Tire Surface Segmentation in Infrared Imaging with Convolutional Neural Networks and Transfer Learning
PATTERN RECOGNITION AND IMAGE ANALYSIS(2021)
摘要
It is crucial to analyze the tire under dynamic conditions to observe a performance close to realistic situations. Particularly, the temperature generated by the interaction of tire and pavement can provide useful information and understanding into how tire components can be optimized. However, in such a situation, data generation and measurement are challenging tasks. High-resolution thermal infrared imaging is a non-contact technology that transforms radiation patterns into a visible image and allows measuring the temperature changes on the surface of the tire. Therefore, the first step towards a systematic analysis of the performance of the tire is to segment the surface. To this end, we present an approach that combines traditional image processing methods with convolutional neural networks. We further investigate transfer learning techniques to improve the prediction of the proposed model on a different dataset. Our ultimate goal is to implement a robust network to segment a broad variety of tires. A segmentation accuracy >0.97 and a validation error <0.06 were achieved with transfer learning. The results have shown that our network can be extended to segment new data accurately.
更多查看译文
关键词
binary segmentation, convolutional neural networks, inception, tire segmentation, thermal infrared imaging, transfer learning, U-Net
AI 理解论文
溯源树
样例
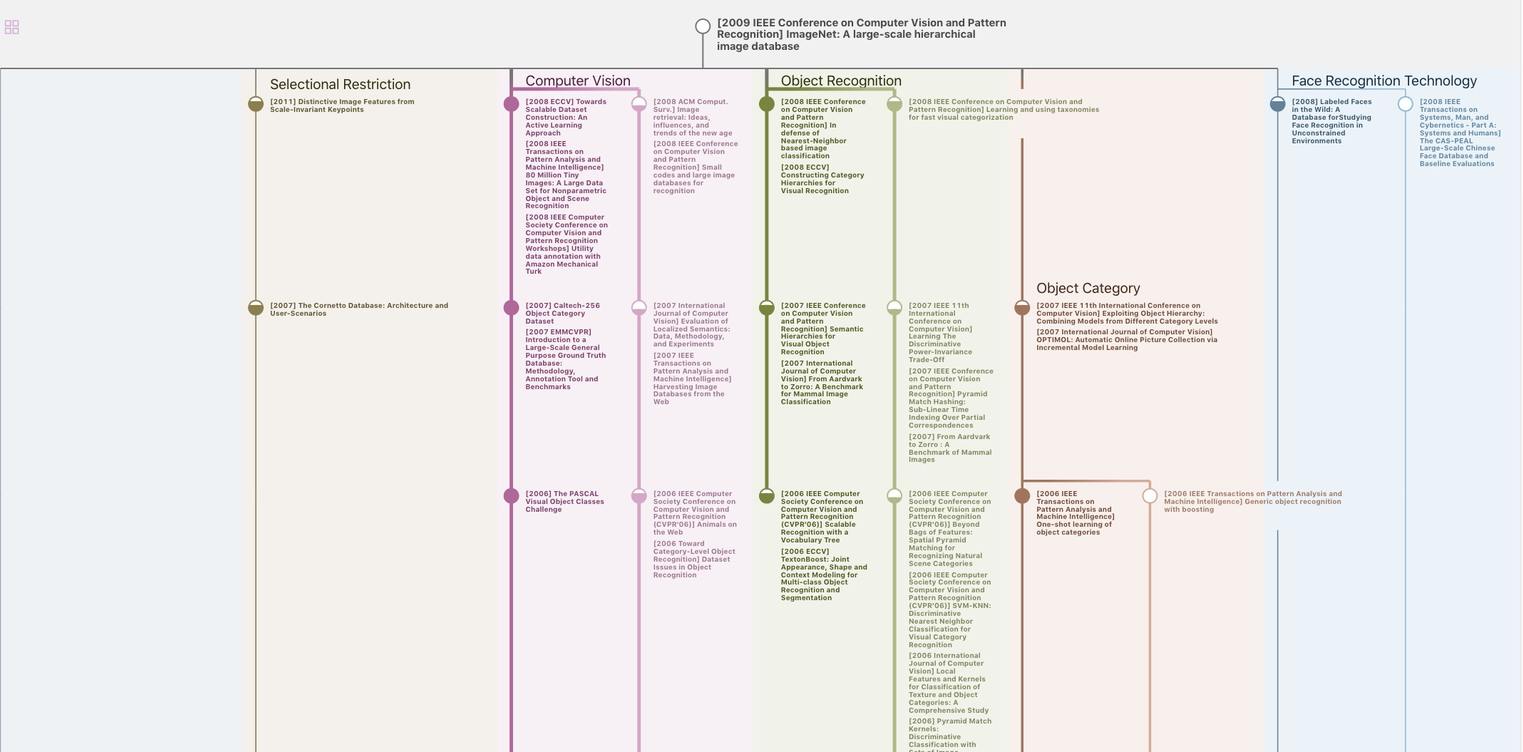
生成溯源树,研究论文发展脉络
Chat Paper
正在生成论文摘要