A Supervised Framework For Recognition Of Liquid Rocket Engine Health State Under Steady-State Process Without Fault Samples
IEEE TRANSACTIONS ON INSTRUMENTATION AND MEASUREMENT(2021)
摘要
Data-driven intelligent models have received more and more attention in liquid rocket engine (LRE) state recognition. However, due to practical limitations, the fault data are rare for model training, which results in poor testing results. To better recognize the state of LRE, we proposed a supervised recognition framework without fault samples. First, we proposed a negative sample generation method. Then, fusion recurrent convolutional neural network (FRCNN) is constructed for data fusion and feature extraction. Finally, we evaluated the recognition results with several quantization indicators. The proposed method is verified with the real monitoring data, which is obtained from the static firing tests of a certain type of LRE. Results show that the FRCNN could recognize the state of LRE with accuracy over 93% under the confidence of 96%, which shows the effectiveness of the proposed framework. And the comparison with the literature method shows the superiority of the proposed framework. In addition, it could also perform real-time monitoring for LRE, and recognize the abnormal at the initial stage of the steady state, which has practical potential in monitoring task. At last, we further discuss and analyze the generated negative sample and the proposed framework.
更多查看译文
关键词
Deep learning, health state, liquid rocket engine (IRE), multi-source data, negative sample
AI 理解论文
溯源树
样例
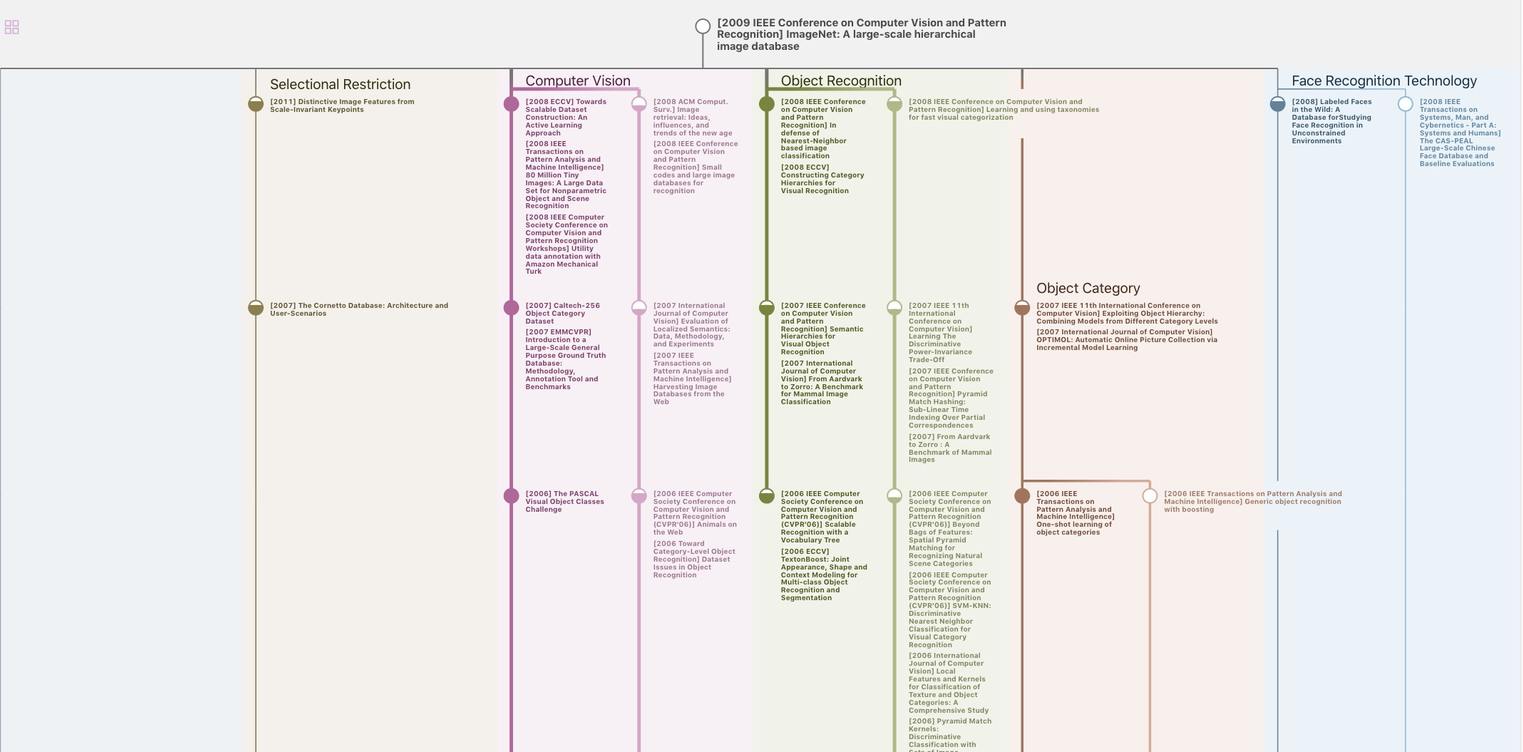
生成溯源树,研究论文发展脉络
Chat Paper
正在生成论文摘要