Increasing the Energy-Efficiency in Vacuum-Based Package Handling Using Deep Q-Learning
ENERGIES(2021)
摘要
Billions of packages are automatically handled in warehouses every year. The gripping systems are, however, most often oversized in order to cover a large range of different carton types, package masses, and robot motions. In addition, a targeted optimization of the process parameters with the aim of reducing the oversizing requires prior knowledge, personnel resources, and experience. This paper investigates whether the energy-efficiency in vacuum-based package handling can be increased without the need for prior knowledge of optimal process parameters. The core method comprises the variation of the input pressure for the vacuum ejector, compliant to the robot trajectory and the resulting inertial forces at the gripper-object-interface. The control mechanism is trained by applying reinforcement learning with a deep Q-agent. In the proposed use case, the energy-efficiency can be increased by up to 70% within a few hours of learning. It is also demonstrated that the generalization capability with regard to multiple different robot trajectories is achievable. In the future, the industrial applicability can be enhanced by deployment of the deep Q-agent in a decentral system, to collect data from different pick and place processes and enable a generalizable and scalable solution for energy-efficient vacuum-based handling in warehouse automation.
更多查看译文
关键词
vacuum-based handling,energy-efficiency,deep Q-learning,automation
AI 理解论文
溯源树
样例
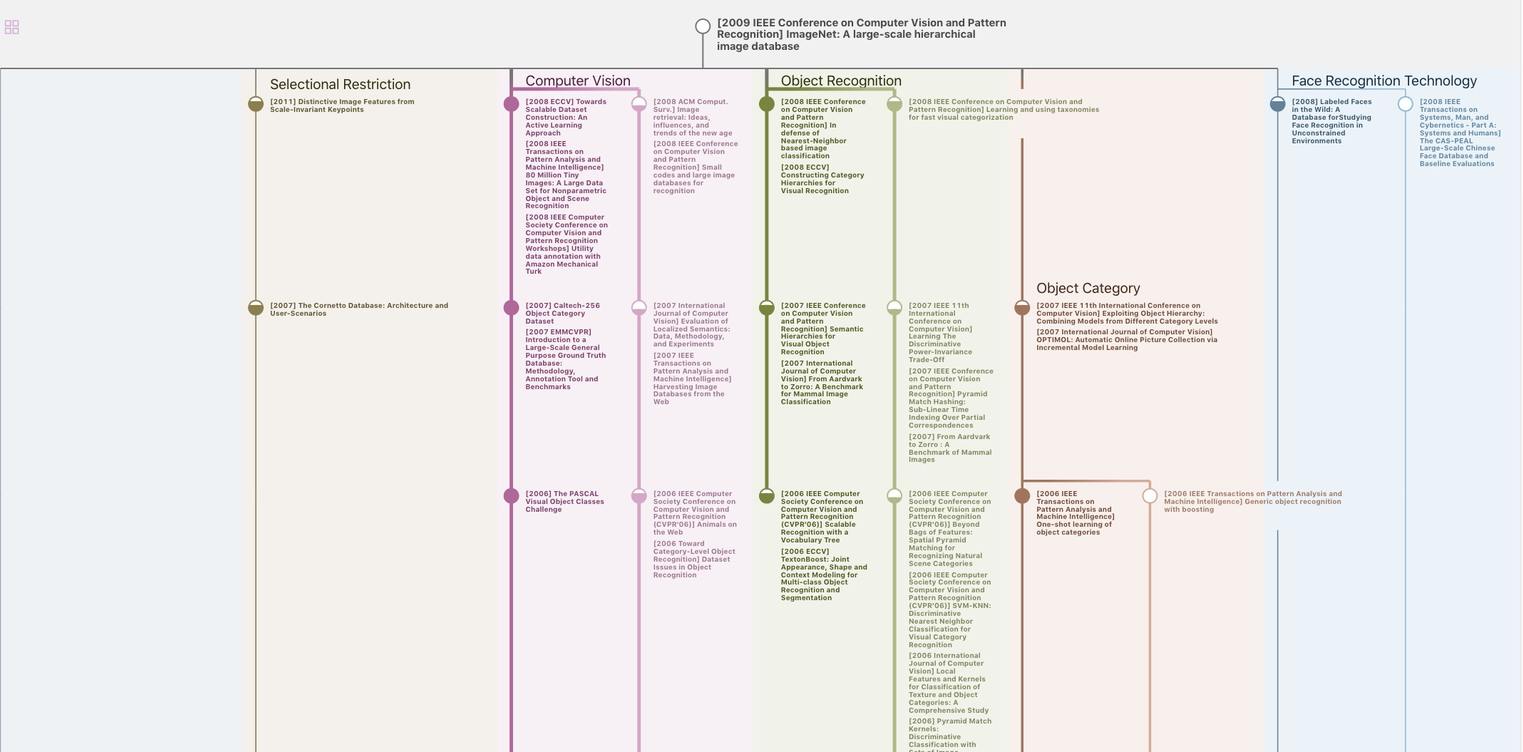
生成溯源树,研究论文发展脉络
Chat Paper
正在生成论文摘要