Variable scale learning for visual object tracking
JOURNAL OF AMBIENT INTELLIGENCE AND HUMANIZED COMPUTING(2021)
摘要
Recently, deep learning achieves competitive accuracy and robustness and dramatically improves the performance of target scale estimation through pre-trained special network branches. Yet, a fast and robust scale estimation method is still a challenging problem for visual object tracking. Early correlation filter tracking algorithm uses a multiscale search method to estimate the scale with the constant number of scale factors and invariant aspect ratio, which is redundant for the video frames with little or no scale change. Also, an independent network branch for target scale state is proposed, but the training network needs an abundance of datasets, and the effect is not very stable for the unseen target object. Aiming at the problems of existing scale estimation solutions, several variable scale learning methods are proposed to explore the scale change of the target. Firstly, we proposed a variable scale factor learning method, which makes us rid of the commonly used multiscale search with the flaws of fixed scale factors. Secondly, we used a multiscale aspect ratio solution to make up for invariant aspect ratio. Thirdly, the first and second scale methods were combined to propose a variable scale aspect ratio estimation method. Finally, the proposed scale estimation methods were embedded into the state-of-the-art ECO (Efficient Convolution Operators) and ATOM (Accurate Tracking by Overlap Maximization) trackers to replace the original scale methods for verifying the effectiveness of our proposed method. Extensive experiments on OTB100, UAV123, TC128 and LaSOT datasets demonstrate that the tracking performance can be improved effectively by using the proposed scale methods.
更多查看译文
关键词
Object tracking,Deep learning,Correlation filter,Scale estimation,Variable scale learning
AI 理解论文
溯源树
样例
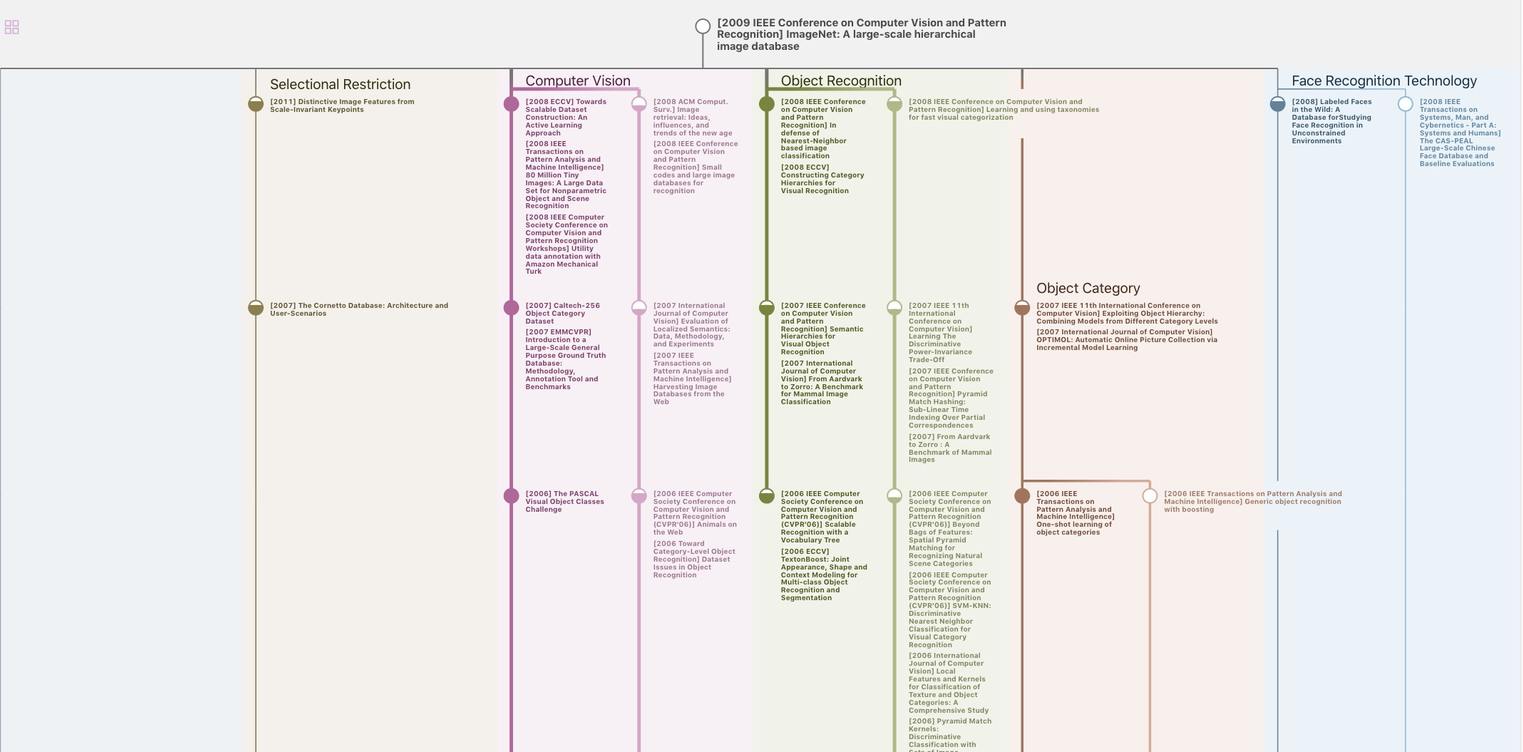
生成溯源树,研究论文发展脉络
Chat Paper
正在生成论文摘要