A Novel Machine Learning Model To Predict The Photo-Degradation Performance Of Different Photocatalysts On A Variety Of Water Contaminants
CATALYSTS(2021)
摘要
This paper describes an innovative machine learning (ML) model to predict the performance of different metal oxide photocatalysts on a wide range of contaminants. The molecular structures of metal oxide photocatalysts are encoded with a crystal graph convolution neural network (CGCNN). The structure of organic compounds is encoded via digital molecular fingerprints (MF). The encoded features of the photocatalysts and contaminants are input to an artificial neural network (ANN), named as CGCNN-MF-ANN model. The CGCNN-MF-ANN model has achieved a very good prediction of the photocatalytic degradation rate constants by different photocatalysts over a wide range of organic contaminants. The effects of the data training strategy on the ML model performance are compared. The effects of different factors on photocatalytic degradation performance are further evaluated by feature importance analyses. Examples are illustrated on the use of this novel ML model for optimal photocatalyst selection and for assessing other types of photocatalysts for different environmental applications.
更多查看译文
关键词
photocatalytic degradation, machine learning, crystal graphic convolutional neural network, molecular fingerprint, artificial neural network
AI 理解论文
溯源树
样例
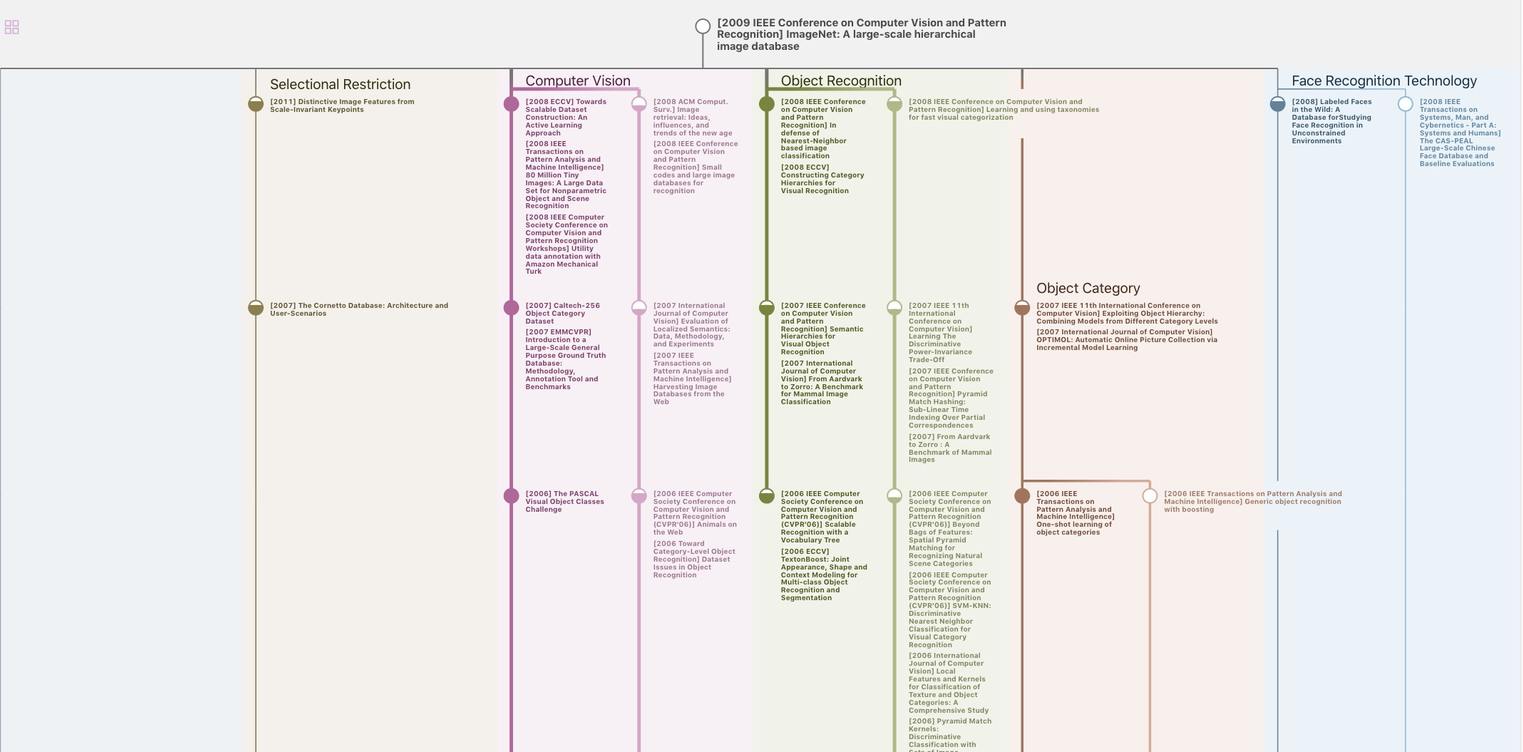
生成溯源树,研究论文发展脉络
Chat Paper
正在生成论文摘要