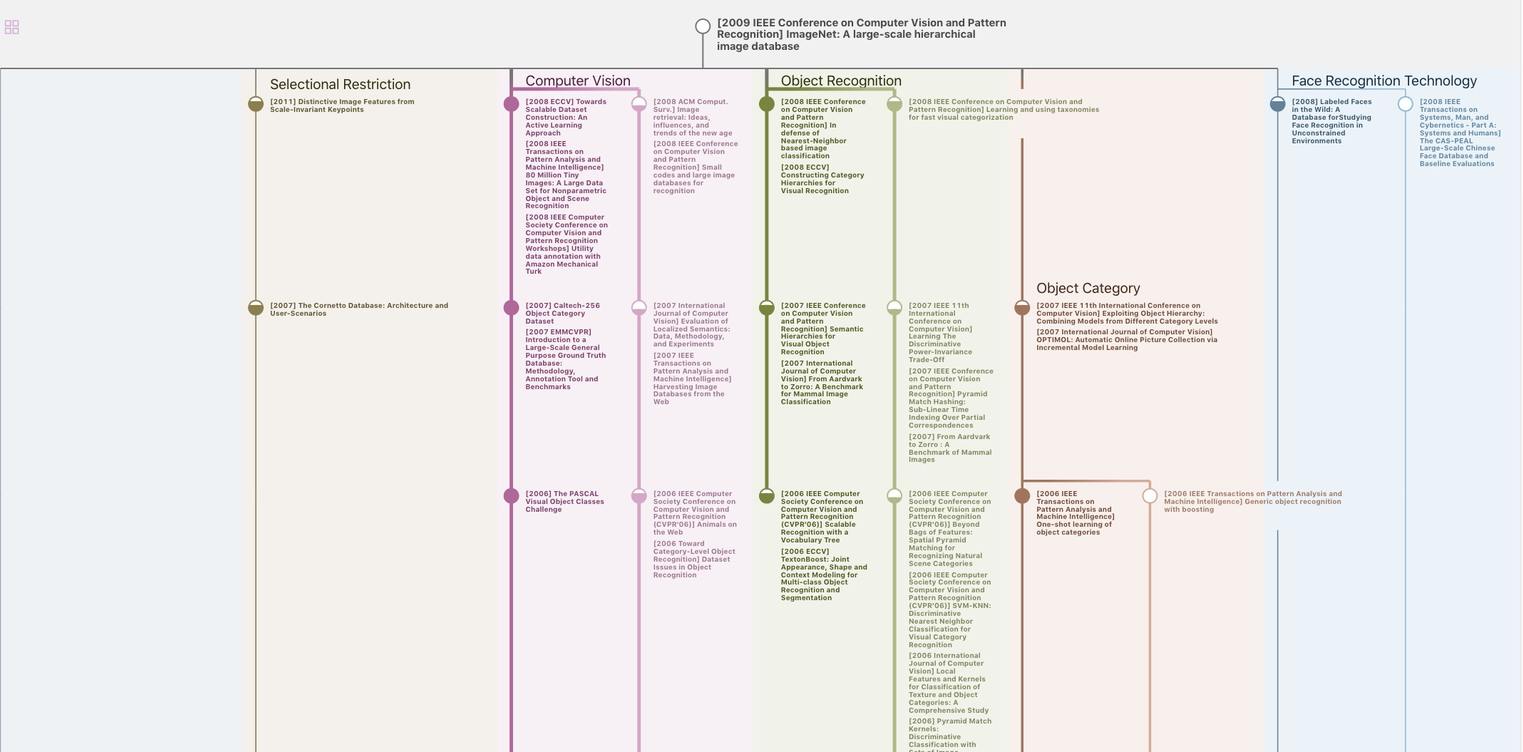
Generate MRT to find the research sequence of this paper
Chat Paper
Summary is being generated by the instructions you defined