Molecular Image-Convolutional Neural Network (Cnn) Assisted Qsar Models For Predicting Contaminant Reactivity Toward Oh Radicals: Transfer Learning, Data Augmentation And Model Interpretation
CHEMICAL ENGINEERING JOURNAL(2021)
摘要
In this study, we used molecular images as a representation for organic compounds and combined them with a convolutional neural network (CNN) to develop quantitative structure-activity relationships (QSARs) for predicting compound rate constants toward OH radicals. We applied transfer learning and data augmentation to train molecular image-CNN models and the Gradient-weighted Class Activation Mapping (Grad-CAM) method to interpret them. Results showed that data augmentation and transfer learning can effectively enhance the robustness and predictive performance of the models, with the root-mean-square-error (RMSE) values on the test dataset (RMSEtest) decreasing from (0.395-0.45) to (0.284-0.339) after applying data augmentation, and the RMSE on the training dataset (RMSEtrain) decreasing from (0.452-0.592) to (0.123-0.151) after applying transfer learning. The obtained molecular image-CNN models showed comparative predictive performance (RMSEtest 0.284-0.339) with the molecular fingerprint-based models (RMSEtest 0.30-0.35). Grad-CAM interpretation showed that the molecular image-CNN models correctly chose the molecular features in the images and identified key functional groups that influenced the reactivity. The applicability domain analysis showed that the molecular image-CNN models have a broader applicability domain than molecular fingerprints-based models and the reactivity of any new compounds with a maximum similarity of over 0.85 to the compounds in the training dataset can be reliably predicted. This study demonstrated that molecular image-CNN is a new tool to develop QSARs for environmental applications and can be used to build trustful models that make meaningful predictions.
更多查看译文
关键词
Convolutional neural network (CNN), Hydroxyl radical, Model interpretation, Machine learning, Molecular images, QSARs
AI 理解论文
溯源树
样例
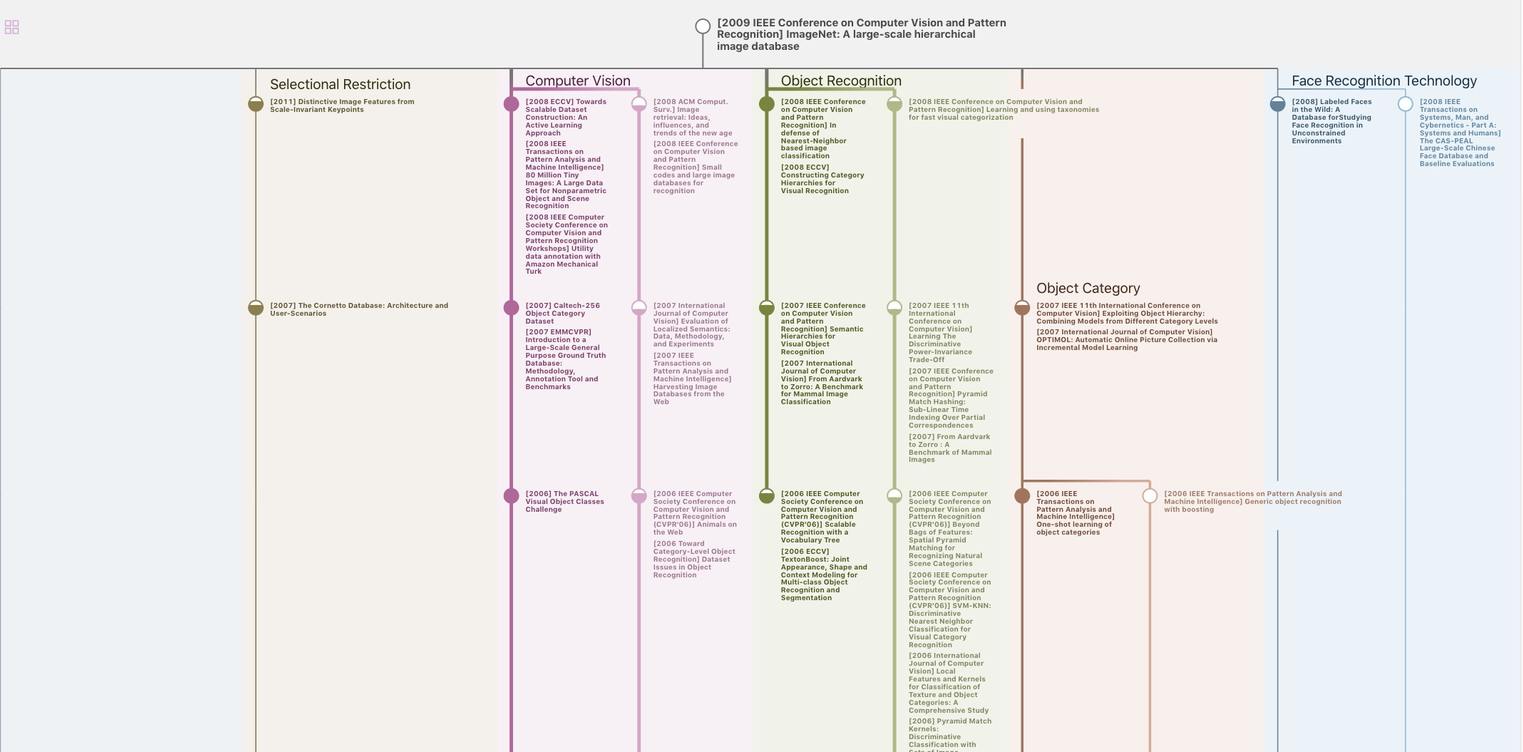
生成溯源树,研究论文发展脉络
Chat Paper
正在生成论文摘要