Hybrid Representation Of Sensor Data For The Classification Of Driving Behaviour
APPLIED SCIENCES-BASEL(2021)
摘要
Monitoring driving behaviour is important in controlling driving risk, fuel consumption, and CO2 emissions. Recent advances in machine learning, which include several variants of convolutional neural networks (CNNs), and recurrent neural networks (RNNs), such as long short-term memory (LSTM) and gated recurrent unit (GRU) networks, could be valuable for the development of objective and efficient computational tools in this direction. The main idea in this work is to complement data-driven classification of driving behaviour with rules derived from domain knowledge. In this light, we present a hybrid representation approach, which employs NN-based time-series encoding and rule-guided event detection. Histograms derived from the output of these two components are concatenated, normalized, and used to train a standard support vector machine (SVM). For the NN-based component, CNN-based, LSTM-based, and GRU-based variants are investigated. The CNN-based variant uses image-like representations of sensor measurements, whereas the RNN-based variants (LSTM and GRU) directly process sensor measurements in the form of time-series. Experimental evaluation on three datasets leads to the conclusion that the proposed approach outperforms a state-of-the-art camera-based approaches in distinguishing between normal and aggressive driving behaviour without using data derived from a camera. Moreover, it is demonstrated that both NN-guided time-series encoding and rule-guided event detection contribute to overall classification accuracy.
更多查看译文
关键词
driving behaviour, convolutional neural networks, recurrent neural networks, telematics sensors
AI 理解论文
溯源树
样例
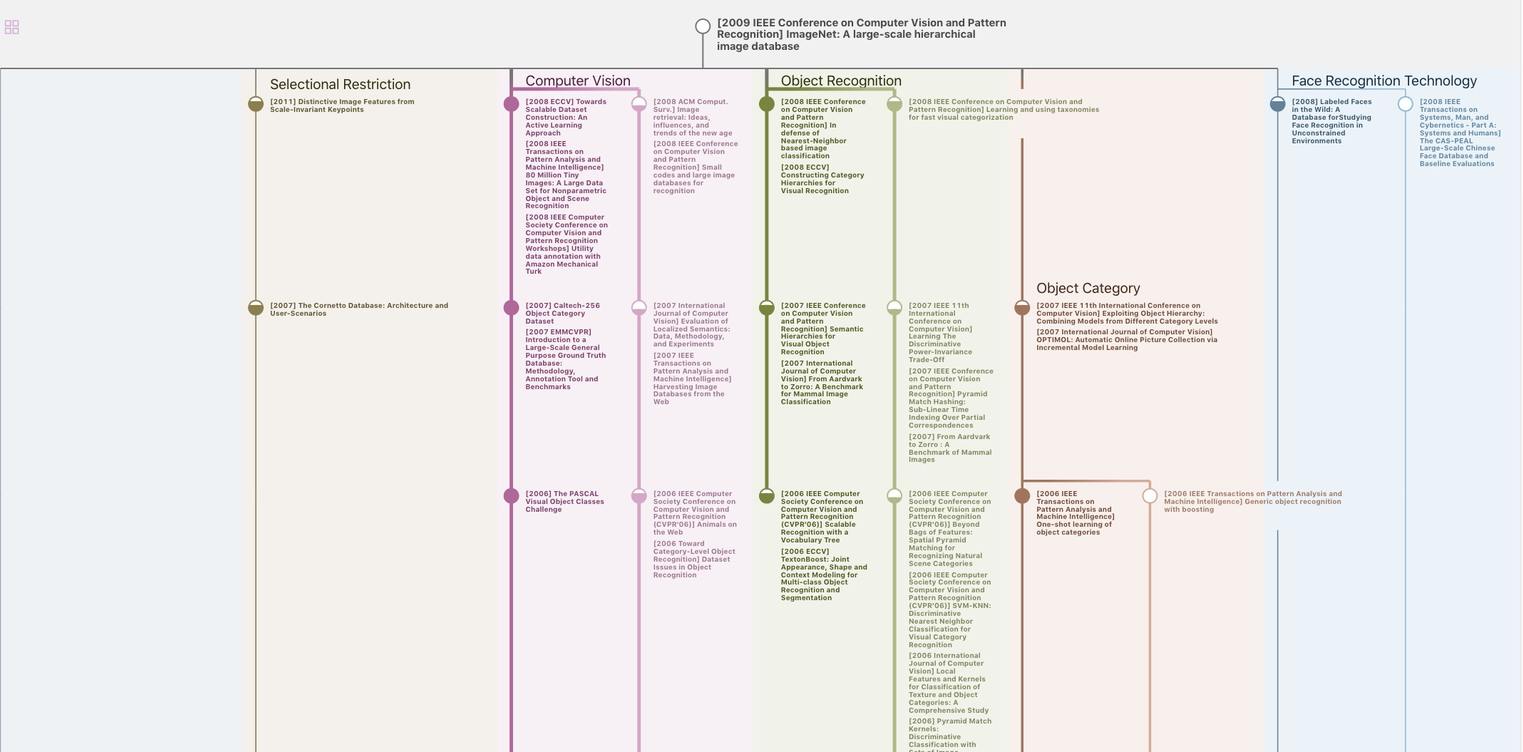
生成溯源树,研究论文发展脉络
Chat Paper
正在生成论文摘要