Wear Debris Segmentation Of Reflection Ferrograms Using Lightweight Residual U-Net
IEEE TRANSACTIONS ON INSTRUMENTATION AND MEASUREMENT(2021)
Abstract
Extraction of lube oil wear debris morphological features is an important means for real-time monitoring of equipment wear, and online visual ferrograph (OLVF) is one of the representative technologies. In the current OLVF wear monitoring, the transmission ferrogram (TF) is basically relied on, but the more informative reflection ferrogram (RF) has not yet been applied, because its complex surface color distribution and bubble interference make it difficult to segment the RE Accordingly, a convolutional neural network (CNN) model called lightweight residual U-net (Res-UNet) is constructed in this article. Simultaneously, with both RFs and TFs, an automatic labeling method is proposed to label the RFs and make a training dataset to implement network training. The experimental results demonstrate that the trained network can achieve accurate segmentation of RFs with excellent anti-interference performance. The proposed method lays the foundation for the feature extraction of reflection OLVF ferrograms and provides an alternative image segmentation method for other image wear debris sensors.
MoreTranslated text
Key words
Automatically labeled, convolutional neural network (CNN), deep learning, ferrography, wear debris segmentation
AI Read Science
Must-Reading Tree
Example
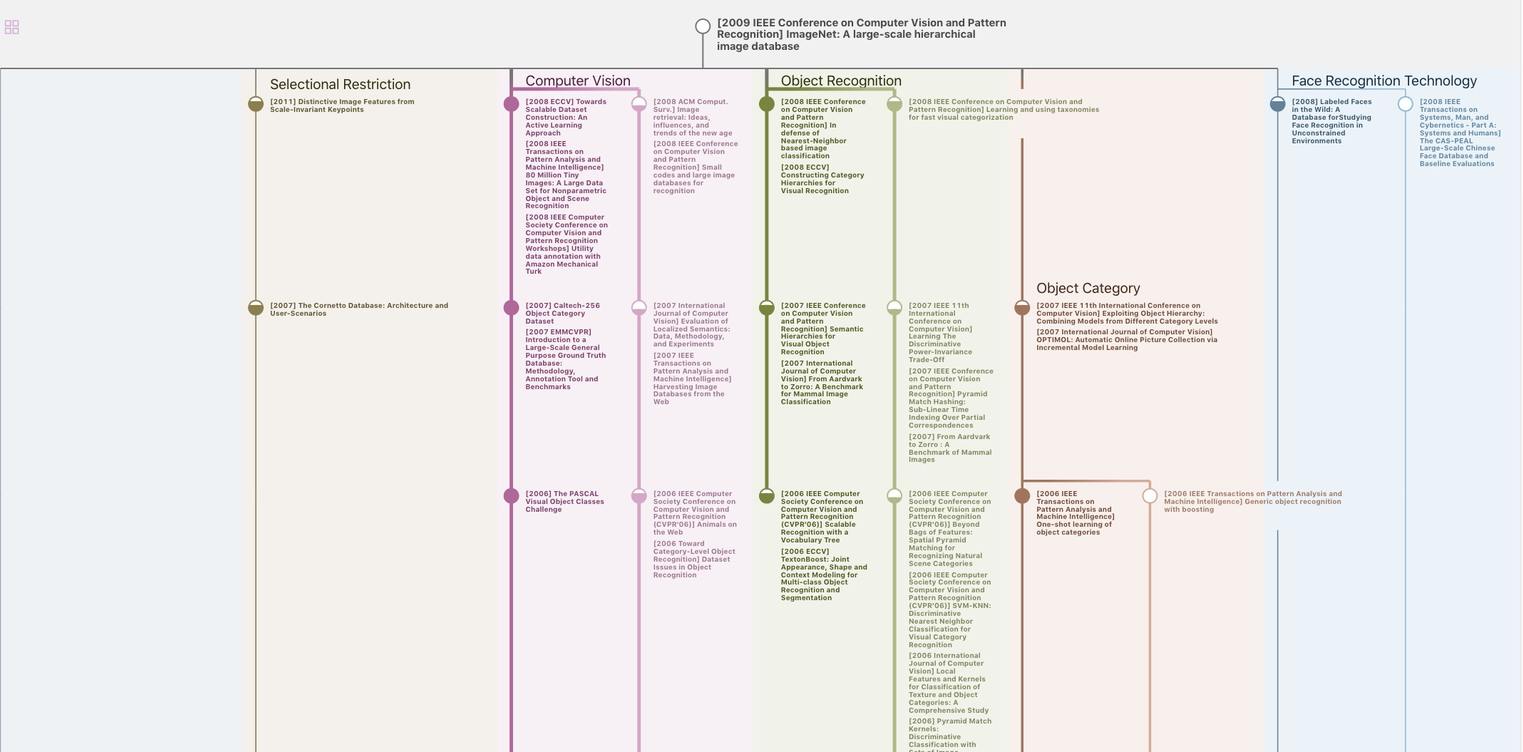
Generate MRT to find the research sequence of this paper
Chat Paper
Summary is being generated by the instructions you defined