Multi-Objective Optimization Of Mig Welding And Preheat Parameters For 6061-T6 Al Alloy T-Joints Using Artificial Neural Networks Based On Fem
COATINGS(2021)
Abstract
To control the welding residual stress and deformation of metal inert gas (MIG) welding, the influence of welding process parameters and preheat parameters (welding speed, heat input, preheat temperature, and preheat area) is discussed, and a prediction model is established to select the optimal combination of process parameters. Thermomechanical numerical analysis was performed to obtain the residual welding deformation and stress according to a 100 x 150 x 50 x 4 mm aluminum alloy 6061-T6 T-joint. Owing to the complexity of the welding process, an optimal Latin hypercube sampling (OLHS) method was adopted for sampling with uniformity and stratification. Analysis of variance (ANOVA) was used to find the influence degree of welding speed (7.5-9 mm/s), heat input (1500-1700 W), preheat temperature (80-125 degrees C), and preheat area (12-36 mm). The range of research parameters are according to the material, welding method, thickness of the welding plate, and welding procedure specification. Artificial neural network (ANN) and multi-objective particle swarm optimization (MOPSO) was combined to find the effective parameters to minimize welding deformation and stress. The results showed that preheat temperature and welding speed had the greatest effect on the minimization of welding residual deformation and stress, followed by the preheat area, respectively. The Pareto front was obtained by using the MOPSO algorithm with epsilon-dominance. The welding residual deformation and stress are the minimum at the same time, when the welding parameters are selected as preheating temperature 85 degrees C and preheating area 12 mm, welding speed is 8.8 mm/s and heat input is 1535 W, respectively. The optimization results were validated by the finite element (FE) method. The error between the FE results and the Pareto optimal compromise solutions is less than 12.5%. The optimum solutions in the Pareto front can be chosen by designers according to actual demand.
MoreTranslated text
Key words
welding parameters, preheat parameters, welding deformation, welding stress, multi-objective optimization, artificial neural network, FEM simulation
AI Read Science
Must-Reading Tree
Example
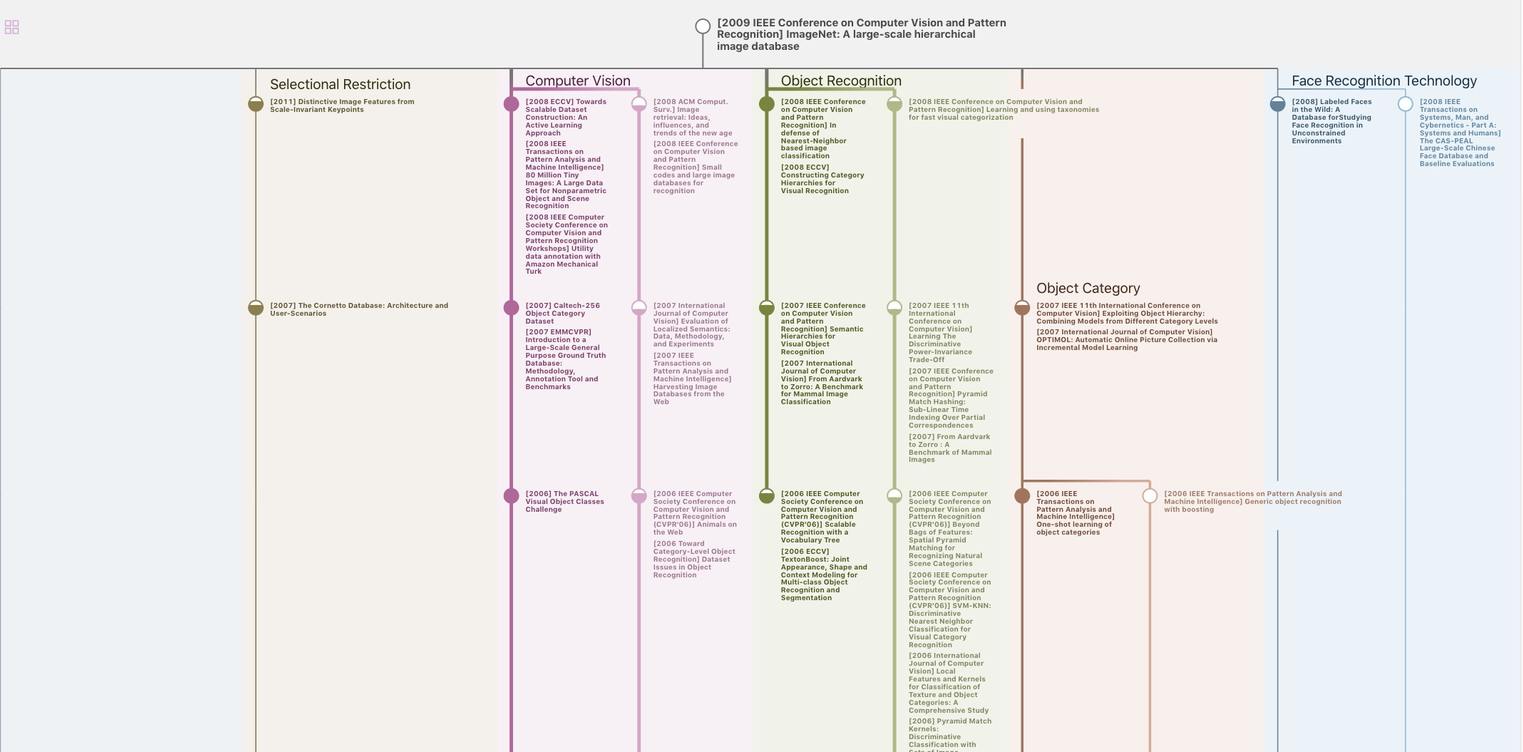
Generate MRT to find the research sequence of this paper
Chat Paper
Summary is being generated by the instructions you defined