Crop yield forecasting and associated optimum lead time analysis based on multi-source environmental data across China
AGRICULTURAL AND FOREST METEOROLOGY(2021)
Abstract
Accurate and timely crop yield forecasts can provide essential information to make conclusive agricultural policies and to conduct investments. Recent studies have used different machine learning techniques to develop such yield forecast systems for single crops at regional scales. However, no study has used multiple sources of environmental predictors (climate, soil, and vegetation) to forecast yields for three major crops in China. In this study, we adopted 7-year observed crop yield data (2013-2019) for three major grain crops (wheat, maize, and rice) across China, and three major data sets including climate, vegetation indices, and soil properties were used to develop a dynamic yield forecasting system based on the random forest (RF) model. The RF model showed good performance for estimating yields of all three crops with correlation coefficient (r) higher than 0.75 and normalized root means square errors (nRMSE) lower than 18.0%. Our results also showed that crop yields can be satisfactorily forecasted at one to three months prior to harvest. The optimum lead time for yield forecasting depended on crop types. In addition, we found the major predictors influencing crop yield varied between crops. In general, solar radiation and vegetation indices (especially during jointing to milk development stages) were identified as the main predictor for winter wheat; vegetation indices (throughout the growing season) and drought (especially during emergence to tasseling stages) were the most important predictors for spring maize; soil moisture (throughout the growing season) was the dominant predictor for summer maize, late rice, and mid rice; precipitation (especially during booting to heading stages) was the main predictor for early rice. Our study provides insights into practical crop yield forecasting and the understanding of yield response to environmental conditions at a large scale across China. The methods undertaken in this research can be easily implemented in other countries with available information on climate, soil, and vegetation conditions.
MoreTranslated text
Key words
Crop yield forecast,Climate extremes,Remote sensing,Random forest,Lead time,Variable importance
AI Read Science
Must-Reading Tree
Example
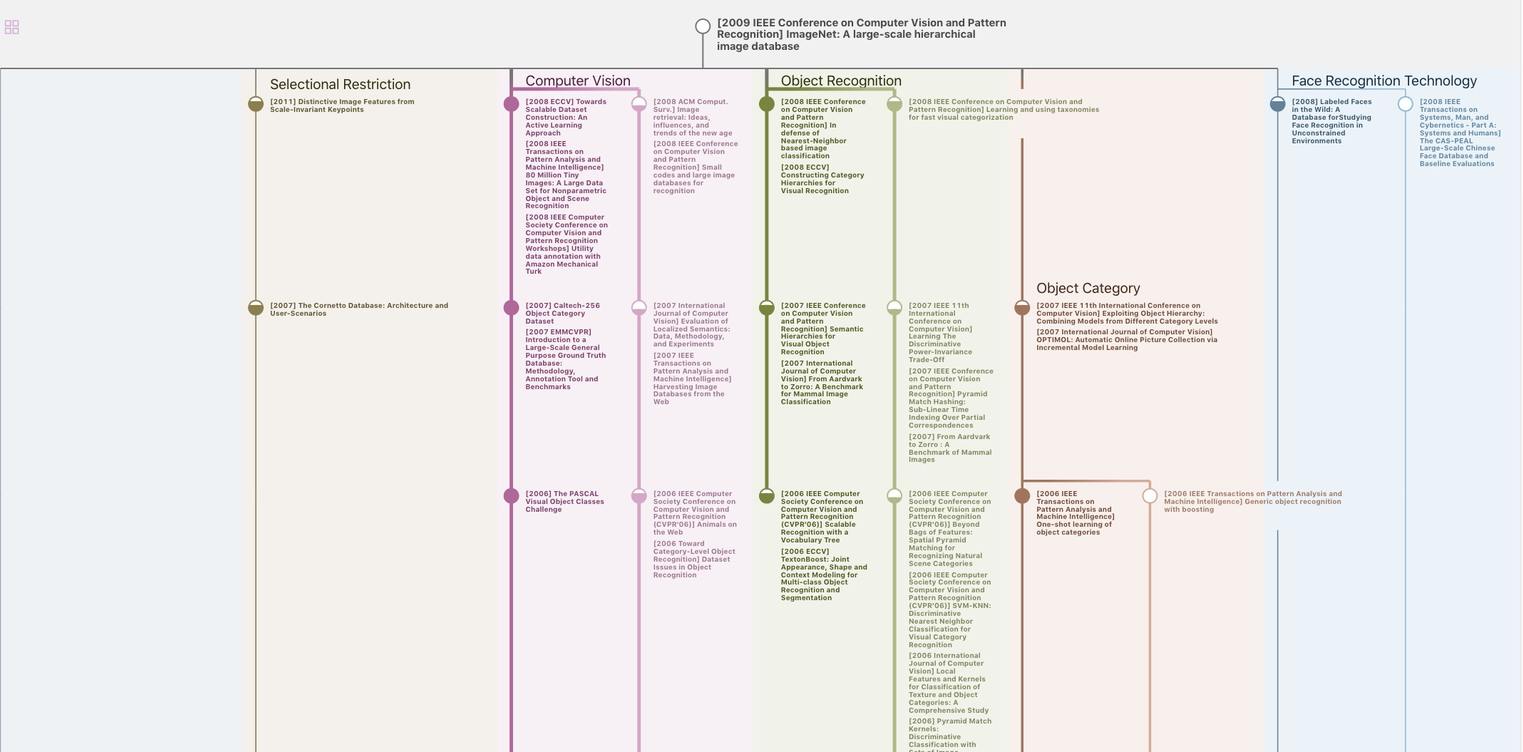
Generate MRT to find the research sequence of this paper
Chat Paper
Summary is being generated by the instructions you defined