Sequential patent trading recommendation using knowledge-aware attentional bidirectional long short-term memory network (KBiLSTM)
JOURNAL OF INFORMATION SCIENCE(2023)
摘要
With the rapid development of the patent marketplace, patent trading recommendation is required to mitigate the technology searching cost of patent buyers. Current research focuses on the recommendation based on existing patents of a company; a few studies take into account the sequential pattern of patent acquisition activities and the possible diversity of a company's business interests. Moreover, the profiling of patents based on solely patent documents fails to capture the high-order information of patents. To bridge the gap, we propose a knowledge-aware attentional bidirectional long short-term memory network (KBiLSTM) method for patent trading recommendation. KBiLSTM uses knowledge graph embeddings to profile patents with rich patent information. It introduces bidirectional long short-term memory network (BiLSTM) to capture the sequential pattern in a company's historical records. In addition, to address a company's diverse technology interests, we design an attention mechanism to aggregate the company's historical patents given a candidate patent. Experimental results on the United States Patent and Trademark Office (USPTO) data set show that KBiLSTM outperforms state-of-the-art baselines for patent trading recommendation in terms of F1 and normalised discounted cumulative gain (nDCG). The attention visualisation of randomly selected company intuitively demonstrates the recommendation effectiveness.
更多查看译文
关键词
Deep learning, knowledge graph, patent trading recommendation, sequential recommendation
AI 理解论文
溯源树
样例
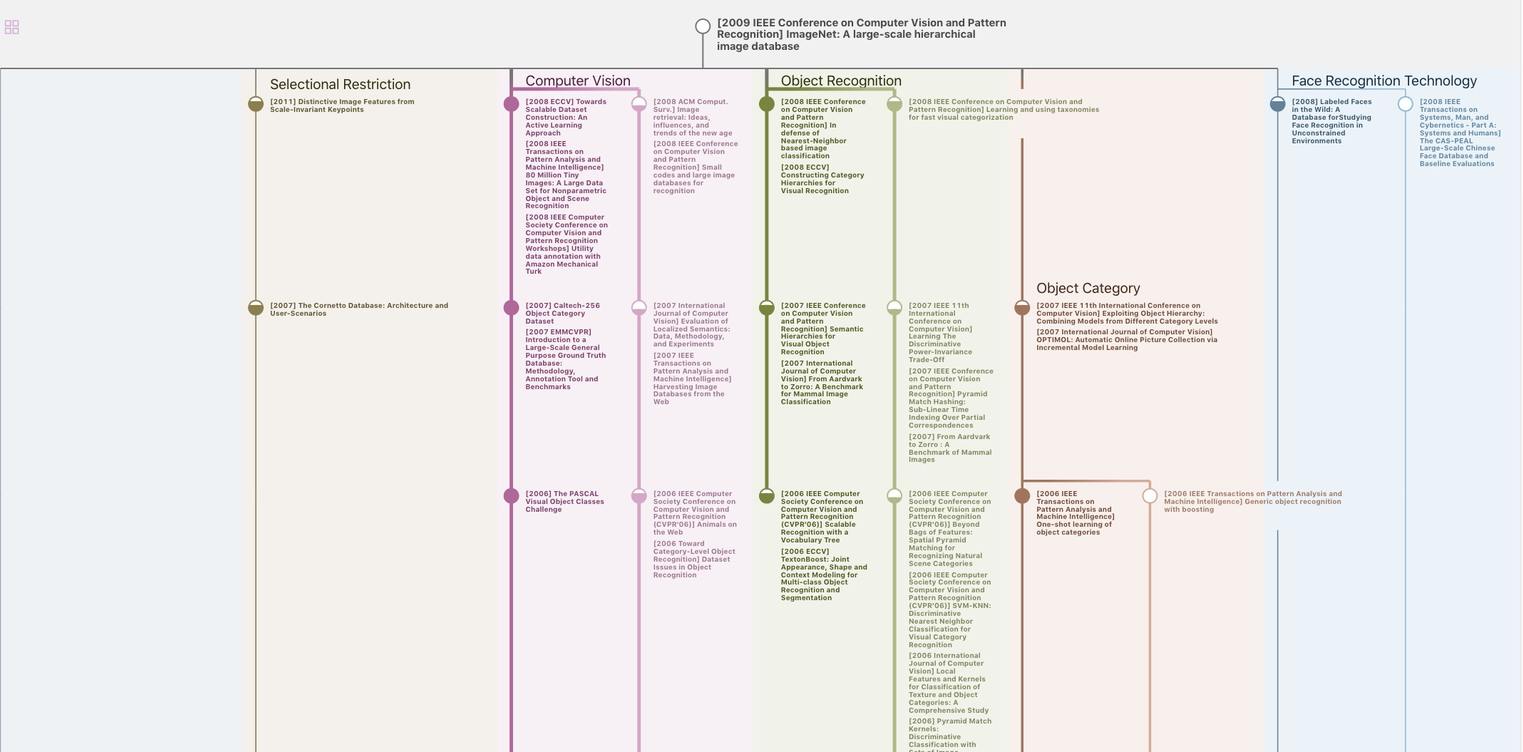
生成溯源树,研究论文发展脉络
Chat Paper
正在生成论文摘要