Optimizing Input Mask For Maximum Memory Performance Of Time-Delay Reservoir Subjected To State Noise
IEICE NONLINEAR THEORY AND ITS APPLICATIONS(2021)
摘要
Reservoir computing is a brain-inspired machine-learning framework that has been successfully used in information processing. A state-of-the-art methodology, called time-delay reservoir (TDR), realizes the reservoir using a single nonlinear physical node with delayed self-feedback. Memory capacity of the TDR is sensitive to the time-multiplexing procedure for input signals with a random mask. The existing memory optimization methods with respect to the masks are limited to the case without state noise, and their computational cost is large. In this article, we optimize the input mask to maximize the memory performance of the TDR with a white Gaussian state noise in a computational time efficient manner within the context of the Fisher memory curve, and then a TDR based on the Mackey-Glass system is used to illustrate our proposed method. The memory-nonlinearity trade-off of the TDRs regarding the input masks is also investigated in view of spectral properties of the spatial Fisher information matrix.
更多查看译文
关键词
reservoir computing, short term memory capacity, time-delay reservoir, fisher memory curve, input mask, optimization
AI 理解论文
溯源树
样例
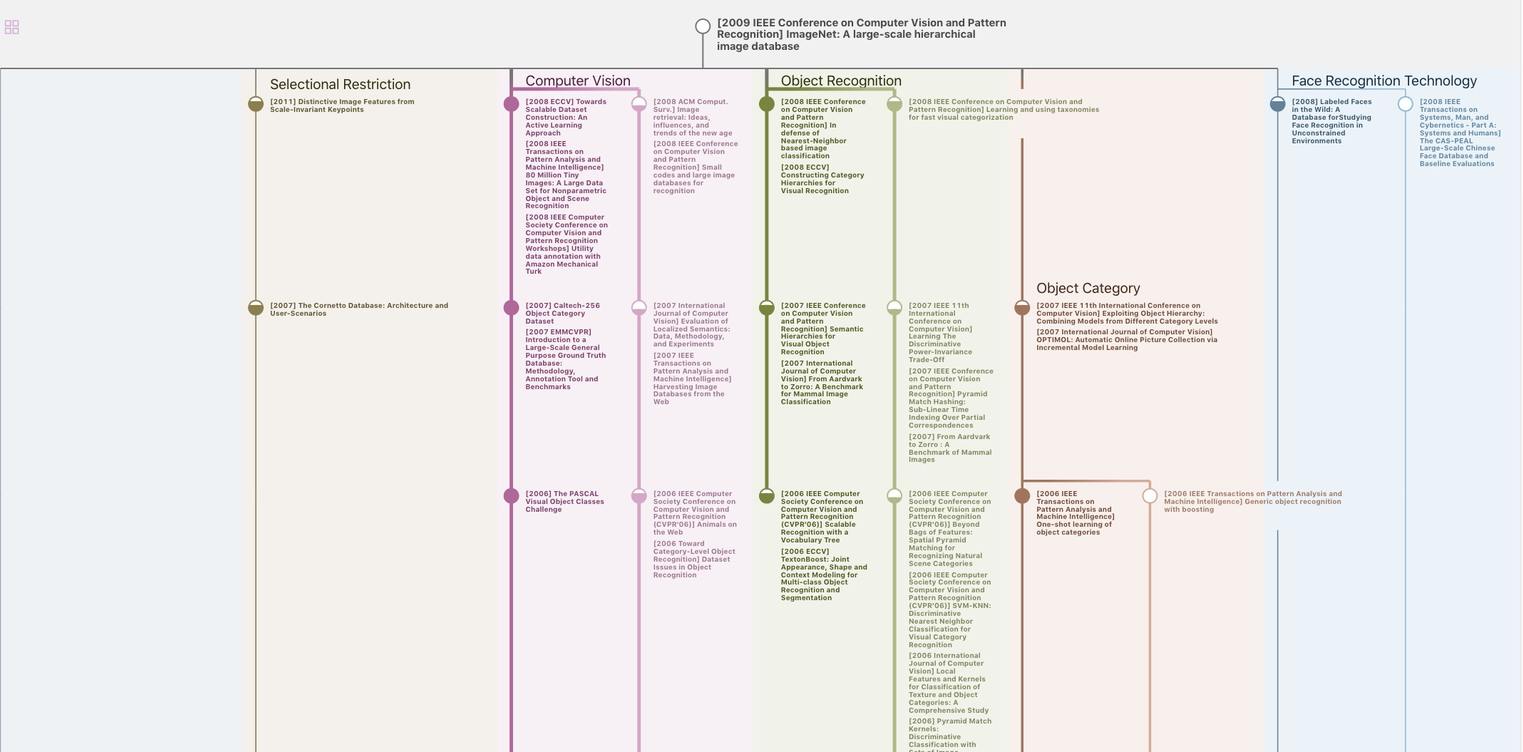
生成溯源树,研究论文发展脉络
Chat Paper
正在生成论文摘要