A Deep Learning Approach For Rock Fragmentation Analysis
INTERNATIONAL JOURNAL OF ROCK MECHANICS AND MINING SCIENCES(2021)
摘要
In mining operations, blast-induced rock fragmentation affects the productivity and efficiency of downstream operations including digging, hauling, crushing, and grinding. Continuous measurement of rock fragmentation is essential for optimizing blast design. Current methods of rock fragmentation analysis rely on either physical screening of blasted rock material or image analysis of the blasted muckpiles; both are time consuming. This study aims to present and evaluate the measurement of rock fragmentation using deep learning strategies. A deep neural network (DNN) architecture was used to predict characteristic sizes of rock fragments from a 2D image of a muckpile. The data set used for training the DNN model is composed of 61,853 labelled images of blasted rock fragments. An exclusive data set of 1,263 labelled images were used to test the DNN model. The percent error for coarse characteristic size prediction ranges within +/- 25% when evaluated using the test set. Model validation on orthomosaics for two muckpiles shows that the deep learning method achieves a good accuracy (lower mean percent error) compared to manual image labelling. Validation on screened piles shows that the DNN model prediction is similar to manual labelling accuracy when compared with sieving analysis.
更多查看译文
关键词
Blasting, Rock fragmentation, Deep learning, DNN
AI 理解论文
溯源树
样例
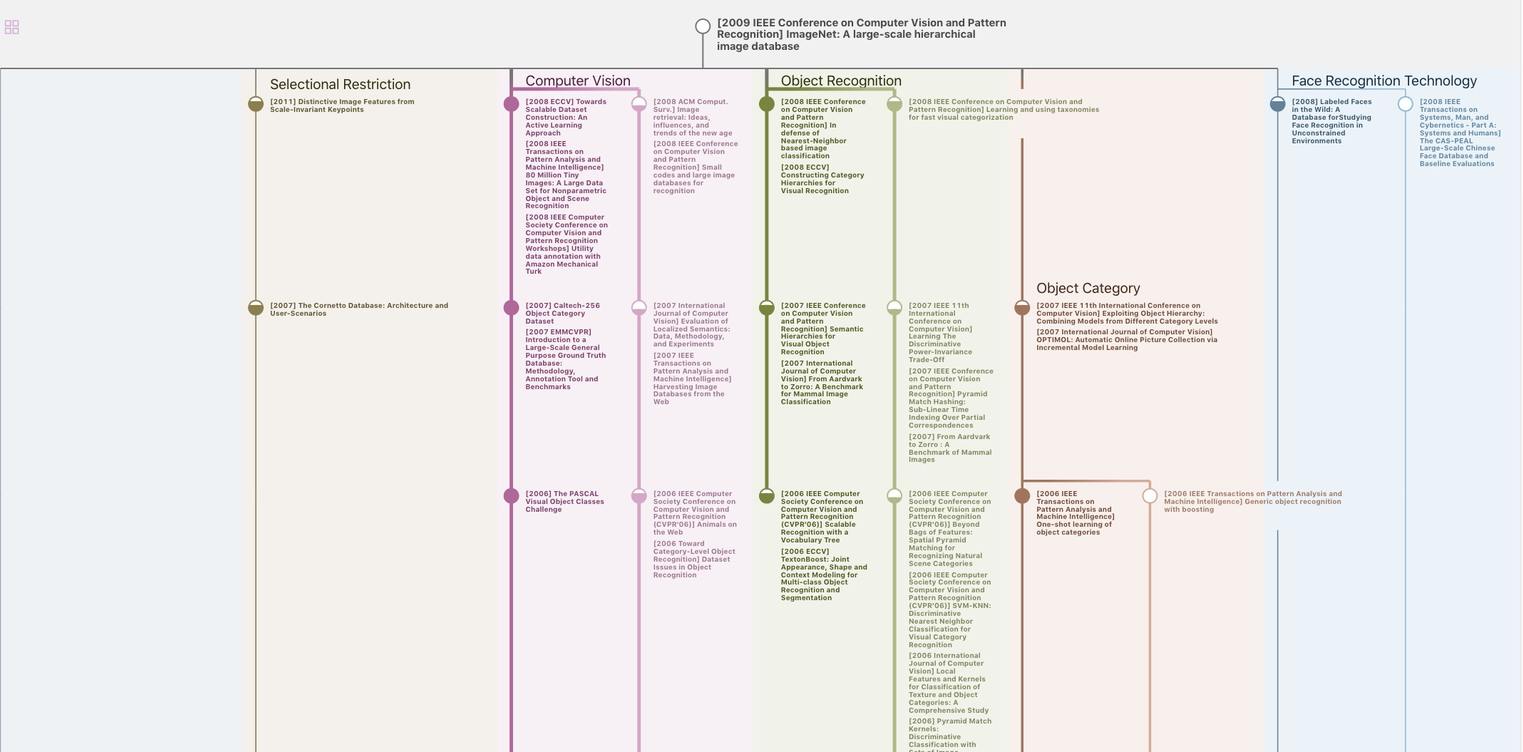
生成溯源树,研究论文发展脉络
Chat Paper
正在生成论文摘要